Mastering Monte Carlo Simulation Portfolio Optimization for Smarter Investments
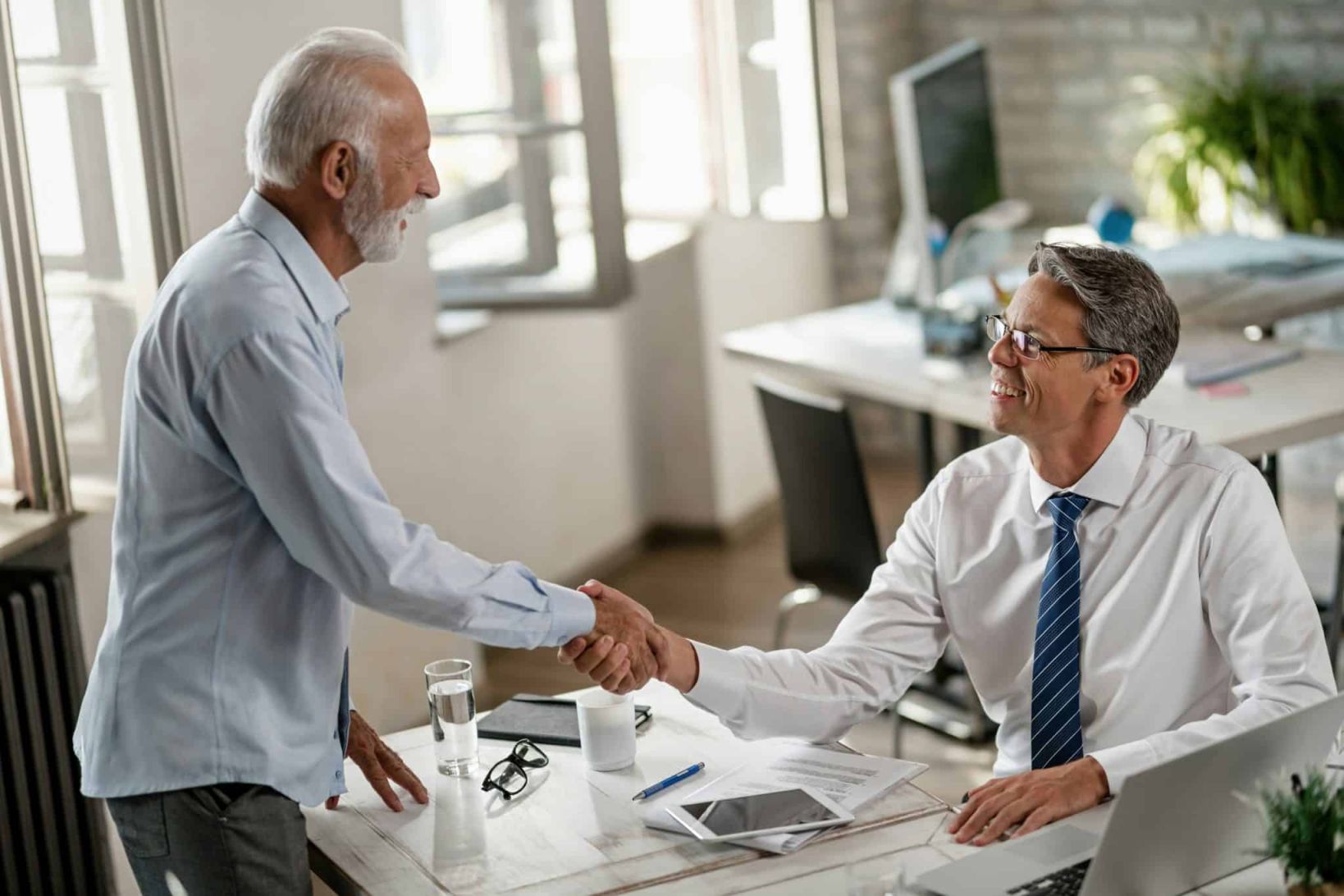
Monte Carlo Simulation optimizes portfolios by simulating thousands of possible future scenarios. By incorporating expected volatility, which influences the calculations of expected returns and risk-adjusted metrics, investors can better understand the trade-off between risk and return. This method helps predict returns and risks, making asset allocation more efficient. This article details how Monte Carlo Simulation works in portfolio optimization, including data gathering and risk analysis, specifically focusing on the Monte Carlo simulation portfolio optimization process.
Key Takeaways
Monte Carlo Simulation (MCS) helps analyze possible investment scenarios, balancing risk and return for effective portfolio optimization.
The quality of input data, such as historical asset prices, is critical for accurate simulation results and informed investment decisions.
Visualizing the efficient frontier using MCS enables investors to identify optimal asset allocations that maximize returns while mitigating risks.
The risk free rate is essential in calculating the Sharpe Ratio, which compares investment returns to risks. Adjusting the risk free rate helps optimize portfolios under varying market conditions, assessing the effectiveness of riskier assets compared to safer ones.
Understanding Monte Carlo Simulation in Portfolio Optimization
Monte Carlo simulation is a method that utilizes repeated random sampling to evaluate and forecast potential investment outcomes. This technique plays a vital role in portfolio optimization, where the objective is to determine an asset allocation strategy that both maximizes returns and minimizes risk. By conducting numerous simulations, investors can explore different scenarios and improve their strategic choices.
The challenge of optimizing a portfolio lies in managing various elements and risk considerations to create an investment mix aimed at enhancing return or reducing exposure to risk. Even small adjustments in how assets are distributed within the portfolio can substantially alter its performance. The Monte Carlo simulation stands out for its capacity to test diverse strategies concerning asset allocation by projecting future risks as well as possible gains.
Monte Carlo simulations can be used to determine the optimal weights for a given portfolio by analyzing mean returns, risk, and covariance associated with the assets.
Utilizing Monte Carlo simulation offers significant advantages when striving for optimal portfolios because it allows projection of future profits using historical data sets. Selecting past yearly returns at random paired with statistical modeling provides insights into how variable the earnings from a portfolio could be, which illuminates broader perspectives on the associated risks and benefits linked with distinct investing tactics.
Ultimately, the use of Monte Carlo simulation acts as a conduit connecting theoretical principles from modern portfolio theory with tangible investment practices. By applying random sampling coupled with meticulous statistical analysis, investors gain valuable assistance navigating through complex decisions about asset distribution—enabling smarter choices that carefully weigh both risks against expected rewards.
Gathering Security Data for Analysis
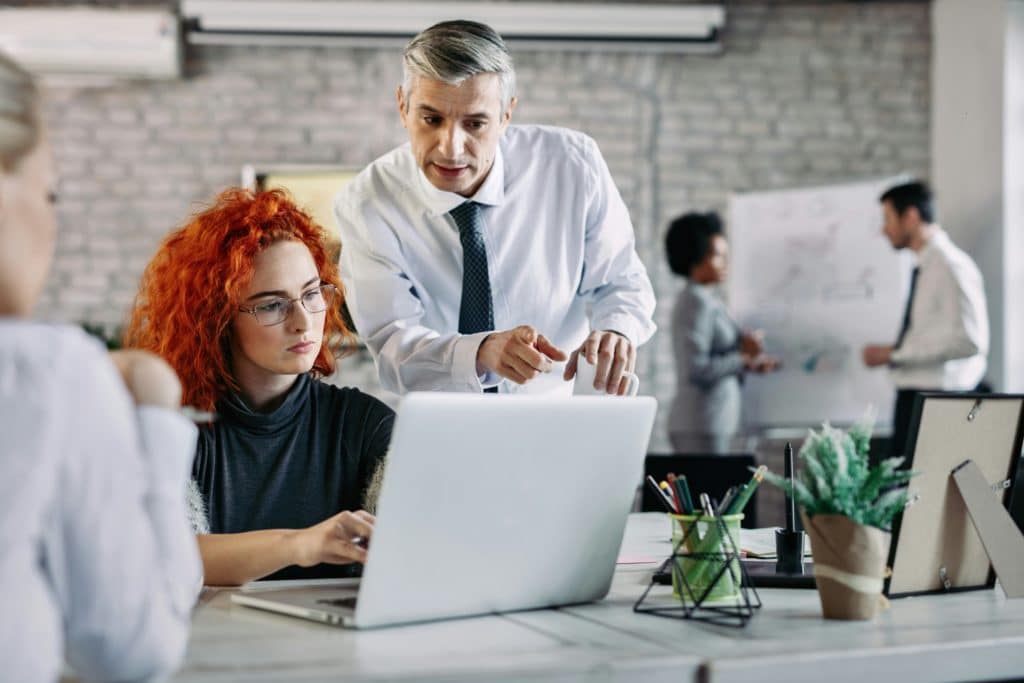
The success of employing Monte Carlo Simulation for portfolio optimization is heavily dependent on the caliber of input data used. Accurate data that offers a window into the past performance of different assets plays an essential role in producing precise simulations. For our assessment, we incorporated adjusted closing prices from a varied selection of assets, such as stocks and gold, to achieve a thorough appraisal.
We sourced this information by utilizing the Alphavantage API, which yielded historical price points covering January 1, 2018 through January 1, 2023. The breadth of this dataset equipped us with the ability to represent diverse market scenarios and trends effectively within our simulation models. Our stock price analysis centered around major technology corporations including Apple, Microsoft Alphabet (Google), Amazon and Tesla.
Laying down an accurate and relevant database was imperative for conducting reliable Monte Carlo simulations – indispensable because inaccuracies in data can result in deceptive outcomes leading to less than ideal investment choices.With credible input secured, we’re now positioned to model potential portfolio returns under various asset allocation strategies using Monte Carlo methods.
Simulating Portfolio Performance
The Monte Carlo Simulation (MCS) enables the examination of random return fluctuations by creating a multitude of hypothetical market conditions using assumptions about asset volatility and interrelationships. By employing past performance data, MCS is capable of predicting future financial outcomes through randomly generated yearly returns, offering an authentic representation of what investors might expect. This technique involves producing returns that are parameterized, which means setting particular statistical distributions for different assets to help project probable earnings and associated risks.
By executing thousands of these simulations, we can gain insight into the potential range in portfolio performance with each iteration picturing a distinct possible future state. The method not only sheds light on anticipated yields, but also delineates related uncertainties, empowering investors with enhanced knowledge for their decision-making processes. Various models — historical ones reflecting actual past performances, forecasted ones based on projected market trends, or purely statistical representations — may all be employed within this approach to predict how portfolios could fare moving forward.
A principal benefit derived from using MCS is its capacity to replicate diverse scenarios within markets and evaluate subsequent possibilities thereof. Crafting many theoretical futures furnishes a thorough outlook on prospective deviations in investment gains or losses. Such comprehensive understanding proves exceedingly beneficial when refining investment approaches and confirming alignment between portfolio configurations and desired financial objectives.
To summarize, employing the Monte Carlo Simulation offers significant advantages in forecasting investment results by harnessing both historical patterns and probabilistic modeling techniques—a critical practice providing valuable insights toward crafting an ideal asset mix aimed at optimizing yield while curtailing exposure to risk factors.
Efficient Frontier Visualization
The concept of the efficient frontier is fundamental to the practice of optimizing a portfolio, delineating those portfolios that provide the maximum expected return for each increment of risk taken. This visualization empowers investors by allowing them to pinpoint optimal portfolios delivering peak expected returns proportionate to their chosen level of risk, which is crucial for enlightened investment strategy formulation and fine-tuning asset distribution.
Employing either historical return data or projections about future market performance facilitates an authentic perspective on what prospective earnings might look like. The Monte Carlo method is instrumental in this context as it equips investors with a view into a range of possible outcomes rather than fixating on singular projected returns, offering a broader awareness regarding how different levels of risk may interact with potential returns.
By incorporating Monte Carlo simulations (MCS) within this visual framework, Clarity emerges concerning how various portfolios could perform over time. Such deeper insight assists investors in refining their decisions pertaining to allocation while striving toward their financial objectives. Ultimately, through leveraging these tools and concepts such as the efficient frontier itself—a vital aid—investors can discern more precisely those investment mixes that adeptly strike a balance between anticipated reward and associated exposure to risk.
Optimizing Portfolio Weights
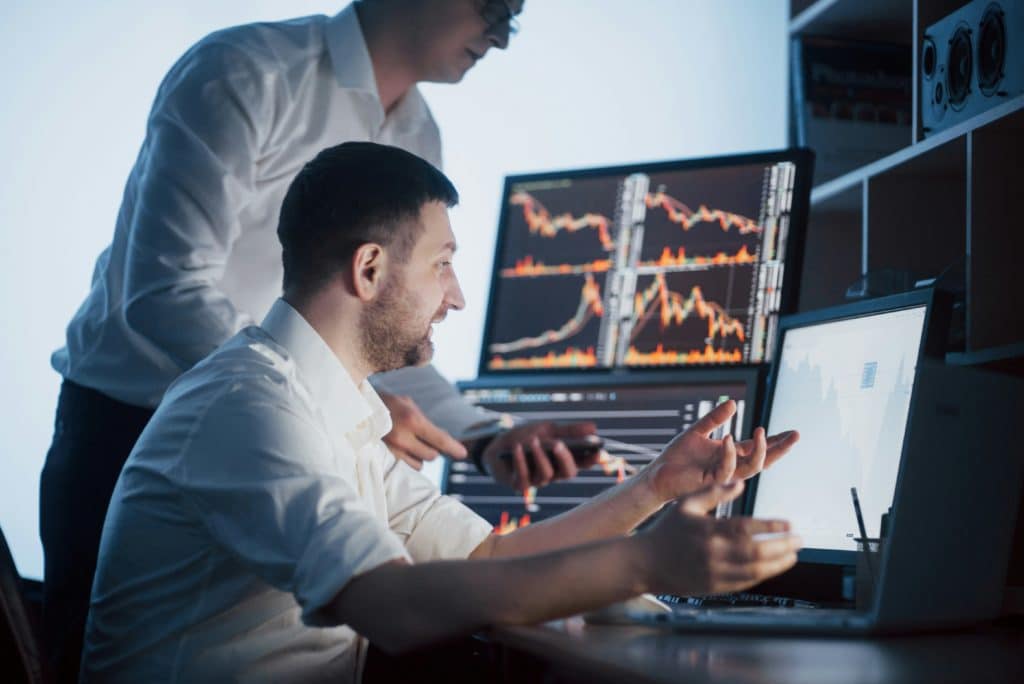
The use of Monte Carlo simulations plays a pivotal role in pinpointing the most favorable portfolio weights to achieve the highest risk-adjusted return. These simulations shed light on both the anticipated returns and risks associated with various securities, thus aiding investors in selecting asset allocations that align with their tolerance for risk and investment objectives. Mean-Variance Optimization is employed as a core strategy to identify these ideal allocations.
For successful portfolio optimization, one must consider not only projected annual returns but also require the covariance matrix detailing how asset returns move together. The Monte Carlo method refines this optimization by tweaking inputs to mitigate estimation inaccuracies and amplify diversification benefits. Consequently, it becomes apparent through this approach that top-performing portfolios often consist of merely a handful of different securities, which leads to an investment approach that is more streamlined and effective.
When optimizing portfolios, utilizing the Sharpe ratio—an important measure quantifying return-to-risk proportion—is vital for maximizing this indicator ensures discovery of portfolios offering superior risk-adjusted earnings crucial data when making strategic investment choices aiming at enhancing overall performance within one’s portfolio.
Ultimately, applying Monte Carlo Simulations (MCS) techniques proves greatly advantageous for those looking to optimize their investments’ distribution effectively harnesses statistical models alongside sophisticated optimization methods serves in identifying an optimal portfolio—one designed explicitly to heighten profits while concurrently reducing exposure, setting up investors down a path of achieving sustained financial triumphs over time.
Analyzing Risk Metrics and Potential Outcomes
Monte Carlo simulation, often referred to as stochastic modeling, serves as a robust mechanism for evaluating the risk associated with investments. Enhanced methods of portfolio optimization can lead to superior management of risks and increased potential for returns by considering the delicate balance between expected risk and return.
It is essential to spread investment across various asset classes in order to diminish risk while boosting the overall value of portfolios. Investors rely on critical risk metrics like Conditional Value at Risk (CVaR) and maximum drawdown to gain an understanding of their portfolio’s susceptibility to losses. These indicators yield significant insights into the possible advantages and dangers that accompany differing investment approaches.
By scrutinizing these metrics alongside potential outcomes from Monte Carlo simulations, investors are equipped with knowledge necessary for making well-informed choices geared towards refining their portfolio strategies over time. This analytical approach is vital in curating a diversified investment plan that both optimizes gains and mitigates exposure to unnecessary risks.
Case Study: Real-World Application
The Monte Carlo simulation serves as a potent instrument for portfolio optimization, providing investors with the capacity to evaluate risk and returns through methods of random sampling. The process of implementing this simulation requires the collection of asset data such as historical price movements and computing average returns while gauging their volatility, often utilizing financial APIs. Through employing random sampling in its methodology, the simulation is adept at producing an array of diverse portfolio combinations which are instrumental in appraising prospective outcomes that pertain to investment approaches.
Visualizing the efficient frontier constitutes an essential phase within this procedure, facilitating investors’ ability to pinpoint ideal asset mixes that deliver maximum Sharpe ratios. Upon executing numerous iterations within the Monte Carlo process, various metrics pertaining to risk—including standard deviation and CVaR—are meticulously scrutinized to steer decisions regarding investments.
Predictions on the long-term value appreciation potential of a well-tuned portfolio are made viable through insights from Monte Carlo simulations over different timeframes. These forecasts encapsulate both possible yields and accompanying risks associated with them. Such applied usage underscores how incorporating MCS into practices concerning portfolio optimization can be greatly advantageous for investors intent on making more informed choices backed by robust quantitative analysis.
Expected Portfolio Value Over Time
After a decade, the anticipated return for an optimal portfolio is projected at 5.51%. The expected range of the ultimate value after this period lies between $103,268 and $267,331. From simulations carried out, the average annualized return on said portfolio stands at 2.0%, with associated risks calculated to be approximately 13.08%.
Different withdrawal approaches can be integrated into these projections, including strategies like constant yearly withdrawals or those based upon a percentage system. Withdrawal rates guided by life expectancy align the amount taken from portfolios in conjunction with estimates of one’s remaining years.
This method significantly betters portfolios that are equally weighted across various securities and offers investors a more strategically sound avenue for managing their investments. Having insight into future values of given investment baskets empowers individuals seeking financial prosperity through smarter decision-making tailored towards fostering ideal investment collections over time.
Summary
Monte Carlo Simulation serves as an essential instrument for refining portfolios, offering investors crucial information to weigh the trade-offs between risk and potential gains. By collecting top-tier data, modeling how a portfolio might perform, showcasing the efficient frontier, and adjusting investment weights accordingly, investors are positioned to fulfill their financial aspirations while aiming for peak returns.
Ultimately, Monte Carlo Simulation translates the intricate concepts of modern portfolio theory into actionable tactics that enhance investment acumen. Investors who embrace and apply MCS can deftly handle market uncertainties on their journey towards enduring wealth creation. The cornerstone of savvy investing is grounded in well-informed choices drawn from exhaustive data analysis and comprehensive simulations.
Frequently Asked Questions
What is Monte Carlo Simulation?
The Monte Carlo Simulation employs a statistical approach that incorporates continuous random sampling to create models of possible investment scenarios for the purpose of evaluating different results.
Investors leverage this technique to facilitate decision-making grounded on an analysis that projects potential future returns.
How does Monte Carlo Simulation help in portfolio optimization?
The Monte Carlo simulation assists in refining the process of portfolio optimization by enabling the examination of various strategies for asset allocation. This helps to predict possible returns and assess associated risks.
Through this form of analysis, it becomes feasible to determine the optimal distribution of assets that balances maximizing returns with reducing risk exposure.
Why is the quality of input data important for Monte Carlo Simulation?
The quality of input data is crucial for Monte Carlo Simulation as it directly affects the accuracy of the simulations and the reliability of the results.
Accurate outcomes are essential for making informed investment decisions.
What is the efficient frontier, and why is it important?
The efficient frontier is critical as it delineates the portfolios that yield the highest expected returns for a specified level of risk, guiding investors in achieving optimal asset allocation and informed investment decisions.
Understanding this concept enables more strategic investment planning.
How does the Sharpe ratio influence portfolio optimization?
The Sharpe ratio significantly influences portfolio optimization by allowing investors to maximize risk-adjusted returns.
This leads to the identification of more efficient investment strategies.