Best Correlation Coefficient Calculator for Accurate Data Analysis
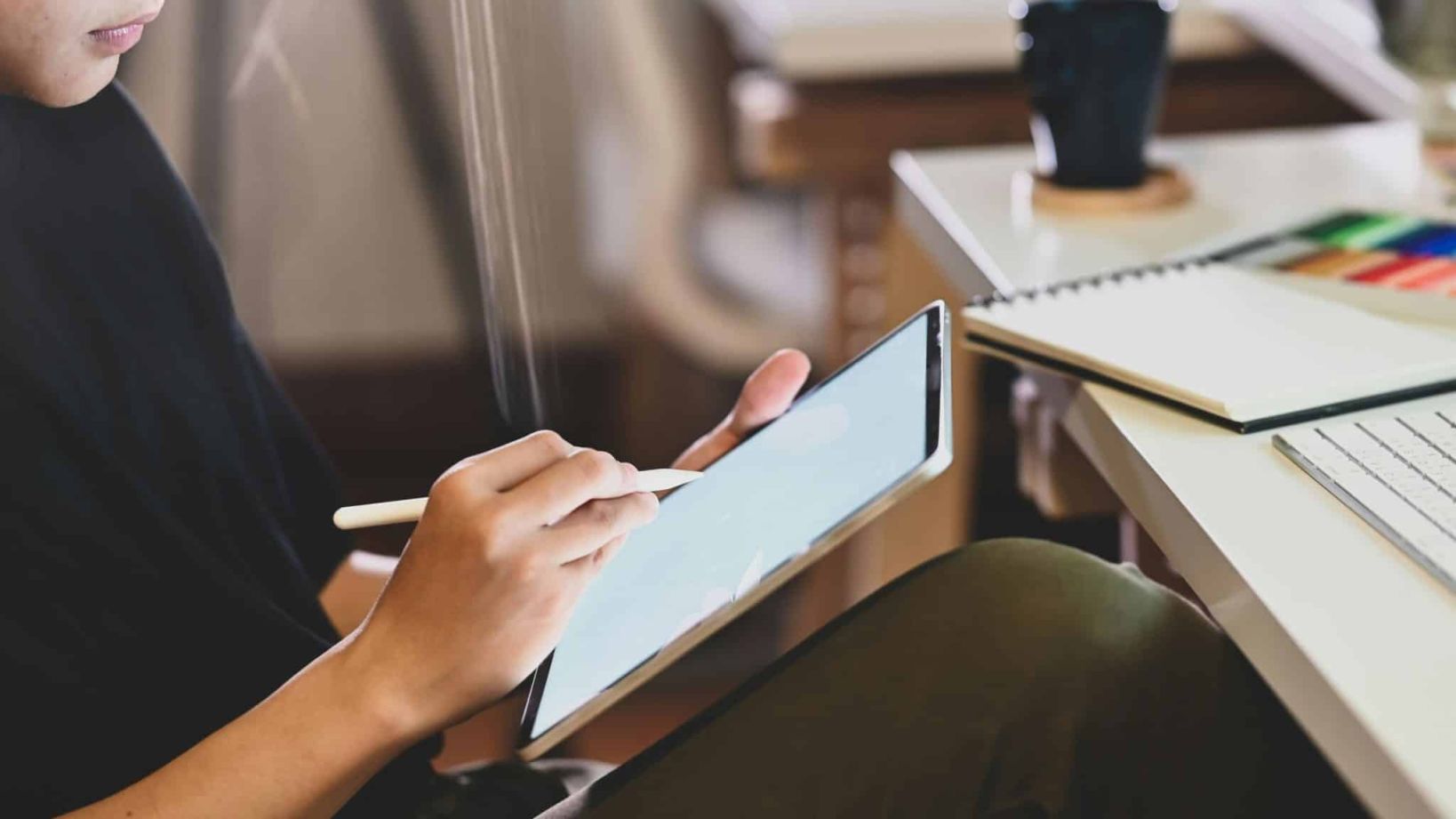
Need to find the relationship between two datasets quickly? A correlation coefficient calculator does just that. This article will guide you on how to use one, what the results mean, and why understanding this value is crucial for your data analysis.
Key Takeaways
Accurate input of data points into a correlation coefficient calculator is critical for obtaining reliable results and understanding the relationship between variables.
The Pearson correlation coefficient quantifies the strength of linear relationships, ranging from -1 to 1. It is calculated using the formula for Pearson’s correlation, which considers the covariance of the variables divided by the product of their standard deviations. However, it is sensitive to outliers and assumes linear relationships.
Different correlation coefficients, such as Spearman’s correlation coefficient, provide alternative approaches for assessing relationships. Spearman’s correlation coefficient is particularly useful for measuring the monotonic correlation between two variables when the data does not meet the assumptions required for Pearson’s correlation coefficient, making it suitable for skewed or non-linear data.
What is the Correlation Coefficient?
The correlation coefficient is a statistical metric that quantifies the strength and direction of the linear relationship between two variables. This dimensionless quantity ranges from -1 to 1, where a value of 1 indicates a perfect positive correlation, meaning both variables increase together in a linear relationship. Conversely, a value of -1 signifies a perfect negative correlation, where one variable increases as the other decreases. A correlation coefficient of 0 indicates no linear correlation, implying that the variables do not have a linear relationship.
Understanding the correlation coefficient is crucial in various fields such as economics, sociology, psychology, and finance. For instance, in finance, it helps in assessing the relationship between different asset returns, aiding in portfolio diversification. In psychology, it can be used to examine the relationship between different behavioral traits. By quantifying the degree of linear association between two variables, the correlation coefficient provides valuable insights into the nature of their relationship, whether it be a perfect positive correlation, a perfect negative correlation, or somewhere in between.
How to Use a Correlation Coefficient Calculator
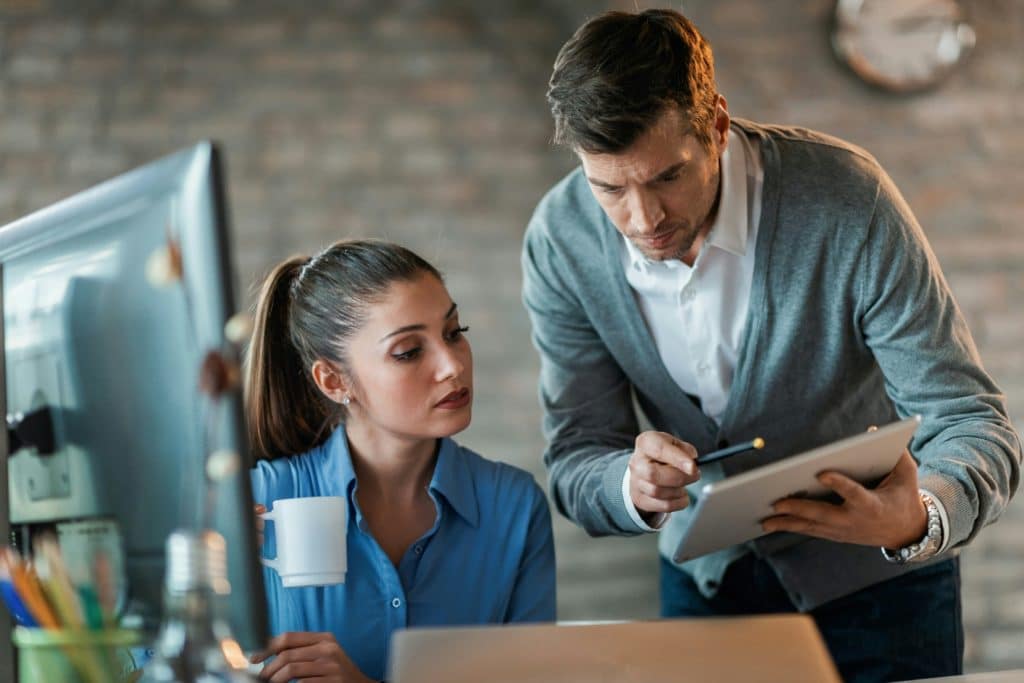
An online tool known as a correlation coefficient calculator streamlines the task of extracting meaningful conclusions from your data. To begin, it is crucial to enter your data points into the calculator with precision because this directly impacts how trustworthy the outcomes will be. Once you’ve inputted values for both sets of variables, simply click ‘calculate’ to obtain the correlation coefficient.
Upon processing your inputted information, the calculator reveals a value indicative of how much and in what manner your variables are related. A positive correlation signifies that an increase in one variable typically coincides with an increase in another, highlighting a direct relationship between them. In contrast, should you observe a negative correlation value after calculation, this would suggest that there is an inverse connection present. Specifically, when one variable rises in value while the other diminishes.
The last phase requires scrutinizing the computed correlation coefficient, which sheds light on not only how strong but also what direction exists within their linear association – whether they move together or oppositely relative to each other. Understanding these dynamics through interpreting this metric facilitates deeper analytical scrutiny and enhances decision-making based on inter-variable interactions within your data set.
Understanding the Pearson Correlation Coefficient
The Pearson correlation coefficient, commonly referred to as Pearson’s R, is a fundamental measure in statistics. This coefficient quantifies the extent of a linear relationship between two variables by assigning it a numerical value that lies within -1 and 1. To compute this value, one divides the covariance between the pair of datasets by their standard deviations’ product. The utilization of such normalized calculations ensures that variable units do not affect the outcome. Understanding how these two metrics interact hinges on analyzing Pearson’s correlation coefficient, which serves as a measure of the linear relationship between the variables.
A perfectly positive correlation is represented by a coefficient with an exact value of 1. This indicates that both variables increase concurrently in perfect unison. Conversely, if the calculation yields -1 as its result, it exemplifies an ideal negative correlation where each variable moves in direct opposition to one another. When there’s no evidence for any kind of linear connection—a scenario often described as zero-correlation—the calculated figure will be at neutral ground: zero itself represents this absence precisely because figures approaching zero hint towards negligible correlations while those verging on either extremity (-1 or +1) suggest markedly stronger ones.
Pearson’s R effectively measures relationships numerically but must be interpreted within context since meaning varies across different research areas and analytical objectives—what constitutes strong correlation like 0.8 might only hold moderate significance elsewhere so consideration should always extend beyond mere numbers.
There are constraints intrinsic to employing Pearson’s R—it operates under assumptions including straight-line interdependence among paired data points along with their distribution adhering strictly according bivariate normal patterns hence distortions from expected norms could easily warp resultant analyses underscoring cautionary usage principles when deploying this particular statistical tool. The validity of using Pearson’s R also relies on whether the data follows a bivariate normal distribution or whether sample sizes are large enough to approximate normality.
Spearman’s Rank Correlation Coefficient
Spearman’s Rank correlation coefficient is a non-parametric measure that assesses the strength and direction of the monotonic relationship between two variables. Unlike the Pearson correlation coefficient, which assesses linear relationships, Spearman’s Rank correlation is particularly useful when the data does not meet the assumptions of normality or when the relationship between the variables is not linear.
To calculate Spearman’s Rank correlation coefficient, the data points are first ranked. Each value in the dataset is assigned a rank, and the correlation coefficient is then calculated based on these ranks. This method makes Spearman’s Rank correlation robust to outliers and suitable for ordinal data or data that does not follow a normal distribution. By focusing on the ranks rather than the raw data, this coefficient provides a clearer picture of the monotonic relationship between two variables, making it a valuable tool in various fields of research.
Example Calculation with a Correlation Coefficient Calculator
Consider a hands-on example to demonstrate the application of a correlation coefficient calculator. Imagine two sets of data, X and Y, which represent the number of hours students have studied and their respective exam scores. By creating a scatter plot, we can visually examine how these two variables might be connected.
The next step is to compute the covariance between both datasets by calculating the mean of each dataset’s deviations multiplied products. After obtaining this covariance value, it is divided by the product of X’s and Y’s standard deviations to yield Pearson’s correlation coefficient. For instance, in our scenario, let us presume that this calculation results in a value of 0.85—indicating there’s typically an increase in test scores alongside increased study hours. Thus reflecting strong positive correlation.
Employing a correlation coefficient calculator makes discerning variable relationships considerably more manageable for users—a testament to such statistical tools’ practicality when dealing with real-world information.
Types of Correlation Coefficients
Despite its widespread adoption, the Pearson correlation coefficient is not the sole technique for gauging relationships between variables. An alternative method, Spearman’s rank correlation coefficient or Spearman’s rho, is particularly valuable when data does not adhere to the prerequisites needed for Pearson correlation analysis. It quantifies both how strongly and in which direction two variables exhibit a monotonic association by examining their rank order. This measure proves advantageous in dealing with non-parametric datasets.
Another important concept is the sample correlation, which is crucial in understanding the statistical properties of bivariate normal distributions. The sample correlation coefficient helps in identifying biased estimates and is significant in regression models and correlation interpretation. Mathematical formulations can derive the adjusted correlation coefficient, enhancing its application in various statistical analyses.
Kendall’s tau represents yet another approach to assessing rank correlations that some prefer due to its suitability for smaller datasets. This metric considers pairs of observations and determines the relationship strength between two variables based on their agreement or disagreement.
For instances where one variable takes on binary values while the other remains quantitative, researchers employ point-biserial correlation as it elucidates how these different types of variables interrelate—the former being binary and the latter continuous. When handling nominal variables, Cramér’s V emerges as an essential tool. It clarifies how strong categorical attributes correlate with each other.
Being acquainted with various types of correlation coefficients enables scholars to pinpoint the most fitting analytical method tailored to their specific set of data—a decision crucial for ensuring precision and substantial insights within research findings given different dataset characteristics and investigative queries.
Importance of Sample Size in Correlation Calculations
The reliability of correlation calculations is heavily dependent on sample size. When the sample size increases, the results become more stable and trustworthy, minimizing potential sampling errors. Larger samples are better representations of the overall population, which leads to sharper estimates of population parameters.
As you increase your sample size, there tends to be a closer alignment between correlation coefficients and the actual value within the population. This tight convergence minimizes how far off a sample’s correlation may deviate from that true existing in a larger group—thereby increasing result precision. On the other hand, limited samples lead to broader confidence intervals. These widen uncertainty around estimated correlations due to increased vulnerability to random variations in data.
To obtain accurate estimations of correlations, it is essential for researchers to calculate necessary sample sizes using proper statistical power analysis while considering desired widths for confidence intervals. Such practices ensure that study outcomes are both reliable and applicable when extrapolated onto broader populations.
Deriving Pearson correlation values based on smaller-sized samples might not reflect an accurate portrayal of those same values at large—this underlines why ample sizing is integral during research planning stages.
Interpreting Correlation Coefficient Values
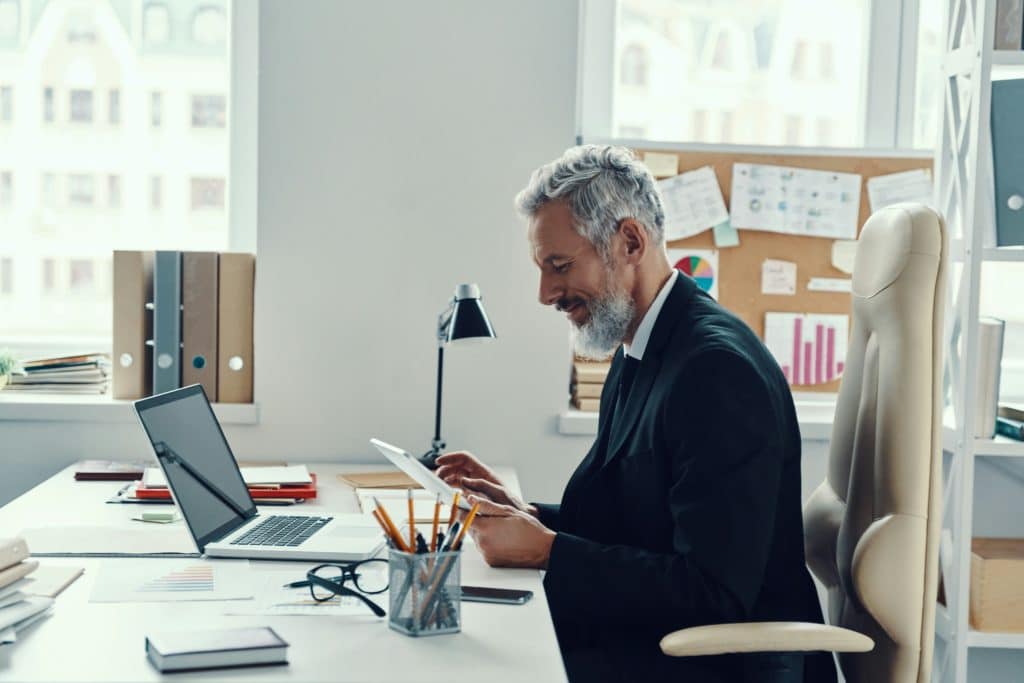
Understanding the values of correlation coefficients is essential in examining the association between variables. A correlation coefficient calculator Presents a value ranging from -1 to 1, which reveals both how strong and in what way two variables are related. A perfect positive linear relationship is indicated by a +1 value, where an increase or decrease occurs simultaneously in both variables. On the flip side, a -1 value denotes a perfect negative relationship, with one variable rising as the other falls consistently.
Values that approach zero indicate an absence of any notable linear connection between two sets of data—this situation is recognized as zero correlation. It’s important to acknowledge that while zero correlation points to no discernible linear linkage, it doesn’t inherently rule out all forms of relationships.
These metrics shed light on the character and potency of interactions among different factors within datasets. For instance, detecting only minor trends would suggest weak correlations. While discovering pronounced patterns indicates stronger links between elements under study. Such precise insights empower researchers to derive significant interpretations from their collected information and make choices backed by clear evidence concerning observed relational strengths and orientations.
P-Value and Correlation Coefficient
The p-value is a statistical measure that helps determine the significance of the correlation coefficient. It indicates the probability of observing a correlation coefficient at least as extreme as the one calculated, assuming that there is no actual correlation between the variables. In other words, the p-value helps assess whether the observed correlation is likely to be due to chance.
Typically, a p-value threshold of 0.05 is used to determine statistical significance. If the p-value is less than 0.05, the correlation coefficient is considered statistically significant, suggesting that the observed relationship between the variables is unlikely to have occurred by random chance. To calculate the p-value, various statistical tests can be employed, such as the t-test or the Fisher transformation.
Understanding the p-value in the context of the correlation coefficient is essential for interpreting the results of data analysis. A statistically significant correlation coefficient, accompanied by a low p-value, provides stronger evidence of a meaningful relationship between the variables, enhancing the reliability of the conclusions drawn from the data.
Limitations of the Pearson Correlation Coefficient
The Pearson correlation coefficient, while widely used, has notable restrictions. Its scope is confined to the detection of only linear relationships, overlooking significant connections when dealing with non-linear patterns. This limitation renders the Pearson correlation inadequate for recognizing non-linear correlations and constrains its usefulness in various contexts.
This metric also displays a high degree of susceptibility to outliers. Outliers can skew the results significantly due to this sensitivity, compromising the robustness of the Pearson correlation coefficient’s outcomes. Consequently, even one outlier holds enough influence over this statistic to potentially result in incorrect conclusions being drawn from data analyses.
It’s critical to understand that possessing a substantial Pearson correlation coefficient isn’t synonymous with having an underlying linear relationship. Other forms like quadratic or distinct patterned associations might exist that evade detection by Pearson’s R alone. Given these caveats regarding usage scenarios and alternative considerations when faced with non-linearity or datasets impacted by outliers, it underscores responsible application practices involving quantitative assessments such as these.
Using Software for Correlation Calculations
In the realm of data analysis, software tools play a critical role in computing correlations. The cor() function within R is particularly useful for calculating correlation coefficients with numeric vectors. This function’s flexibility to manage multiple types of correlation calculations makes it highly valuable to both researchers and analysts.
Similarly, Python offers potent libraries such as NumPy, SciPy, and pandas that come equipped with functions designed for computing different kinds of correlation coefficients. Specifically, the.corr() method in pandas allows users to construct a correlation matrix within DataFrames which provides an extensive overview of how datasets interrelate.
For more tailored calculation needs, SciPy includes functions like pearsonr(), spearmanr(), and kendalltau(), each dedicated to evaluating specific types of correlation coefficients.
Utilizing these sophisticated software instruments is essential for precise computation of correlation coefficients during data analysis tasks. They significantly simplify the process while boosting accuracy and consistency—facilitating more productive and thorough analyses.
Advanced Topics in Correlation Analysis
For those delving deeper into the analysis of correlations, advanced topics such as adjusted, weighted, and partial correlations provide a more nuanced understanding. Specifically, the adjusted correlation coefficient delivers more precise estimates for large datasets by taking into consideration the quantity of variables and predictors involved. This refinement helps in assuring a more reliable quantification of how strongly related the variables are.
In situations where certain observations carry greater significance within a dataset, weighted correlation coefficients come into play. By assigning various weights to individual data points, this method enables an analysis that accurately reflects each observation’s relative importance.
Meanwhile, partial correlation offers insight into the direct relationship between two variables while simultaneously controlling for additional factors. It isolates their connection from other influences which may affect it—clarifying what is otherwise obscured when multiple variables interact with one another.
Adjusted Correlation Coefficient
By taking into account both the sample size and the quantity of predictors, the adjusted correlation coefficient delivers a more reliable indicator of relationship strength. It revises the conventional correlation to compensate for how many variables there are in relation to the size of your sample, which results in a truer estimation.
When it comes to large datasets, where typical measures of correlation may fall short on reliability, this refined calculation provides an improved representation of linear relationships between variables. The adjusted correlation coefficient’s attention to these aspects makes it particularly useful for studies with extensive data sets.
Weighted Correlation Coefficient
The weighted correlation coefficient takes into consideration the differing relevance of observations in a dataset by applying a weight vector that gives various weights to data points according to their importance. This technique enables a more refined analysis by accentuating specific observations, thereby improving the precision of the correlation measure.
In situations where not all observations carry equal value—for example, when some points are more trustworthy or vital within a dataset—the use of weighting ensures these significant points exert greater influence on the calculation of correlation. This results in an analysis that is both customized and exacting.
Partial Correlation
Partial correlation is a method used by researchers to examine the relationship between two variables while accounting for the impact of other variables. This technique calculates how strongly connected two variables are by focusing solely on their direct association and excluding the effects of any additional factors.
This technique enhances comprehension of the true connection between the analyzed variables by eliminating external variable influences, making it particularly valuable in multifaceted datasets with interacting elements. It provides a more precise depiction of straightforward relationships present within data sets.
Summary
To summarize, calculators for determining the correlation coefficient are vital in the realm of data analysis as they provide a means to measure and comprehend the interplay among different variables. Acquiring proficiency in their application—from entering data to making sense of outcomes—is crucial for researchers and those analyzing data. The Pearson correlation coefficient is central to statistical assessments, offering perspectives on linear correlations while also having inherent restrictions. By acknowledging these boundaries and incorporating other forms of correlation like Spearman’s rho or Kendall’s tau into our toolkit, we enhance our analytical capabilities.
Delving deeper into correlation studies with topics such as adjusted, weighted, and partial correlations gives rise to more refined scrutiny that is key when engaging intricate datasets from which one seeks significant conclusions. Grasping these advanced concepts aids us in addressing complex sets of data effectively. Utilizing computational tools available within R or Python programming languages allows us not only expediently but also accurately carry out these computations thereby ensuring precision within our investigative endeavors. In persistently pursuing knowledge about—and applying—these advanced techniques, we tap into the latent power housed within our datasets. This empowers sound decision-making processes alongside novel discoveries.
Frequently Asked Questions
What is the Pearson correlation coefficient?
The Pearson correlation coefficient, commonly known as Pearson’s R, quantitatively assesses the strength and direction of the linear relationship between two variables. This coefficient ranges from -1 to 1, where values close to 1 indicate a strong positive correlation, values near -1 indicate a strong negative correlation, and values around 0 suggest no linear correlation.
How do I use a correlation coefficient calculator?
To effectively use a correlation coefficient calculator, accurately enter your data points for both datasets and click ‘calculate’ to receive the correlation coefficient value.
This process provides insight into the relationship between the two sets of data.
What are the limitations of the Pearson correlation coefficient?
The correlation coefficient known as the Pearson correlation is notably limited by its susceptibility to outliers and its narrow concentration on linear correlations, which may cause it to miss non-linear relationships.
Why is sample size important in correlation calculations?
Sample size is crucial in correlation calculations as larger samples enhance the reliability of estimates by minimizing sampling errors and yielding more stable results.
Therefore, a well-calibrated sample size is essential for accurate correlation analysis.
What is partial correlation?
Partial correlation measures the direct relationship between two variables by controlling for the influence of other factors, ensuring that the connection observed is purely between the two variables in question without any outside disturbances.