Best Variance Calculator for Accurate Data Analysis
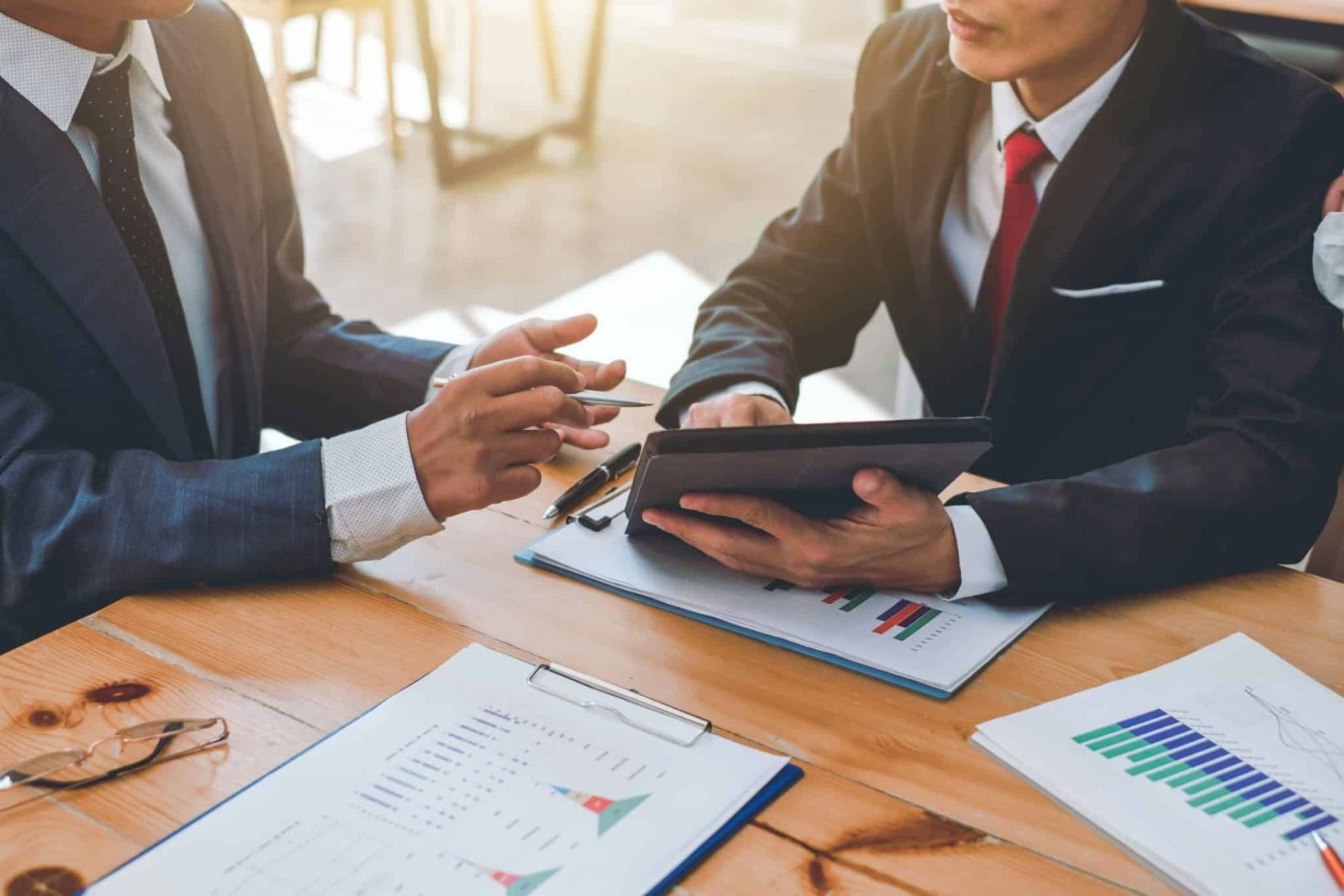
A variance calculator helps you quickly find how much your data varies from the mean through statistical analysis. Enter your numbers, and get instant results for variance and other key stats. This tool saves time and ensures accuracy in data analysis.
Key Takeaways
A variance calculator automates the calculation of key statistical measures, enhancing efficiency and accuracy in data analysis.
Variance quantifies the spread of data points from the mean, providing critical insights in fields such as finance, quality control, and academic research.
Proper understanding of population vs. sample variance is essential for accurate analysis, with different formulas applied based on the dataset’s context.
How to Use a Variance Calculator
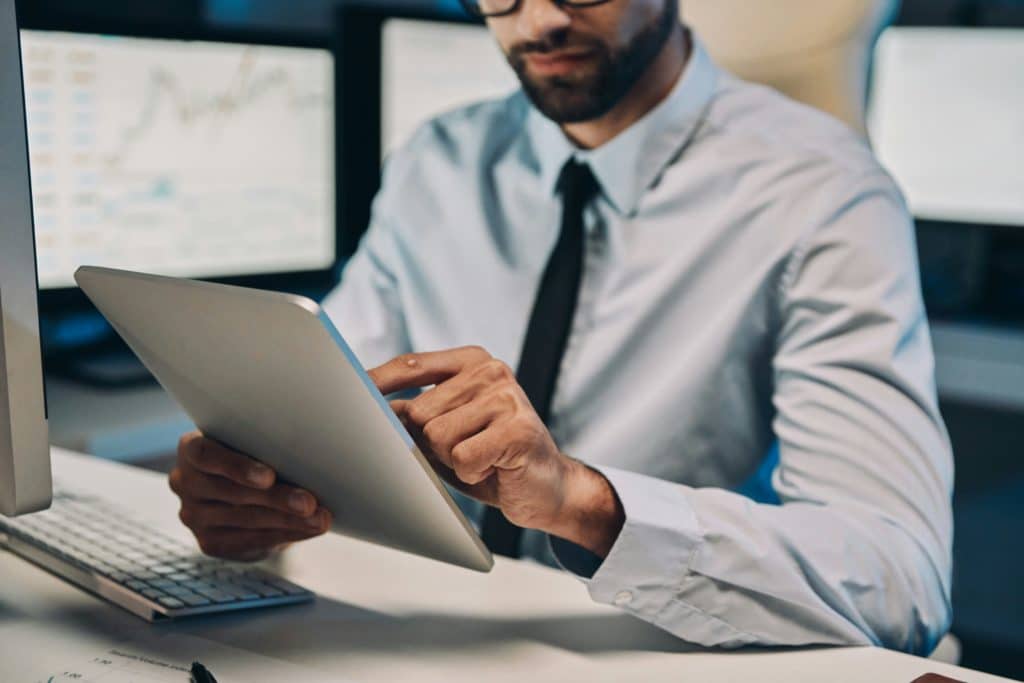
A variance calculator streamlines your analysis of data by automating the process. Its key function is to assess how far each individual value within a dataset deviates from the average, offering an accurate measure of how spread out the data values are. This tool is immensely useful for analyzing both compact and large collections of figures, helping to save time and minimize errors associated with manual computations. Inputting your data into the calculator is straightforward—you can type in values separated by spaces, commas or line breaks or simply copy and paste them directly from other documents like spreadsheets.
After you input your sample dataset into the tool, it will execute calculations to determine various statistical measures such as variance, standard deviation, mean (average), sum of squares related to those values, and overall sample size. Utilizing this technology proves especially valuable in sectors that require precise analyses like finance or quality control operations as well as scholarly investigations.
Leveraging a variance calculator enables automatic handling of intricate mathematical processes involved in understanding datasets, which allows users more bandwidth to concentrate on deciphering the outcomes they produce. The immediacy with which this device relays insights bolsters productivity while also swiftly revealing any anomalies present—facilitating prompter action based on those findings.
Understanding Variance
Variance is a statistical measure that captures data variability by showing how much individual numbers in a data set differ from the average value, offering insightful perspectives on the dispersion of data. It’s determined by taking the mean of squared deviations from this average, which helps gauge the consistency and dependability of data points. A variance calculator tool can assist by providing vital details such as standard deviation, size of the sample under consideration, its mean value, and summing up squares for comprehensive analysis.
The calculation process for variance adapts depending on whether you’re examining an entire population or merely a sample extracted from it. This distinction plays an essential role because it alters not only computation methods but also influences how results are understood.
By revealing whether your samples exhibit tight clustering around their average (indicative of low variance) or if they’re spread out over a wider range (suggesting high variance), you obtain critical information about variation within your dataset. Such insights prove especially valuable in sectors like finance where understanding risk levels and predicting fluctuations hinge upon assessments derived from measures like variance.
What is Variance?
Variance indicates the extent to which data points in a dataset deviate from their average value. It is determined by taking the mean of squared differences between each point and the mean, thereby making all deviations non-negative and giving more weight to larger ones. This yields a measure of variability that’s essential for statistical modeling and analysis expressed as squares.
There are two main categories of variance: population variance, denoted by sigma squared (2), encompasses every data point within an entire population. Whereas sample variance includes just a portion of those values from the broader group. To achieve an unbiased estimate with sample variance, one subtracts 1 from the total number of data points (n – 1) during calculation. Understanding this distinction is vital for precise evaluation and interpretation of data.
Why Variance Matters
Variance is important as it provides a squared measure of data variability, crucial for understanding data spread and making statistical inferences. In investment analysis, variance measures potential risk and volatility, aiding informed decision-making. A higher variance indicates greater variability in data points, suggesting that values are more dispersed, while a lower variance suggests that data points are closely clustered around the mean.
Variance is also crucial in quality control to identify inconsistencies in manufacturing outputs and in academic research to analyze experimental data variability.
Ultimately, variance gives a clear picture of how data points differ from the average, making it a powerful tool across various domains.
Steps to Calculate Variance
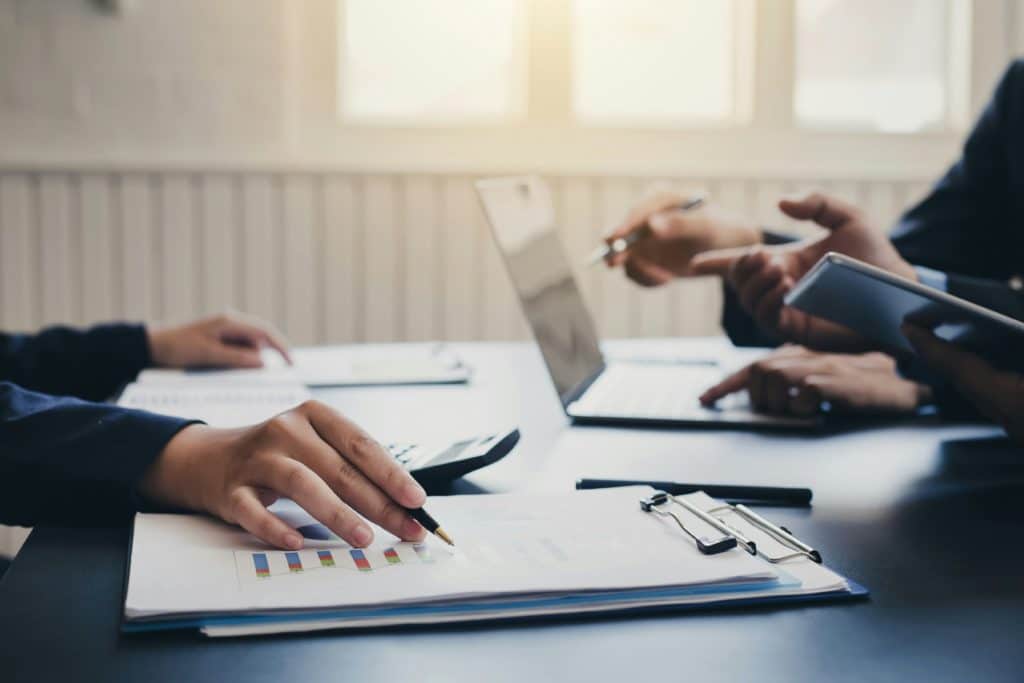
The process of calculating variance is designed to ensure both precision and dependability in the analysis of data. It involves determining the average value (mean), computing how much each data point deviates from that mean, summing up these squared deviations, and then dividing by either N or N-1 depending on whether you’re dealing with population or sample variance respectively. Each of these steps is crucial for accurately depicting how widely distributed the data points are around their central value, setting a solid foundation for more complex analytical tasks.
By adhering to this methodical approach when calculating variance, we can gain deeper insights into the consistency and reliability inherent within our dataset. This technique holds particular importance for individuals in fields such as research analysis, quality control management, and financial investigation.
We will delve into every step involved meticulously to cement a comprehensive grasp on executing precise calculations of variance.
Step 1: Find the Mean
The initial step in calculating variance is finding the mean, the central value of a dataset. Sum all data values and divide by the total number of data points to find the mean. The formula is: Mean = (Sum of all data values) / (Total number of data points). The mean is fundamental for statistical analyses, serving as the baseline for measuring data dispersion.
For example, calculating the mean of monthly sales figures gives the average monthly sales. This average serves as a reference point to see how each month’s sales figures deviate from the norm. Thus, finding the mean is crucial for understanding the overall trend and variability of your data.
Step 2: Calculate Deviations from the Mean
To determine how much each data point differs from the mean, one must subtract the average value from every individual value. This process reveals the deviation for each point by calculating the difference between that specific data point and the mean. For instance, if we have a mean of 10 and a particular data value is 12, then this results in a deviation of 2 because you deduct 10 (the mean) from 12 to get your answer.
It’s critical to measure these deviations since they are used as foundational elements when it comes time to calculate variance. By analyzing all variations from the average across your dataset, you can assess its overall range and inconsistency. Every deviation needs precise calculation so that subsequent steps based on these figures—like computing variance—are accurate and reflective of your data’s true spread.
Step 3: Sum the Squared Deviations
Begin by taking each deviation, squaring it to transform all values into positive ones and to highlight the greater deviations. This action is critical for reliably assessing how much the data varies. By aggregating these squared differences, you obtain an aggregate sum of squares (SS), which serves as a thorough gauge of data spread.
To illustrate, consider deviations such as 2, -3, and 4. Their corresponding squared figures would be 4, 9, and 16 respectively. The aggregation of these squared variations yields the total SS—this figure plays a pivotal role in calculating variance. Such summation ensures that every difference is considered and set up for subsequent steps in the computation process.
Step 4: Divide by N or N-1
To calculate the population variance, take the sum of squares (SS) and divide it by N, which represents the total number of data points. The formula is expressed as: Variance = SS / N. In contrast, when computing variance for a sample from this data, subtract one from N to arrive at an unbiased estimation. Thus the formula becomes: Variance = SS / (N-1).
Employing Bessel’s correction by using N-1 accounts for underestimation in variability that can occur when sampling from a population. This adjustment helps maintain precision and dependability within your calculations, whether you’re assessing sample or full population datasets.
Population Variance vs. Sample Variance
Understanding the distinctions between population variance and sample variance in a data set is essential for precise analysis of data. The population variance applies to data covering the entire population, offering an exact measure of how much variability exists within it. On the other hand, when dealing with a subset or sample from that population, we use sample variance which includes an adjustment in its computation to account for an underestimation of the true variability found across the whole population.
Both categories employ distinct formulas tailored to their respective needs. For calculating population variance, one uses 2 = SS/N where SS represents the sum of squares derived from all differences and N denotes every individual piece within our dataset.
Conversely, computing sample variance requires modifying this approach using: Variance = SS / (N-1), wherein (N-1) compensates for smaller samples sizes ensuring that estimates remain unbiased and reflective of actual conditions. Mastery in choosing and correctly applying these specific formulas is imperative for conducting analyses accurately.
Population Variance Formula
Use the population variance formula when analyzing the entire population to get a precise measure of variability. The formula is: 2 = SS/N, where 2 is the population variance, SS is the sum of squares, and N is the total number of data points. This formula accounts for every data point, accurately measuring data value deviation from the mean, including the sample standard deviation and the population standard deviation.
To calculate SS, use: SS = sum(xi – mu)2), where xi represents each data point and mu is the population mean. This involves squaring each deviation from the mean and summing them up.
Finally, divide the sum of squares by the number of observations (N) to get the population variance. This method accounts for all data points, providing a comprehensive measure of variability.
Sample Variance Formula
When dealing with a subset of sample data from the larger population, you should apply the sample variance formula. The equation to use is: Variance = SS / (N-1), where this adjustment—commonly referred to as Bessel’s correction—compensates for potential underestimation of the true variability within a population by using N-1 instead of just N in its calculation. To obtain the sample standard deviation, simply extract it from this variance formula.
To figure out the sum of squares (SS) relevant to your sample, employ essentially identical steps as those used when computing population variance: SS = (xi – x)2). Here, “xi” signifies every individual piece of data and “x” symbolizes the average value within your sample. First determine your sum of squares. Then divide that amount by N-1 in order to arrive at an accurate and reliable estimation for your sample’s variance. Following these instructions will give you precise results even when working with limited datasets.
Standard Deviation and Variance Relationship
Understanding the Connection
The standard deviation and variance are two closely related statistical measures that describe the spread or dispersion of a data set. Variance is calculated by taking the average of all the squared differences between each data point and the mean. This gives us a measure of how much the data values deviate from the mean, but in squared units. To bring this measure back to the original units of the data, we take the square root of the variance, which gives us the standard deviation.
In simpler terms, while variance gives us a sense of the overall spread of the data points, the standard deviation provides a more intuitive measure of this spread by expressing it in the same units as the data values themselves. For instance, if you have a data set of test scores, the variance will tell you how much the scores vary in squared units, whereas the standard deviation will tell you the average deviation in the same units as the test scores.
Understanding this connection is crucial for accurate data analysis, as it allows you to interpret the variability of your data set more effectively. If you know the variance, you can easily find the standard deviation by taking its square root, and vice versa.
Using a Variance Calculator
Employing a sample variance calculator facilitates handling intricate data analysis tasks in a practical and time-efficient manner. You have the option to enter your set of data values into the tool, which accepts formats that include spaces, commas, or line breaks. This adaptability streamically integrates with documents or spreadsheets for inputting information swiftly and diminishes the need for laborious manual entry. Once inputted, the calculator rapidly provides essential statistical measures such as variance, standard deviation, sample size mean value per observation (mean), along with sum of squares calculation, offering an extensive suite for prompt assessment and comprehension.
The automated process provided by a variance calculator serves not only to conserve valuable time but also reduces human error potential. Its efficiency becomes exceptionally advantageous when modifications are required on-the-fly. It enables immediate recalculation without delay.
Utilizing a variance calculator guarantees precision and expediency in analyzing either population-based or sample-specific datasets. As you employ this tool within various professional contexts, it allows greater concentration on interpreting meaningful conclusions from analyzed results instead of being mired down by complex calculations.
Standard Deviation Calculator
A standard deviation calculator is an invaluable tool for quickly determining the standard deviation of a data set. Whether you’re working with population data or a sample data set, this calculator simplifies the process. For population data, it uses the population standard deviation formula, and for sample data, it employs the sample standard deviation formula.
To use the calculator, simply input your data values, and it will compute the standard deviation for you. This tool is particularly useful for comparing the variability of different data sets, as it provides a clear measure of how spread out the data points are around the mean. In fields like finance, quality control, and academic research, understanding the standard deviation is essential for making informed decisions based on data analysis.
Benefits of a Variance Calculator
Utilizing a variance calculator offers multiple advantages that are essential for anyone engaged in the field of data analysis. These key benefits include:
A substantial decrease in potential human errors, leading to calculations with greater precision.
Vital accuracy when working with extensive datasets or intricate statistical models where small miscalculations can skew results significantly.
The automation provided by such calculators guarantees the consistency and exactness of your outcomes.
Beyond enhancing calculation accuracy, a variance calculator also improves efficiency by facilitating quick determination of how far apart data points lie from their average value. This enables users to allocate more time to analyzing what these results signify rather than getting caught up in executing complex computations.
Automated tools for calculating variance deliver immediate insights into any anomalies present within the dataset, promoting faster decision-making and enhanced proficiency in examining data patterns. In essence, employing a variance calculator not only streamlines demanding mathematical tasks, but also bolsters superior statistical investigation practices.
Applications of Variance in Different Fields
Data analysis is a critical measure widely utilized in various domains, highlighting its significance for analyzing data. Within the realm of finance, variance plays an indispensable role in gauging the potential risk tied to the returns on investment, thus aiding investors to more adeptly oversee their portfolios. It quantifies asset price volatility and yields insight into both stability and prospective gains from investments.
In terms of quality control efforts, computations of variance aid in detecting irregularities within manufacturing processes, which pave the way towards enhancing product uniformity and excellence. Academic researchers depend heavily on variance when dissecting experimental data as it aids them in comprehending fluctuations within their findings.
Within machine learning disciplines, employing variance proves crucial for evaluating algorithmic performance while preventing overfitting. This ensures algorithms’ robustness with new datasets they encounter. Variance calculators facilitate these endeavors by streamlining intricate calculations and depicting data visually — heightening efficiency as well as precision throughout these applications.
Advanced Topics in Variance
Homogeneity of Variance in Statistical Tests
Homogeneity of variance, also known as homoscedasticity, is a fundamental assumption in many statistical tests. It refers to the condition where the variance of a dependent variable is consistent across all levels of an independent variable. In simpler terms, it means that the spread or dispersion of data values is uniform across different groups or categories.
Ensuring homogeneity of variance is crucial for the validity of statistical tests. If the variance is not equal across groups, the results of the test may be biased or misleading. To assess this assumption, several statistical tests can be employed, such as the F-test and Levene’s test. These tests help determine whether the variance is equal across all groups, ensuring the reliability of the test results.
In cases where the assumption of homogeneity of variance is violated, data transformation methods like logarithmic transformation, square root transformation, and standardization can be used to meet this assumption. These methods adjust the data to make the variance more uniform across groups, thereby enhancing the validity of the statistical analysis.
Understanding and ensuring homogeneity of variance is essential for accurate and reliable statistical analysis. By using appropriate tests and transformations, you can ensure that your data meets this critical assumption, leading to more valid and trustworthy results.
Common Mistakes in Variance Calculation
Calculating variance is a critical task, yet it’s often marred by common errors that can distort the outcomes. Understanding data variability is essential to ensure accurate variance calculations. A primary oversight occurs when there isn’t a clearly established baseline against which to measure variations. Without this consistency, the resulting variance might not truly capture how much the data varies.
Mistakenly applying inappropriate formulas for either population or sample variance constitutes another widespread issue. Utilizing an incorrect formula could yield deceptive findings, underscoring the importance of choosing correctly based on what your data analysis entails.
To mitigate such inaccuracies, employing a variance calculator can be quite beneficial. It automates computing processes and ensures proper application of relevant formulas—thus heightening result precision while diminishing manual calculation efforts.
By grasping these frequent missteps and steering clear of them, you’ll enhance both the exactness and dependability of your calculated variances in analyses involving diverse sets of data.
Summary
To summarize, the grasp of variance and its precise calculation is essential for proficient statistical analysis in diverse disciplines. Employing a calculator designed to compute variance simplifies this process, diminishes error probability, and allows analysts to concentrate on interpreting outcomes. Variance serves as an influential indicator of data variability whether utilized in evaluating investment risks, certifying product quality or scrutinizing experimental findings. Leveraging the capabilities of calculators that measure variance can bolster your analytical endeavors and guide you toward well-informed choices grounded on reliable statistical measures.
Frequently Asked Questions
What is the difference between population variance and sample variance?
Population variance is calculated using every data point within a data set, while sample variance adjusts the calculation by using n – 1 instead of n to compensate for the smaller subset of data points, providing an unbiased estimation.
Why is it important to calculate variance?
Determining the variance is crucial in data analysis since it provides insight into how data are dispersed and their level of uniformity, which is vital for making educated choices in pivotal sectors like finance, research, and quality management.
Comprehending the variance enhances the ability to manage risks effectively and devise more sophisticated strategies.
How does a variance calculator help in data analysis?
By automating intricate computations and reducing the chance of human error, a variance calculator improves data analysis by providing essential statistical measures. It allows users to quickly acquire precise results that aid in the more effective interpretation of data.
Can I use a variance calculator for both population and sample data?
Yes, a variance calculator can be used for both population and sample data values, applying the relevant formulas for accurate results.
What are some common mistakes to avoid when calculating variance?
To avoid common mistakes in calculating variance and understanding data variability, ensure you define the baseline for comparison clearly and use the correct formulas.
Utilizing a variance calculator can also help minimize such errors.