Mastering How to Calculate Correlation Coefficient: A Step-by-Step Guide
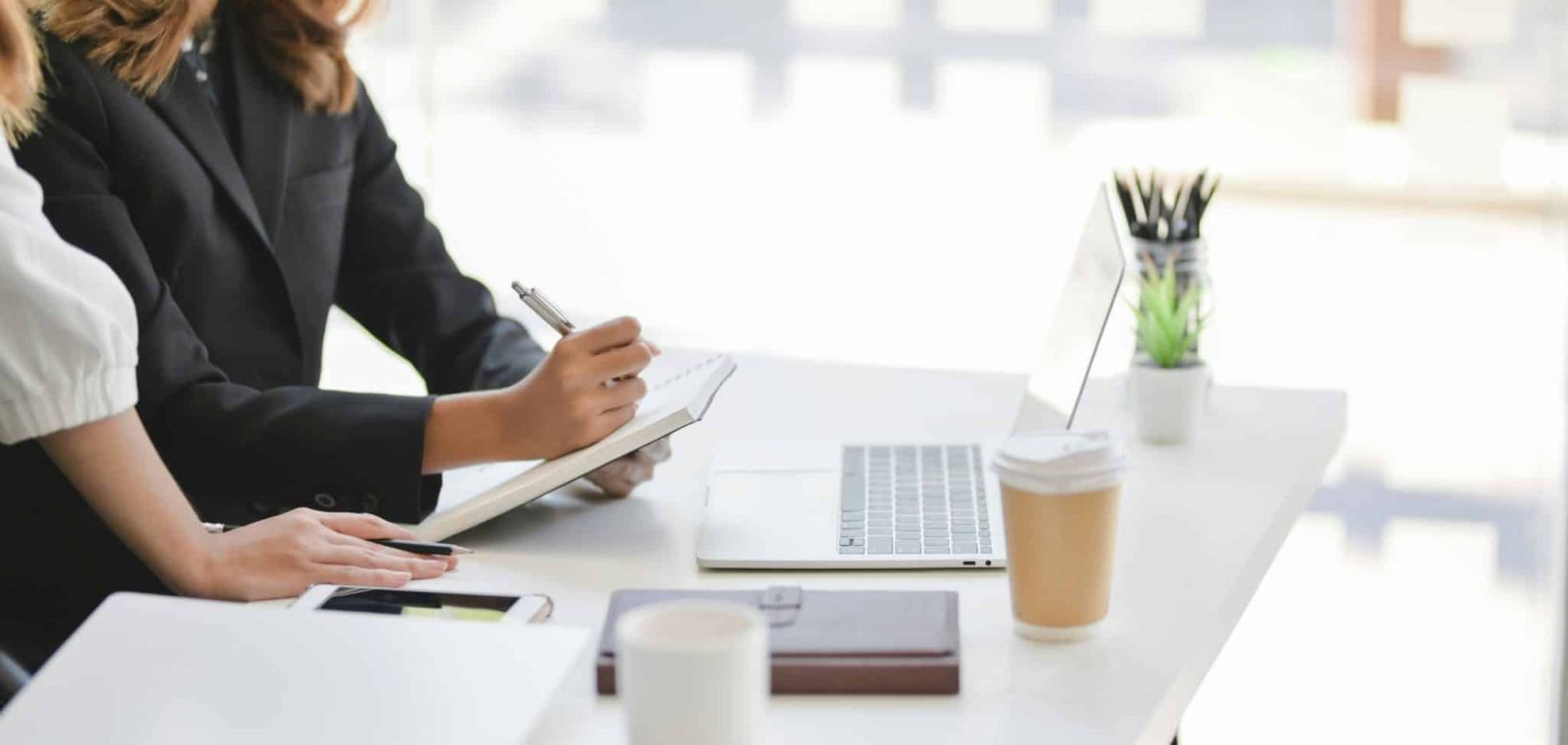
Need to understand how to calculate correlation coefficient? This guide covers the entire process step-by-step, ensuring you can accurately determine how to calculate the correlation coefficient and the relationship between two variables. Let’s dive into the details.
Key Takeaways
The correlation coefficient quantifies the strength and direction of a linear relationship between two variables, with values ranging from -1 to 1.
Calculating the correlation coefficient involves several steps, including data organization, mean and standard deviation calculation, z score computation, and summing the products of z scores.
Interpreting the correlation coefficient requires understanding its proximity to 1 or -1 for strong correlations and recognizing its limitations regarding causation and non-linear relationships.
Understanding the Correlation Coefficient
The correlation coefficient serves as a statistical index to assess the strength and directionality of the linear association between two distinct variables. It encapsulates the degree to which two variables are connected within a linear framework. Pearson’s correlation coefficient is a statistical metric that quantifies and describes the strength of the linear relationship between two variables. This specific measure spans from -1 to 1, with values approaching either extreme indicating more pronounced correlations, while those near zero point toward weaker associations.
When there’s a positive correlation at play, this implies that an uptick in one variable typically leads to an increase in another variable too. In contrast, there is a negative correlation where an increase in one variable will often result in a decrease of another variable. For example, if examining height and weight data points might reveal that taller individuals display higher weights indicative of positive correlation. Meanwhile, observing exercise quantity and bodyweight could manifest an inverse relationship whereby increased exercise correlates with lower body weight — clearly reflected by numerical representation provided by the correlation coefficient deemed crucial for thorough data analysis.
Utilizing visual representations such as scatter plots can shine light on how robustly two variables are interconnected based on their linearity. By scattering individual data points across a graphed space, we can perceive trending directions or discernible patterns echoing what our calculated Pearson correlation quantifies numerically—this graphical interpretation greatly aids simplifying comprehension of directional strengths denoted through our chosen metric’s numeral value.
Step-by-Step Guide to Calculating the Correlation Coefficient
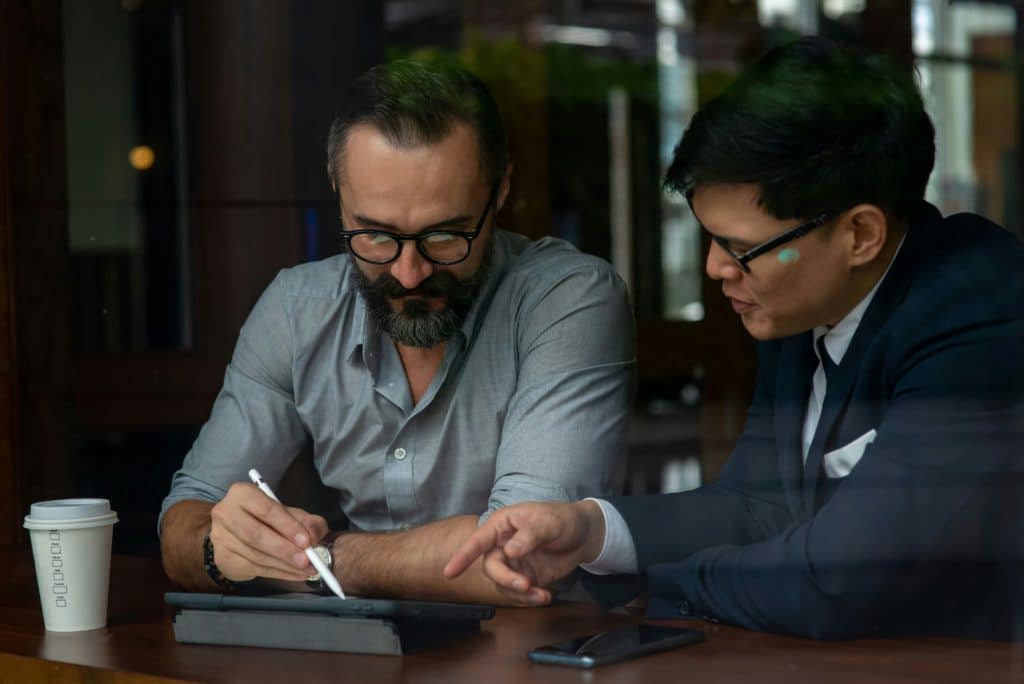
The process of calculating the correlation coefficient consists of a sequence of precise steps designed to determine the degree of correlation between your data sets. These meticulous procedures are crucial in ensuring that the resulting calculation accurately represents how your data is related.
To simplify this task, we will dissect it into understandable segments, leading you from selecting your data sets all the way through to executing the final computation needed to ascertain the correlation coefficient. Let’s get started.
Identify Your Data Sets
To commence the computation of the correlation coefficient, your initial task is to sift and arrange your data. This requires sorting out the numbers into x and y variables, which symbolize the pair of quantitative variables you intend to scrutinize. Take for instance, when examining how study time relates to test scores: ‘hours studied’ serves as your x variable while ‘test scores’ would be classified as your y variable.
Ensure that your collected information satisfies certain standards for conducting a correlation analysis. It’s essential that you have corresponding sets of complete and pertinent data points relative to the subjects being examined. Incomplete or faulty values can skew outcomes, culminating in unreliable interpretations.
Once you’ve structured and affirmed that these data collections are appropriate, they lay down a solid basis from which an accurate determination of the relationship between variables—the correlation coefficient—can be derived. Understanding the relationship between these variables can be quantified using the sample correlation coefficient, which is calculated using a specific formula.
Calculate the Means of X and Y Variables
After arranging your data sets properly, proceed to determine the average values for both x and y variables. This is an essential phase because these averages will act as foundational benchmarks for subsequent computations. To compute the mean of the x variables, add up all the individual x values and then divide this sum by the total count of x values you have. The same methodology applies when calculating the mean for y variables.
Calculating these means is vital to normalize all data points, which is a key aspect in precisely evaluating how x and y variables are related to each other. These calculated averages establish a baseline that facilitates comparison among various data points, thereby laying the groundwork necessary for advanced calculations that follow.
Determine the Standard Deviations
Proceeding to the subsequent phase involves calculating the standard deviations for both x and y variables. The standard deviation is an indicator that quantifies the extent of variation or scatter within a data set. To calculate it, one must assess how far each piece of data strays from the average value, then compute the mean of these individual variances.
This step of normalization is essential as a precursor to computing z-scores in future analyses.
Compute the Z Scores
Having determined the means and standard deviations, you can now calculate the z scores for each individual data point. A z score will tell you how far a particular data point lies from its mean in terms of standard deviations. For x variables, to find the z score (z(x))(i), use the formula: (x(i) – mean of x) / standard deviation of x, where ‘x(i)’ represents an individual value within your data set.
This same method should be employed for y variables using their respective formula that aligns with that used for x variables. By converting both sets into standardized units or z scores, it allows one to evaluate all included data points and y values on an equal scale irrespective of their original scales or units. This normalization is crucial when drawing comparisons between varied datasets as well as understanding interactions among different variables within your study.
Multiply and Sum the Products
During this phase, you will take the z scores of the respective x and y variables and perform multiplication on them. This action is instrumental in gauging how strongly the two variables are connected to each other. Following their multiplication, you should tally up these multiplied results. The resulting total plays a crucial role in computing the final value for the correlation coefficient.
This calculated sum of products becomes a pivotal figure within the formula for determining the correlation coefficient, reflecting to what extent both variables correspond with one another. Through combining standardized values into one collective figure, it establishes a basis from which we can ascertain precisely what level of correlation exists between our set of variables.
Final Calculation
To ascertain the correlation coefficient, which is commonly symbolized as ‘r,’ the culmination of the computational process involves dividing the sum total of product sums by one less than the total count of data pairs. This value offers a quantitative assessment regarding both magnitude and orientation pertaining to any linear relationship that might exist between two distinct variables.
Adhering strictly to these procedural steps ensures precise determination of this statistical metric, thereby shedding light on how closely intertwined your sets of data are in terms of their underlying relational dynamics.
Example Calculation of Correlation Coefficient
To demonstrate the calculation process, let’s examine a set of data concerning newborns’ weights and lengths. Imagine we have this collection of weight values in kilograms: 3.4, 3.6, 3.8, 4.2, and 4.5. Along with these length measurements in centimeters: 50.1, 51.2, 52.3, 53.54, and. Taking the total weight (19kg) and total length (.262 cm), then dividing by our count of data points — which is five — yields average measures for each dataset at kg for weight and cm.
We proceed to determine the standard deviations for both datasets with hypothesized approximations being kg for the weights and cm for the weights.
For lengths. With these figures on hand we go on to compute z scores corresponding to individual observations within our sets Afterward convert standard values by multiplying related pairs from both sets their products are summed up Subsequently dividing accumulated product-of-z scores sum by one less than quantity comprises gives us correlation coefficients.
In relation example presented previously discovered r equates roughly This number suggests there exists discernible strength degree association between infants’ body mass amount measured aligned proportionally indicates tandem increment events.
Visualizing Data with Scatter Plots
Utilizing scatter plots for visual representation of data offers a robust insight into the connection between two quantitative variables. This method of plotting each individual piece of data as dots can uncover trends, correlations, and anomalies that might be obscured when examining raw numbers alone. On such a graph, the x-axis serves to represent one variable while the y-axis denotes another.
Beforehand:
A scatter plot has the capability to reveal if there’s a positive correlation, negative correlation or an absence thereof among variables being examined. For example, observing a clear upward trajectory on such a plot suggests there is indeed a positive correlation. Conversely, spotting downward movement implies a negative correlation exists. The lack of any recognizable trend could indicate no apparent relationship at all.
Subsequently:
The presence or absence of relationships amongst variables can become evident through scatter plots.
A distinct upward progression points to positive correlation.
An obvious downward slope signifies negative correlation.
No visible pattern indicates an absence of correlational ties.
Employing what is known as ‘a line best fit’ may serve to even illuminate how strong this connection between factors actually is by providing us with tangible lines which depict these interactions visually.
Interpreting the Correlation Coefficient
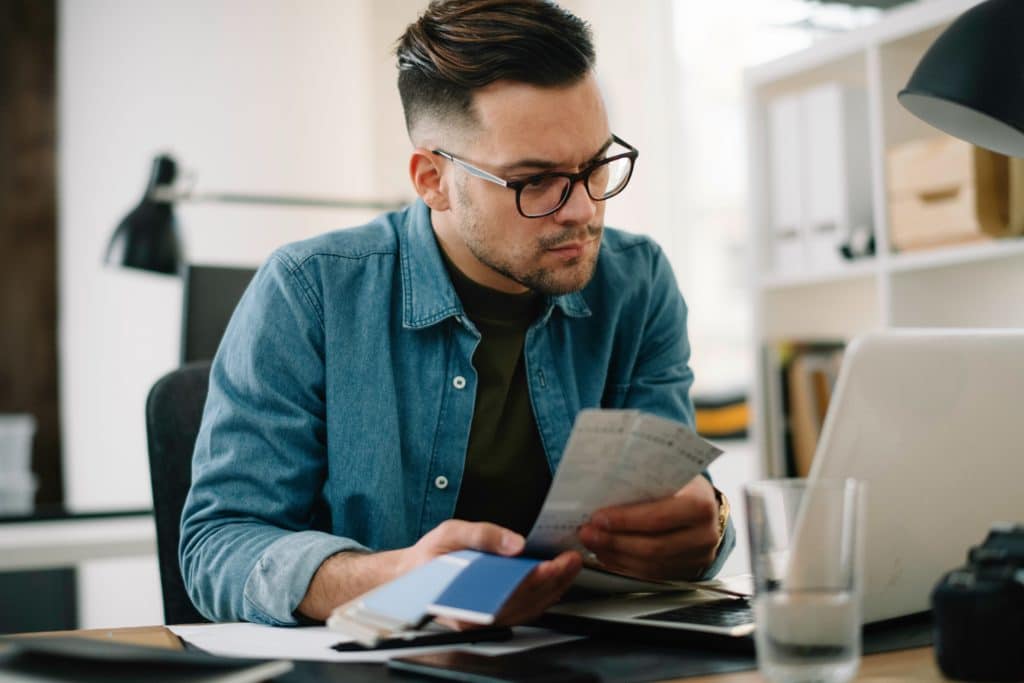
Grasping the significance of the correlation coefficient is essential when analyzing how two variables are related. When a correlation coefficient approaches 1, it signifies that there exists a strong positive linear relationship where an increase in one variable coincides with an increase in another. For instance, a correlation coefficient of 0.85 indicates such a robust positive association between the pair of variables being examined. In contrast, should the coefficient approach -1, this implies a stark negative correlation whereby as one variable goes up, the other tends to go down.
In situations where the correlation coefficient hovers around zero—like values at -0.05 or 0.05—the implication is that there’s either no or only negligible linear relation between these entities. Comprehending these numerical boundaries assists us in intuitively making sense out of our data and deducing significant insights concerning how correlated our variables might be.
It’s important to bear in mind that the scope of what can be understood through this metric extends solely to linear correlations which means even if data may possess apparent patterns suggesting some form of connection doesn’t necessarily translate into them falling neatly along a straight line on visualization graphs or charts—interpreting results judiciously with attention paid to broader contextual information surrounding your dataset is always crucial before jumping to any definitive conclusions about relationships amongst those different sets—or individual points—of values collected from various sources during research activities (or similar scientific explorations).
Pearson vs. Spearman Correlation Coefficients
The Pearson correlation coefficient is a measure designed to evaluate the linear association between two continuous variables. It operates most effectively when the data involved is normally distributed and displays a linear trend. Its sensitivity to outliers must be noted, as even a single outlier can substantially alter the value of the Pearson correlation coefficient, potentially leading to conclusions that are not accurate.
On the other hand, Spearman’s rank correlation coefficient addresses monotonic relationships by utilizing ranked values rather than actual numerical data. Due to this approach, Spearman’s method exhibits greater resilience against outliers and distributions that deviate from normality. It proves particularly advantageous for analyzing ordinal data or scenarios where variables exhibit a uniform pattern but do not maintain an exact linear relationship.
It is crucial when presenting findings on correlations to clearly indicate which type of correlation analysis was employed. By doing so, you ensure precision in communication and assist others in comprehending both context and limitations inherent within your reported outcomes.
Testing Significance of the Correlation Coefficient
To assess whether the correlation observed in data reflects a true relationship or is merely coincidental, one examines the statistical significance of the correlation coefficient. This evaluation typically employs hypothesis testing and hinges on calculating a p-value. The null hypothesis proposes no association between variables while the alternative suggests there is a meaningful correlation.
The significance level set—often 0.05—is used as a threshold for the calculated p-value to determine if we can dismiss the null hypothesis. Therefore, embracing that there’s significant correlation if it falls below this threshold. A specific instance where p-value registers at 0.03 would imply less than a 3% probability that random chance caused such an apparent link, thereby marking statistical importance.
In contrast, using critical values demands comparing sample-derived coefficients with predetermined tabular figures based upon t-distribution tailored by degrees of freedom deduced from subtracting two from total sample size counts. Expressly communicating these findings through both reported p-values and pronounced indications of statistically relevant correlations equips researchers with robust grounds to accurately interpret their analyses outcomes.
Reporting the Correlation Coefficient
For coherence and uniformity, employ a regulated structure while disclosing the correlation coefficient. According to the APA. Style guidelines, one should present the r value along with the degrees of freedom (n-2) followed by the p value. An illustration of how results might be communicated is as follows: r(28) = 0.47, p 0.05.
Ensuring precise disclosure is vital for the clear comprehension and correct interpretation of findings by peers. Such openness in quantitative research is imperative for allowing others to reproduce studies and validate outcomes effectively.
Common Mistakes in Correlation Analysis
A frequent error in correlation analysis is the false belief that a correlation denotes causality. The presence of a correlation between two variables doesn’t automatically imply that one causes the other to change. Take, for instance, discovering a connection between ice cream sales and occurrences of drowning. This does not indicate eating more ice cream leads to drowning risks. It’s possible another factor like increased temperatures could be affecting both factors.
It’s also crucial not to overlook outliers within your data set as they can greatly skew the value of the correlation coefficient and lead you to incorrect assumptions about how two variables relate. To prevent such distortion in assessing their relationship accurately, it’s imperative to detect and properly handle these exceptions before conducting any calculations involving correlations.
Finally, refrain from applying correlation analysis when dealing with data where relationships aren’t linear since the purpose of using a correlation coefficient is primarily for measuring linear connections between variables. For example, considering income relative to age might display non-linear characteristics. Employing a simple calculation method designed for straight-line associations may thus yield results which don’t truly represent their interconnectedness.
Summary
Grasping the methodology for calculating and interpreting the correlation coefficient is crucial when analyzing data. Adhering to a specific process, starting with choosing your data sets and culminating in the calculation, allows you to precisely evaluate both how strong and in what direction two variables relate to each other. It’s important that you consider carefully any possible errors or misinterpretations during analysis to ensure precise conclusions are drawn. With this understanding, informed decision-making becomes more robust due to enhanced insights from your research and analyses of the relationship between various variables within your data.
Frequently Asked Questions
What is the correlation coefficient?** **?
The correlation coefficient quantifies the strength and direction of a linear relationship between two variables, ranging from -1 to 1; values closer to either extreme indicate stronger correlations.
How do you calculate the correlation coefficient?** **?
To calculate the correlation coefficient, first identify your data sets and find the means and standard deviations of the variables.
Then compute the z-scores, multiply and sum the products, and finalize the calculation to obtain the correlation coefficient.
What is the difference between Pearson and Spearman correlation coefficients?** **?
The primary difference between Pearson and Spearman correlation coefficients lies in their applications: Pearson measures linear relationships for continuous data, while Spearman assesses monotonic relationships through data ranking, which offers greater robustness against outliers and is suitable for ordinal data.
Why is it important to test the significance of a correlation coefficient?** **?
It is important to test the significance of a correlation coefficient to ascertain whether the observed relationship is statistically significant or merely a product of random variation.
This evaluation typically employs p-values in hypothesis testing to draw reliable conclusions.
What are common mistakes to avoid in correlation analysis?** **?
It is essential to not make the mistake of inferring causation from correlation, dismissing outliers, or utilizing correlation analysis on nonlinear relationships since these errors can result in faulty conclusions.
A comprehensive grasp of these common pitfalls is vital for conducting dependable correlation analysis.