What Is Agentic AI? A Quick Guide
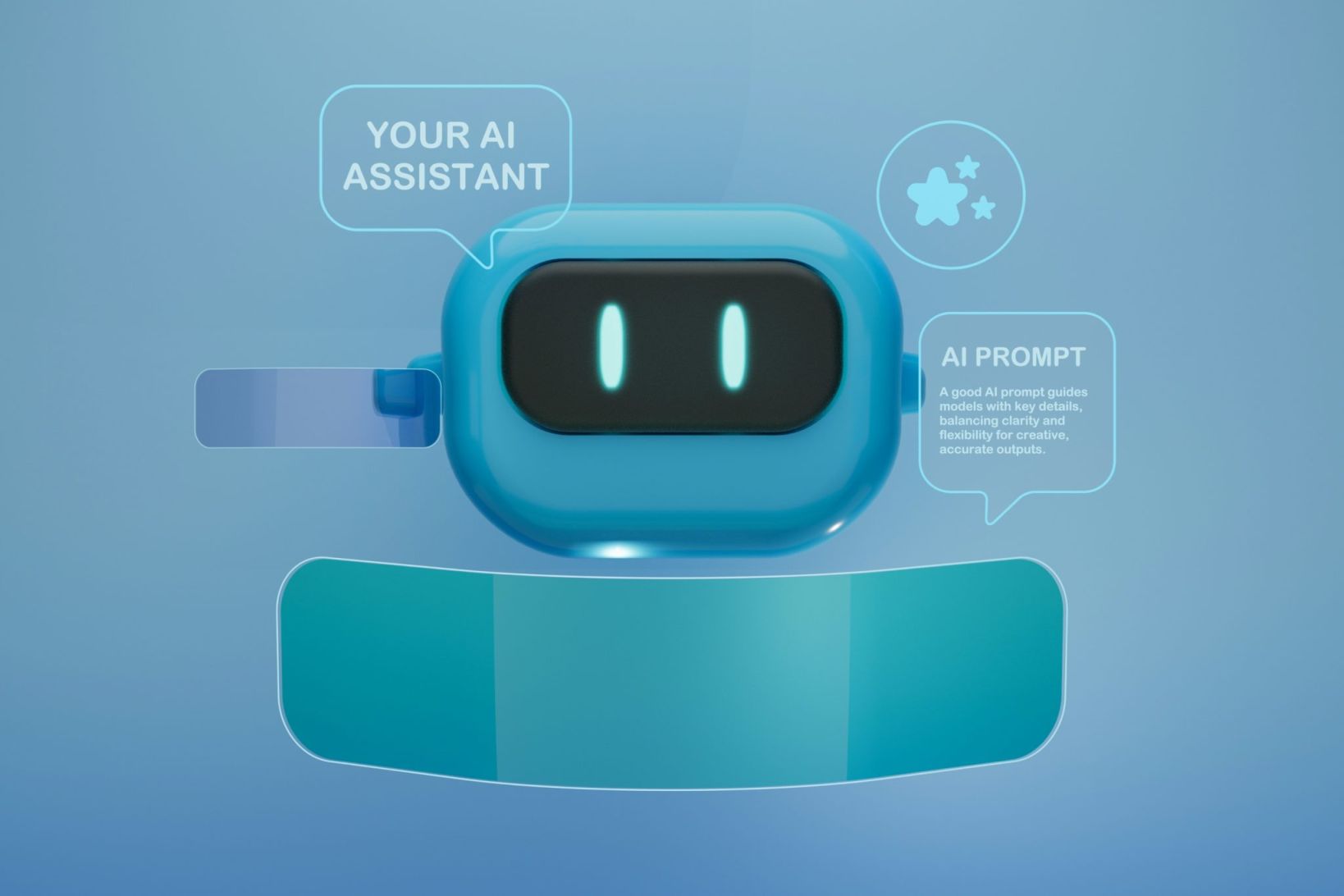
Unlike traditional AI, Agentic AI operates autonomously, making decisions and taking actions without constant human input. This next-generation technology is transforming industries by automating workflows, enhancing customer interactions, and optimizing decision-making. In this article, we’ll explore its fundamentals, benefits, and real-world applications.
Understanding Agentic AI
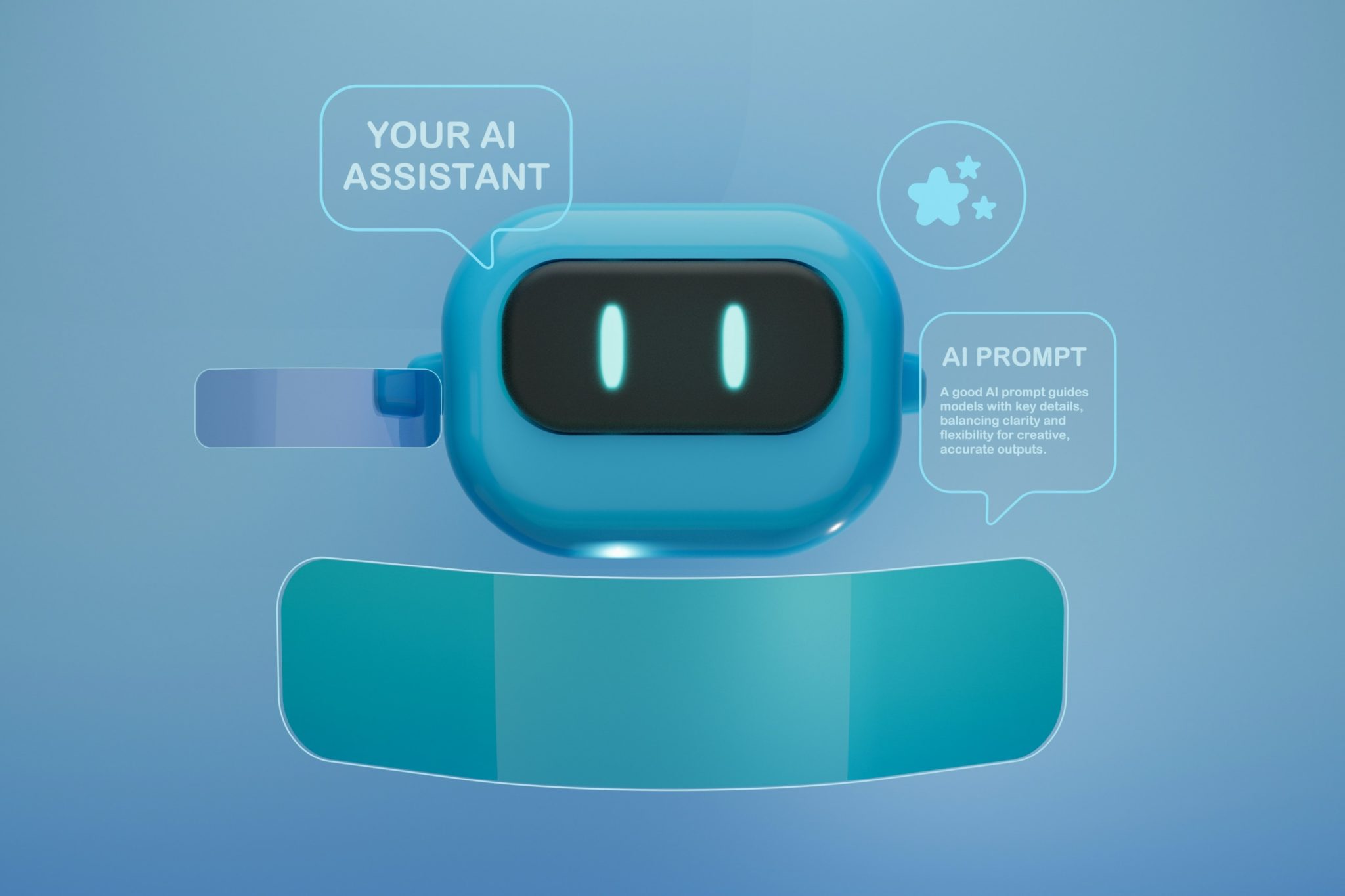
Agentic AI marks a major advancement in artificial intelligence, enabling AI agents to function autonomously with minimal human oversight. These AI systems feature autonomous decision-making, advanced reasoning, and the ability to adapt using real-time data.
Unlike traditional AI, which relies on predefined rules, agentic AI systems make independent decisions based on their operating context. While traditional AI focuses on single-agent tasks, Agentic AI employs multiple agents to autonomously manage complex workflows.
Standout features of Agentic AI include:
Ability to work independently, managing complex tasks with little human help. This AI can learn and adapt from feedback, improving its decision-making over time.
Ability to autonomously manage intricate processes, distinguishing it from traditional AI systems that lack context-driven adaptability. This flexibility is vital for real-time decision-making and continuous learning, positioning Agentic AI as a transformative force in various industries.
For example, in dynamic environments like supply chain management, an agentic AI system can forecast demand, place orders, and handle logistics on its own, cutting down costs and boosting efficiency.
How Agentic AI Operates
Agentic AI utilizes a sophisticated distributed systems platform, facilitating seamless communication and information sharing among multiple AI agents. This collaborative framework allows AI agents to pool their collective intelligence, effectively tackling complex problems that might be challenging for a single AI agent. It’s akin to a team of experts combining their knowledge and skills to devise optimal solutions. Multiple AI agents work in harmony to boost overall performance.
A critical component of how Agentic AI functions is through machine learning, which allows these systems to learn from vast amounts of data, continually improving their performance and identifying patterns that inform their decision-making processes. For example, in customer relationship management, AI agents can analyze customer interactions in real-time, providing personalized responses that enhance the customer experience.
Natural language processing (NLP) is another cornerstone of Agentic AI, enabling these systems to understand, interpret, and generate human language. This capability is instrumental in applications such as virtual assistants, where understanding and responding to human queries accurately is paramount. By analyzing data in real-time, agentic AI systems can identify patterns, optimize strategies, and apply reasoning and iterative planning to solve complex problems effectively.
Core Features of Agentic AI
Autonomy:
Allows AI agents to perform tasks independently.
Enables informed decision-making without constant human intervention.
Beneficial in environments requiring quick decision-making, like financial trading or emergency response.
Adaptability:
Designed to learn from new data sets and environments.
Quickly responds to changing conditions.
Example: In manufacturing, adjusts production schedules in real-time based on demand or supply chain disruptions.
Contextual Understanding:
Enhances decision-making capabilities.
Interprets data and situations based on surrounding information.
Essential in applications like healthcare, improving diagnostic accuracy and treatment plans.
Comparing Agentic AI with Traditional AI
When comparing Agentic AI with traditional AI, several key differences emerge that highlight the advancements of agentic systems.
Feature |
Traditional AI |
Agentic AI |
Operational Approach |
Based on predefined rules and parameters |
Highly autonomous, makes independent decisions |
Adaptability |
Limited flexibility, struggles with changing contexts |
Adapts actions based on real-time data analysis |
Complexity Handling |
Best for well-defined, simpler tasks |
Manages multifaceted, dynamic scenarios independently |
Decision-Making |
Reactive—responds to inputs as they occur |
Proactive—anticipates and adjusts to future needs |
Use Cases |
Automating repetitive tasks in controlled environments |
Handling dynamic environments (e.g., logistics, supply chains) |
Cost |
Lower initial costs, but limited long-term scalability |
Higher setup costs but reduced human oversight and greater efficiency |
Customization |
Fixed capabilities with minimal evolution |
Highly customizable and continuously evolves with technology |
Benefits of Implementing Agentic AI
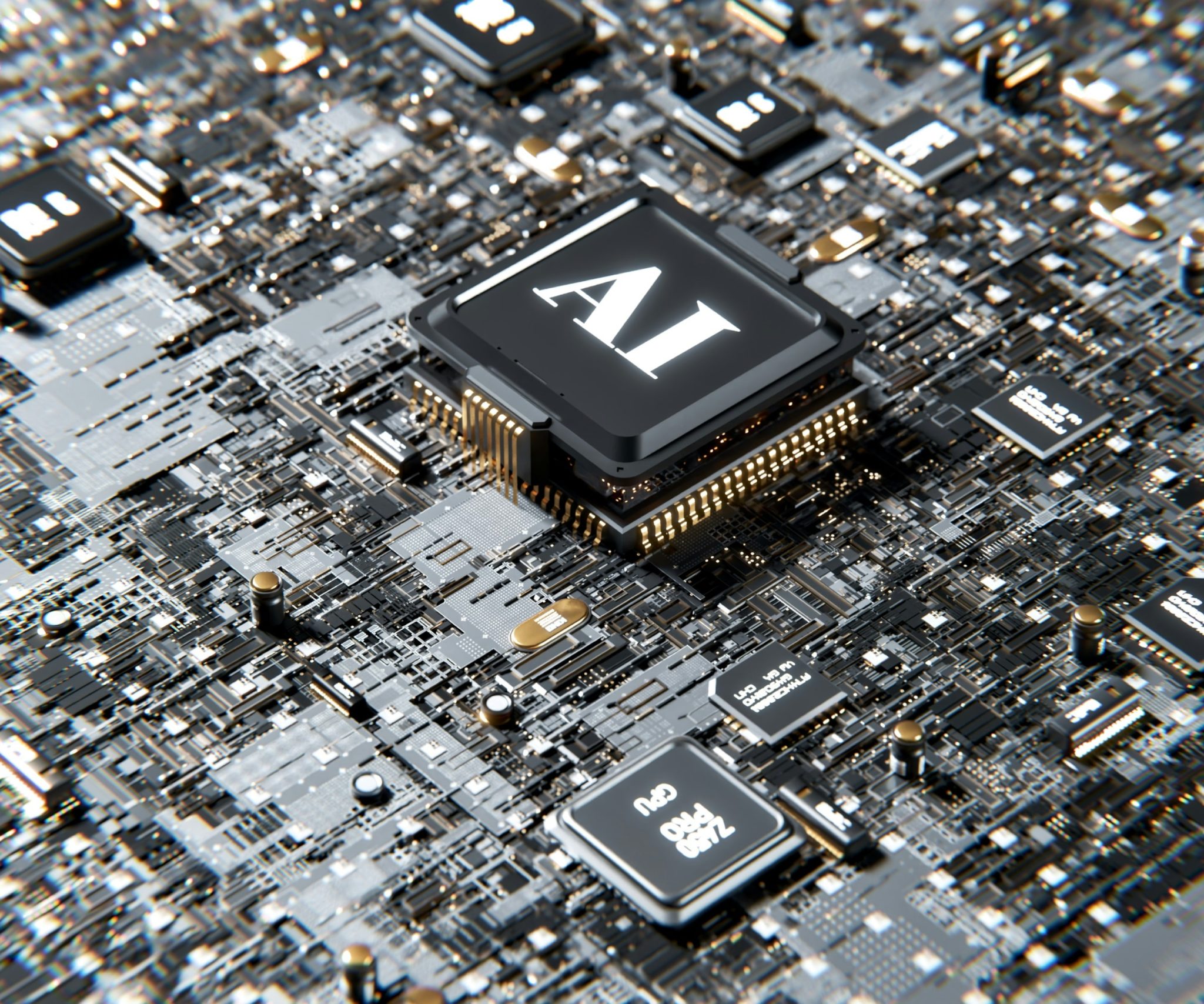
Operational Efficiency and Task Automation
Agentic AI significantly enhances operational efficiency across various sectors.
Automates complex tasks, resulting in faster processing times and reduced error rates.
Example: In the insurance industry, Agentic AI streamlines claims processing by automatically verifying data and flagging discrepancies.
Service Delivery and Resource Management
Improves service delivery and operational effectiveness through autonomous prioritization and resource allocation.
Saves time and reduces operational costs, making businesses more agile and responsive to market demands.
Example: Dynamic adjustments in logistics facilitated by enabling AI agents optimize supply chain management, resulting in cost savings and improved delivery times.
As AI agents operate, they further enhance efficiency and responsiveness.
Data Analytics and Decision-Making
Transforms data analytics by providing immediate, actionable insights instead of relying on static reports.
Real-time data analysis optimizes decision-making processes, allowing businesses to swiftly respond to emerging trends and challenges.
Example: Whether optimizing marketing strategies or improving customer service, Agentic AI’s ability to analyze and act on real-time data is transformative.
Enhanced Adaptability and Efficiency
Agentic AI enhances adaptability and efficiency in various ways. By leveraging advanced machine learning algorithms and natural language processing, agentic AI systems can analyze vast amounts of data, identify patterns, and make informed decisions in real-time. This enables organizations to respond quickly to changing market conditions, customer needs, and other dynamic factors. Additionally, agentic AI can automate repetitive tasks, freeing human teams to focus on strategic, high-value work. This not only improves productivity but also reduces the risk of human error, leading to increased efficiency and accuracy.
For instance, in the retail sector, agentic AI systems can analyze customer purchasing behavior and adjust inventory levels accordingly, ensuring that popular products are always in stock. In the financial industry, these systems can monitor market trends and execute trades autonomously, optimizing investment strategies and maximizing returns. By continuously learning and adapting, agentic AI systems help organizations stay agile and competitive in an ever-evolving landscape.
Real-World Applications of Agentic AI
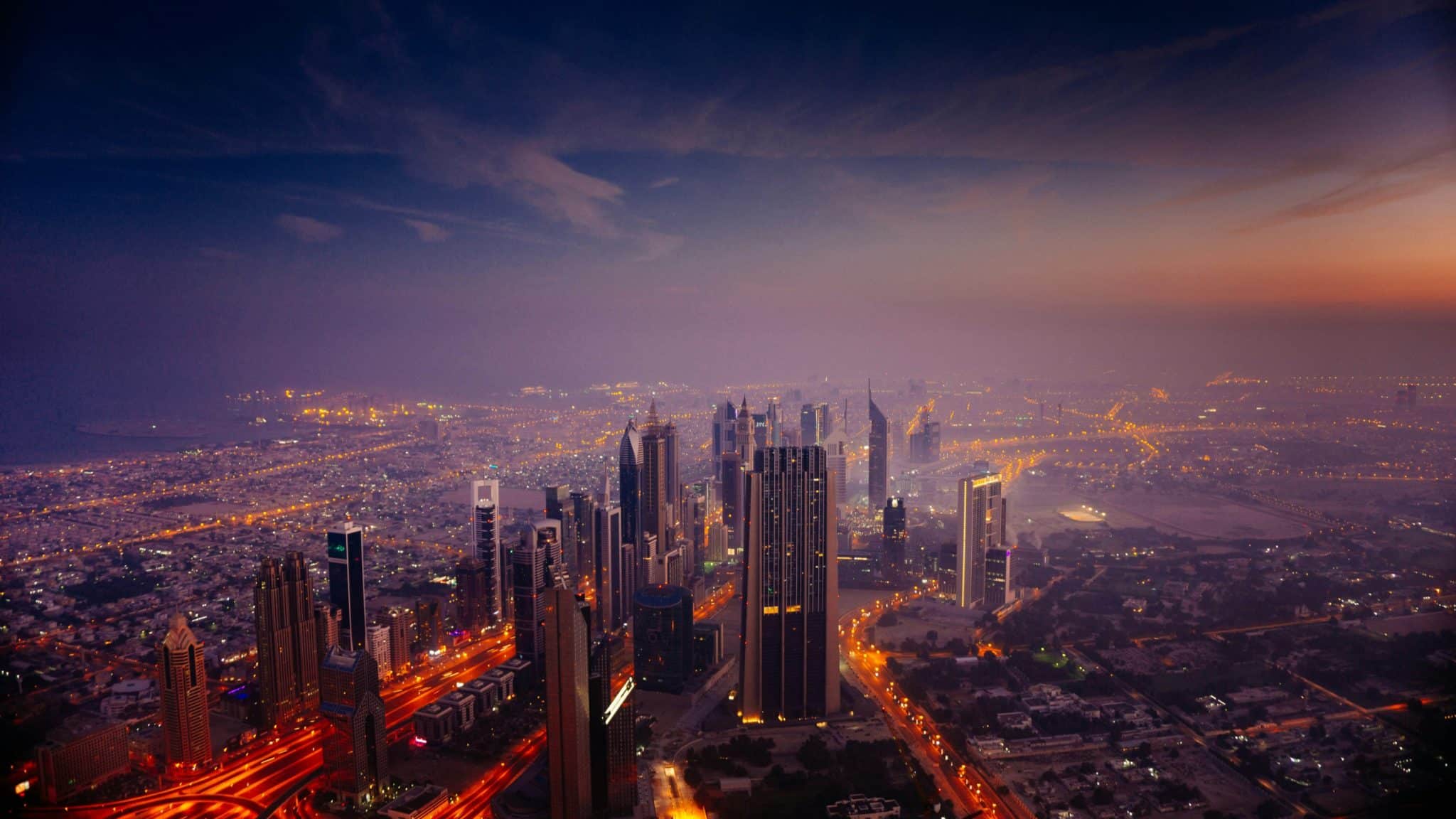
Agentic AI’s real-world applications span various industries, driving workflow automation and enhancing decision-making processes. From supply chain management to customer relationship management and incident response, the versatility of Agentic AI makes it an invaluable tool for modern enterprises.
In the following subsections, we will delve into specific applications to illustrate how Agentic AI is transforming these areas.
Enhancing Supply Chain Management
In the realm of supply chain management, Agentic AI proves to be exceptionally effective. By continuously analyzing and responding to disruptions, these AI systems can autonomously manage supply chains, ensuring smooth operations even in the face of unforeseen challenges. For instance, Agentic AI can predict demand fluctuations and automatically place orders, significantly reducing operational costs and improving efficiency.
Moreover, by leveraging real-time data, Agentic AI can optimize logistics and inventory management, reducing waste and enhancing overall supply chain performance. This capability is particularly beneficial in industries with complex supply chains, where timely and accurate decision-making is crucial for maintaining competitive advantage.
Improving Customer Relationship Management
Agentic AI also plays a pivotal role in improving customer relationship management (CRM). By analyzing vast amounts of customer data, AI systems can deliver personalized and timely responses, enhancing the overall customer experience. For instance, Agentic AI chatbots can provide tailored interactions based on individual customer preferences and past behaviors.
Additionally, Agentic AI enables companies to predict and address customer needs proactively, leading to higher customer satisfaction and loyalty. By integrating with existing enterprise systems, these AI solutions can streamline customer interactions and improve operational efficiency, making CRM more effective and responsive to customer demands.
Automating Incident Response
Automating incident response is another critical application of Agentic AI. By automating various incident response protocols, these systems significantly enhance the efficiency and effectiveness of managing incidents. For example, companies like Leidos have achieved a 99% reduction in mean time to resolution for incidents by leveraging Agentic AI.
Agentic AI can automate tasks such as triggering response protocols, notifying team members, initiating rollback procedures, and generating incident reports, reducing the need for constant human intervention. This automation allows human employees to focus on strategic initiatives, thereby improving overall operational efficiency and reducing the impact of incidents on business operations.
Key Technologies Enabling the AI Tools
Several key technologies enable the advanced capabilities of Agentic AI. One of the most influential is the use of large language models (LLMs) like GPT-3 and GPT-4, which allow AI systems to comprehend and generate human-like text. These generative ai models support natural conversations and decision-making, making them essential for applications such as virtual assistants and customer service chatbots.
Advancements in machine learning algorithms and increased computational power have also played a crucial role in the development of Agentic AI. Machine learning enables these systems to learn from data and improve over time, enhancing their adaptability and decision-making capabilities. For example, deep learning models can analyze complex data sets to identify patterns and make predictions, supporting more accurate and effective AI solutions.
Cloud computing and edge computing further enhance the capabilities of Agentic AI by providing scalable computing power and storage capacity. Cloud computing supports the scalability and security of AI agentic workflows, allowing businesses to handle large volumes of data and complex processes efficiently. Meanwhile, edge computing facilitates faster decision-making by processing data closer to where it is generated, enabling real-time responses and reducing latency.
Challenges and Ethical Considerations
Despite the numerous benefits, implementing Agentic AI also presents several complex challenges and ethical considerations. Establishing robust governance frameworks is crucial for defining accountability and ensuring ethical AI practices are followed. Institutions must prioritize ethical AI to maintain public trust and comply with regulatory standards.
One of the primary ethical concerns is algorithmic bias, which can lead to certain demographic groups being unfairly flagged as high-risk. Employing diverse datasets and fairness-aware algorithms is essential to mitigate these risks and ensure equitable AI implementations. Continuous monitoring of agentic AI systems is necessary to identify biases and ensure ethical compliance throughout their lifecycle.
Moreover, the lack of transparency in Agentic AI systems complicates compliance efforts, especially in justifying flagged transactions. Sensitive data processed by these systems increases the risk of data breaches, necessitating strict compliance with data protection laws. Addressing these challenges requires a concerted effort to balance innovation with ethical considerations, ensuring that the deployment of Agentic AI aligns with societal values and norms.
Autonomy and Decision-Making Capabilities
Those AI systems’ autonomy and decision-making capabilities are key differentiators from traditional AI systems. By combining machine learning, natural language processing, and knowledge representation, agentic AI systems can reason, perceive their environment, and take autonomous action. This enables them to make decisions without human oversight, adapt to new situations, and optimize their actions to achieve specific goals. Furthermore, agentic AI systems can learn from their experiences and improve their performance over time, leading to increased autonomy and decision-making capabilities.
For example, in the healthcare sector, agentic AI systems can analyze patient data, diagnose conditions, and recommend treatment plans without the need for constant human intervention. In the transportation industry, autonomous vehicles equipped with agentic AI can navigate complex traffic scenarios, make real-time route adjustments, and ensure passenger safety. By operating independently and making informed decisions, agentic AI systems enhance efficiency and effectiveness across various domains.
The Future of Agentic AI
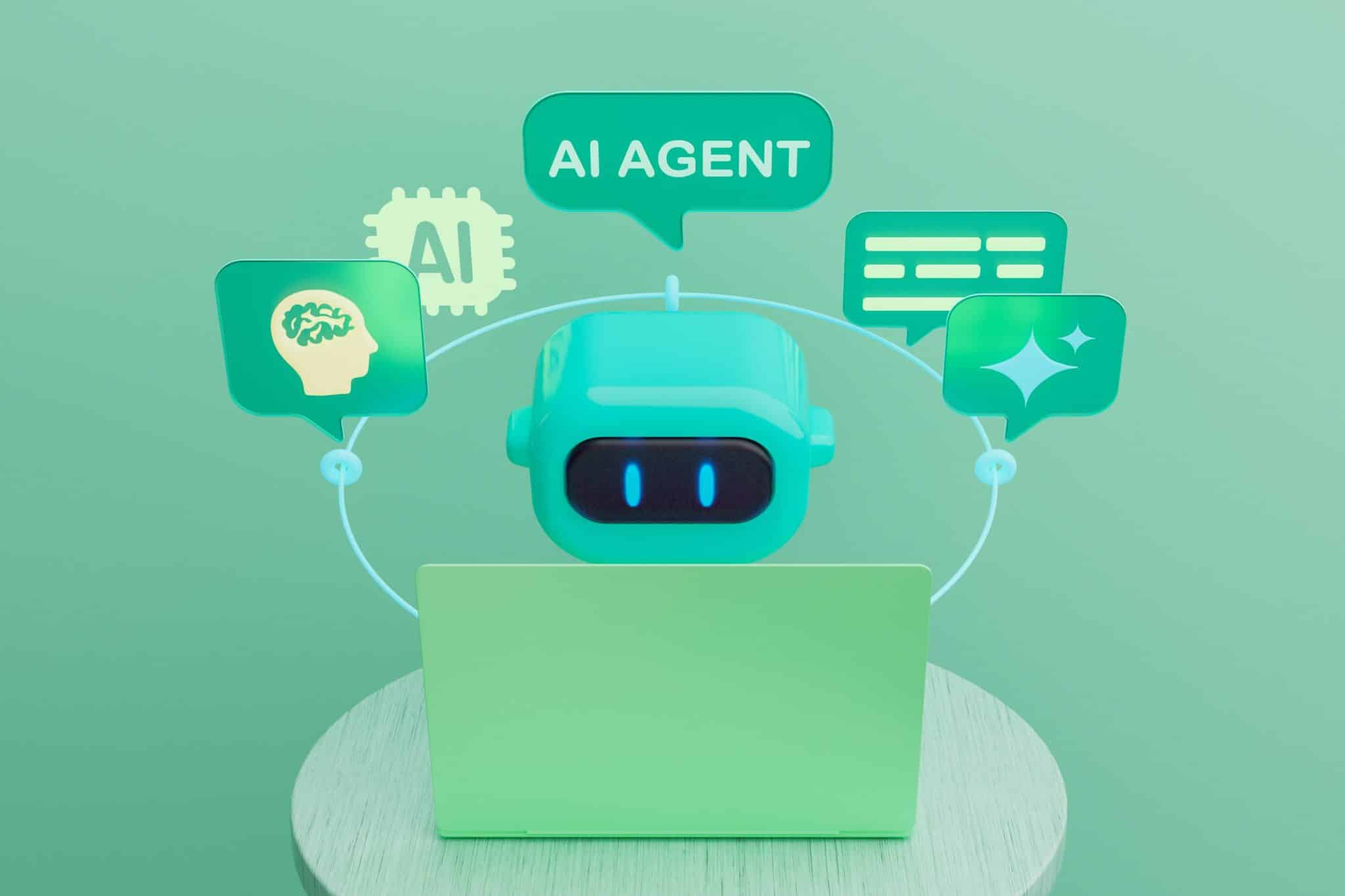
The future of Agentic AI promises to be transformative, with advancements expected to integrate capabilities like cross-domain learning and real-time collaboration. As Agentic AI systems continue to learn and improve through data and experience, their applications will expand, impacting sectors such as healthcare, finance, and manufacturing. For instance, in healthcare, Agentic AI can enhance diagnostic systems through collaboration among specialized agents, leading to more accurate and timely diagnoses.
However, the evolution of Agentic AI may also introduce new economic and social dynamics, potentially leading to job displacement in certain fields. Collaboration between human workers and Agentic AI systems will be crucial to boost overall productivity and engagement, ensuring that the workforce adapts to these changes.
Gartner forecasts that the integration of Agentic AI into enterprise software applications will rise from less than 1% in 2024 to 33% by 2028, enabling this significant shift in autonomous decision-making. As we look ahead, the continuous advancement and adoption of Agentic AI will redefine the landscape of technology and human interaction, paving the way for a future where intelligent systems work seamlessly alongside humans to solve complex problems and drive innovation.
Quickly Building Agentic Workflows
Building Agentic AI workflows quickly and effectively requires a strategic approach that integrates these systems with existing enterprise systems. Tailoring the implementation to specific organizational needs is crucial for ensuring that Agentic AI interacts effectively with software and fulfills its intended roles. For example, in dynamic environments like logistics, AI agents must be able to switch between different roles based on task requirements, allowing for flexible and adaptive workflows.
Successful implementation often involves collaboration across various departments, particularly IT, to ensure that the systems are integrated smoothly. Pilot projects are essential for testing the viability and effectiveness of these workflows to execute tasks before scaling them up to full deployment. This phased approach helps identify potential issues and refine the system to better meet organizational goals.
Moreover, the integration of Agentic AI with multiple platforms and data sources allows businesses to automate complex workflows that involve multi-step processes. By automating repetitive tasks and interpreting complex instructions, Agentic AI can significantly enhance operational efficiency and free up human resources for more strategic initiatives.
Implementation and Integration
Implementing and integrating agentic AI systems requires careful planning and consideration. Organizations must first identify the specific tasks and workflows they want to automate and then determine the most suitable agentic AI solution. This involves evaluating the capabilities of different agentic AI systems, assessing their compatibility with existing enterprise systems, and ensuring they meet the organization’s security and compliance requirements.
A successful implementation strategy often begins with pilot projects to test the feasibility and effectiveness of the agentic AI system in a controlled environment. These pilot projects help identify potential challenges and allow for adjustments before full-scale deployment. Collaboration between IT, operations, and other relevant departments is crucial to ensure a smooth integration process.
Integration with Enterprise Systems
Integrating agentic AI systems with enterprise systems is vital for maximizing their capabilities. By linking these AI systems to existing data sources, applications, and infrastructure, organizations can create a cohesive operational framework. This setup allows smooth collaboration between humans and AI agents, ensuring AI systems have the necessary data to make informed decisions and act autonomously. Integration also aligns AI systems with company goals and procedures, reducing errors and enhancing efficiency.
For instance, in manufacturing, connecting agentic AI with enterprise resource planning (ERP) systems can streamline production, optimize resource use, and minimize downtime. In customer service, integrating AI chatbots with customer relationship management (CRM) systems enables personalized and efficient interactions. By ensuring seamless integration with enterprise systems, businesses can fully leverage agentic AI’s benefits, driving performance and productivity improvements.
How does InvestGlass leverages AI in CRM?
InvestGlass is at the forefront of revolutionizing customer relationship management (CRM) by integrating advanced AI capabilities into its platform. By harnessing the power of agentic AI, InvestGlass is able to provide a more personalized, efficient, and responsive CRM experience. This innovative service uses AI agents to analyze vast amounts of customer data in real-time, enabling businesses to tailor interactions and anticipate customer needs with precision.
With machine learning and natural language processing, InvestGlass enhances customer interactions, seamlessly integrates with enterprise systems, and boosts operational efficiency. The result? Reduced costs, improved service, and stronger customer loyalty—making InvestGlass an essential tool for modern businesses.
Ready to harness the power of AI-driven automation? Discover how InvestGlass can revolutionize your operations today.
FAQs
What is Agentic AI?
Agentic AI means artificial intelligence systems that can operate without human supervision, making autonomous decisions based on real-time data and learning from experiences to improve their decision making.
How is Agentic AI different from traditional AI?
Agentic AI is different from traditional AI by being autonomous and making independent decisions whereas traditional AI depends on pre-defined rules and parameters. This allows Agentic AI to navigate complex scenarios based on context needs.
What are some real-world use cases of Agentic AI?
Agentic AI is used to enhance supply chain management by predicting demand, personalize customer relationship management and automate incident response to boost operational efficiency. These use cases show its potential to improve business processes. Agentic AI is driven by technologies like large language models (GPT-3 and GPT-4), machine learning and cloud and edge computing for scalability and real-time decision making.
What are the concerns around Agentic AI?
Concerns around Agentic AI are: strong governance, algorithmic bias, transparency and data protection.