
Artificial Intelligence: The Game-Changer in Anti-Money Laundering
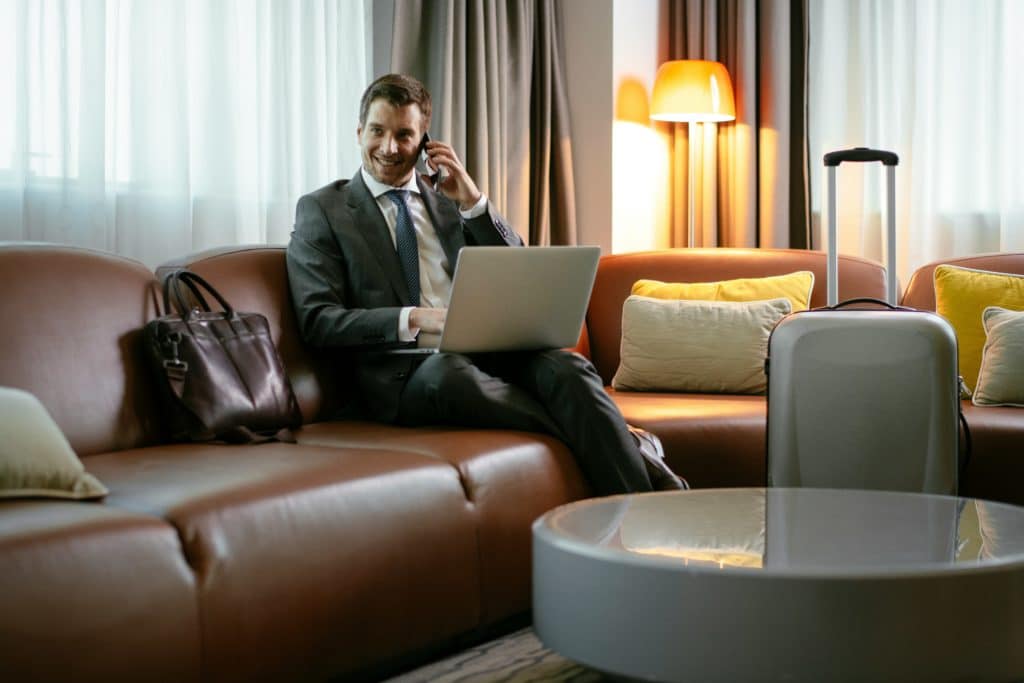
Introduction
The global economy faces a substantial threat from money laundering, the act of disguising the source of illegally acquired funds. This criminal activity costs billions of dollars each year. To counter this threat, governments and financial institutions have established Anti-Money Laundering (AML) regulations. In recent years, Artificial Intelligence (AI) has emerged as a powerful tool, significantly enhancing the effectiveness of AML efforts. This article will examine how AI is revolutionizing AML practices, exploring its benefits, challenges, and future potential.
AI’s Impact on AML
The fight against financial crime is undergoing a significant revolution with the integration of Artificial Intelligence (AI) into Anti-Money Laundering (AML) practices. This article explores how AI is reshaping key areas of AML compliance.
1. Revolutionizing Transaction Monitoring: Traditional AML systems, reliant on pre-defined rules, often struggle to detect increasingly sophisticated money laundering techniques. AI, particularly Machine Learning (ML), offers a powerful solution. By analyzing vast volumes of transaction data in real-time, ML algorithms can identify complex patterns and anomalies that deviate from normal customer behavior. These anomalies could indicate potential money laundering attempts, even as criminal tactics evolve. For example, AI can flag unusual sequences of transactions or geographically inconsistent activity, providing valuable insights for further investigation.
2. Streamlining Customer Due Diligence (CDD) and Know Your Customer (KYC): KYC processes are a cornerstone of effective AML compliance. AI can significantly streamline these processes by automating tasks such as customer identity verification, risk profile assessments, and ongoing customer monitoring. Additionally, Natural Language Processing (NLP) technologies play a crucial role. NLP can analyze unstructured data from diverse sources, such as social media and news articles, to identify potentially risky individuals or entities. This comprehensive approach enhances the accuracy and efficiency of due diligence efforts.
3. Improving Suspicious Activity Reporting (SAR): Financial institutions are legally obligated to report suspicious activities to the authorities. AI can automate the generation of Suspicious Activity Reports (SARs) by flagging transactions that match pre-defined risk profiles or patterns indicative of money laundering. This not only improves efficiency but also reduces the number of false positives – a persistent challenge with traditional AML systems.
4. Unlocking the Power of Data Integration and Analysis: AI excels at integrating diverse data sources, including both structured (e.g., financial transactions) and unstructured data (e.g., social media posts). This comprehensive data analysis helps build detailed customer profiles and transaction histories, offering a more holistic view of potential illicit activities. For instance, AI can cross-reference financial transactions with other data points, such as corporate ownership structures and sanction lists, to identify hidden risks that may not be apparent when analyzing data in isolation.
By leveraging the power of AI, financial institutions can significantly enhance their AML compliance efforts, ultimately contributing to a more secure financial system.
Benefits of AI in AML
The integration of AI into AML practices unlocks several key benefits for financial institutions. Firstly, AI automates numerous time-consuming tasks within AML, such as transaction monitoring and customer due diligence. This frees up valuable resources within compliance teams, allowing them to focus on more strategic initiatives. Secondly, AI excels at processing vast amounts of data with exceptional speed and precision. This enables the identification of suspicious activities that might escape human analysts, ultimately leading to a more robust AML framework. Furthermore, AI boasts inherent adaptability. As criminals develop new money laundering tactics, AI systems can continuously learn and adjust, ensuring AML measures remain effective in the face of evolving threats. Finally, by streamlining processes and improving detection accuracy, AI contributes to significant cost reductions within AML compliance operations.
Challenges and Considerations
While AI offers undeniable advantages, its implementation in AML also presents significant challenges. One critical concern is the impact of data quality and availability on AI’s effectiveness. Inconsistent or incomplete data sets can lead AI to generate inaccurate risk assessments and potentially miss suspicious activities altogether.
Furthermore, ensuring regulatory compliance with AI systems adds another layer of complexity. Financial institutions must navigate evolving regulations and standards related to data privacy and the explainability of AI decisions. Regulators are increasingly scrutinizing the use of AI in decision-making processes, requiring institutions to demonstrate transparency and accountability.
Bias within AI models is another potential pitfall. If trained on biased data sets, AI systems can perpetuate these biases in their outputs, leading to unfair or discriminatory outcomes. To mitigate this risk, financial institutions must prioritize transparency in their AI models and conduct regular audits to identify and address potential biases.
Finally, integrating AI with existing AML systems can be a complex and expensive undertaking. Significant investments in technology, training, and ongoing maintenance are required to ensure a smooth and effective integration.
The Road Ahead
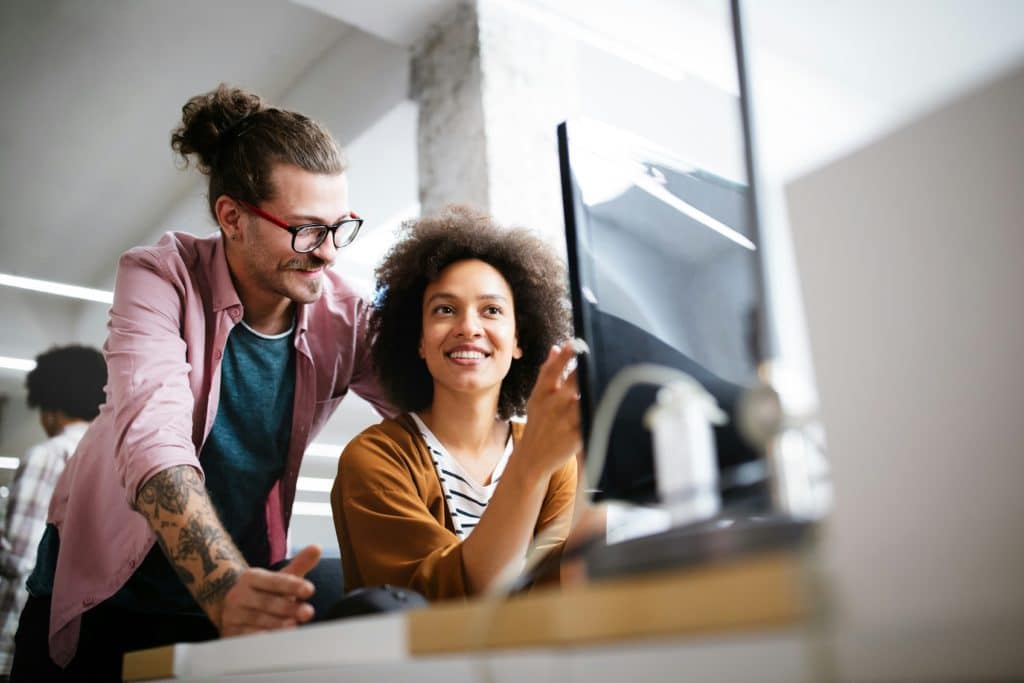
The future of AI in AML is brimming with potential. As technology advances, we can expect even more sophisticated AI algorithms capable of not only identifying suspicious activity but also understanding and predicting human behaviors relevant to money laundering. Enhanced data analytics capabilities will further refine detection methods, and greater integration with global AML initiatives will foster a more unified approach to combating financial crime. Collaboration between financial institutions, technology providers, and regulators will be paramount in shaping these advancements and ensuring their responsible implementation.
Conclusion
AI is undoubtedly revolutionizing the fight against money laundering. Its ability to detect and prevent illicit financial activities with unparalleled efficiency and accuracy offers a significant advantage. While challenges remain, particularly regarding data quality and regulatory compliance, the benefits of AI are undeniable. As technology continues to evolve, AI will undoubtedly become an indispensable tool in the global effort to combat money laundering.