What are the Challenges of AI Adoption in Banking

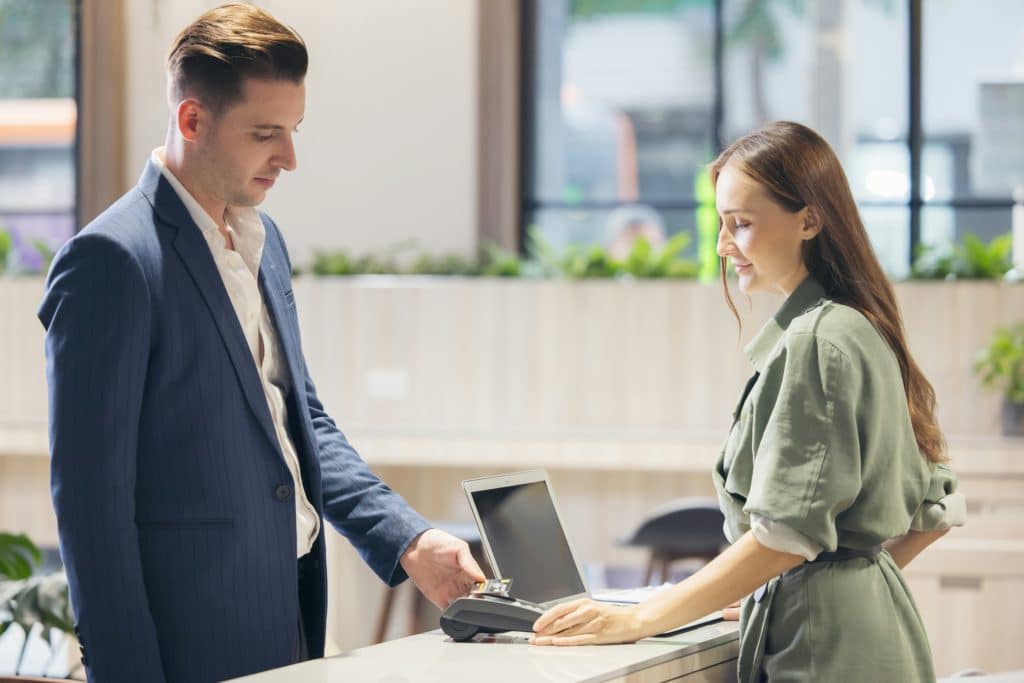
The integration of artificial intelligence (AI) is a key driver of digital transformation in the financial and banking industries, offering numerous benefits such as enhanced fraud detection, personalised customer experiences, and streamlined operations. However, fully realizing AI’s potential is impeded by significant challenges. Financial institutions must navigate a complex landscape of technical hurdles, regulatory complexities, data privacy concerns, and ethical dilemmas, necessitating a robust AI implementation strategy. Overcoming these obstacles is crucial for banks to harness AI safely and responsibly.
Data Quality and Availability
Ensuring the quality and accessibility of data is fundamental for the effective use of AI technologies in banking. AI systems, particularly those based on machine learning and deep learning, require substantial amounts of high-quality data. In banking, this data often includes sensitive personal and financial information that demands meticulous handling. Insufficient or biased training data can lead to unreliable AI outputs, affecting decision-making in areas like investment management, fraud prevention, and market analysis. Banks must invest in robust data management practices and technologies to ensure the accuracy, completeness, and unbiased nature of their data. Data integration plays a crucial role in these practices.
High-quality data is the lifeblood of AI systems. For instance, accurate fraud detection relies on historical transaction data to identify patterns indicative of fraudulent activity. Similarly, personalized customer experiences are enhanced through detailed customer profiles and transaction histories. Therefore, banks must ensure data is clean, consistent, and comprehensive. Data silos within banks often pose a significant barrier, preventing seamless data integration necessary for AI systems. Overcoming these challenges requires investing in data integration platforms and establishing strong data governance frameworks.
Risk Management and Compliance
The adoption of AI introduces new risks that must be managed within a complex regulatory environment designed to protect consumers and maintain financial stability. For instance, the EU AI Act exemplifies the growing emphasis on robust AI risk management. Banks must implement strong governance and internal controls based on AI risk management principles to address challenges such as algorithmic bias and security vulnerabilities, ensuring AI systems are deployed safely and ethically. Regulatory compliance is crucial in this context, requiring a comprehensive understanding of the regulatory landscape and a proactive approach to compliance.
Banks must also ensure that AI systems are transparent and explainable. Regulators are increasingly demanding that financial institutions provide explanations for decisions made by AI, particularly in areas like credit scoring and loan approvals. This “black box” nature of some AI systems can be problematic, as it can be challenging to understand how decisions are made. Algorithmic bias can further impact transparency and fairness, making it essential to develop AI models that are interpretable and provide clear documentation of AI processes as crucial steps toward regulatory compliance.
Legal and Ethical Considerations
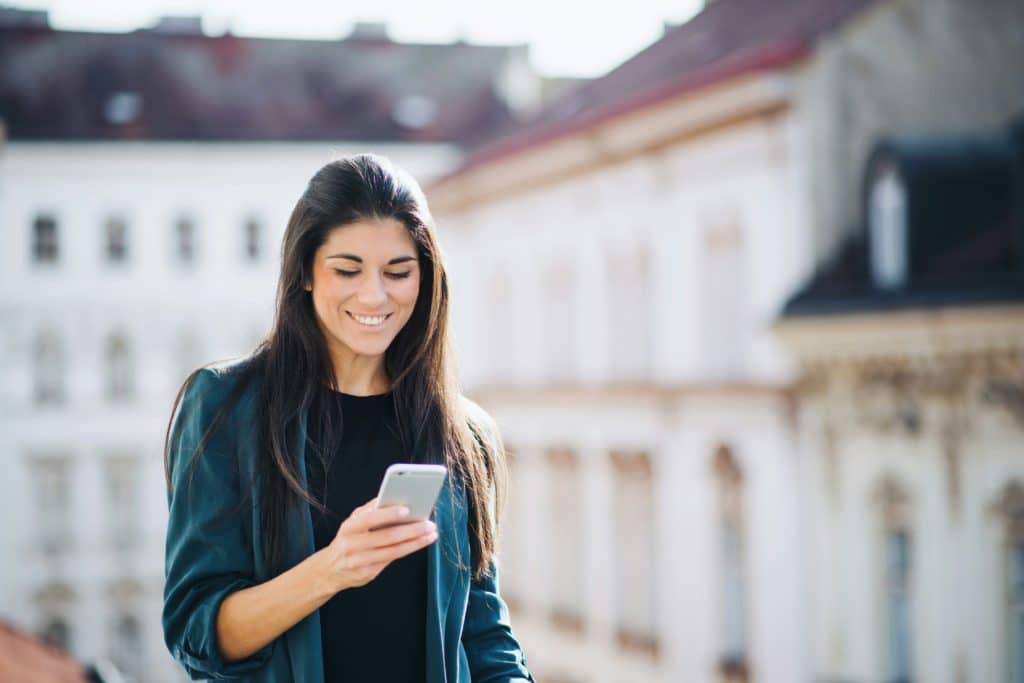
AI systems pose challenges related to data privacy, algorithmic fairness, and transparency that financial institutions must carefully navigate. Issues related to these challenges are paramount. Legal counsel is essential for complying with regulations and safeguarding consumer rights. The use of AI in credit scoring and fraud detection, for instance, highlights concerns about potential bias in machine learning models, which can erode trust. Financial institutions must strive to develop AI systems that are transparent, fair, and accountable to maintain public confidence. Additionally, ethical AI is crucial for maintaining consumer trust.
Ensuring fairness in AI involves addressing biases that may arise in training data or through algorithmic processes. Biased AI systems can lead to discriminatory practices, which not only damage the institution’s reputation but also result in legal repercussions. Implementing fairness-aware machine learning techniques and regularly auditing AI systems for biases are critical measures to uphold ethical standards.
Security Risks
AI systems handling sensitive financial data create new security and financial risks. Inadequate security measures can result in data breaches, compromising data integrity and privacy. Banks must implement robust security protocols to protect against cyber threats and ensure the secure use of AI tools. Cybersecurity plays a crucial role in preventing data breaches by safeguarding sensitive information. This includes adhering to strict data security standards and employing advanced encryption techniques. Continuous monitoring and updating of security measures are essential to counter evolving threats.
The dynamic nature of cyber threats necessitates a proactive approach to AI security. Financial institutions must stay ahead of potential vulnerabilities by investing in advanced cybersecurity technologies, such as AI-driven threat detection systems that can identify and respond to threats in real time. Additionally, regular security training for employees and conducting thorough security assessments are vital practices to safeguard against breaches.
Operational and Strategic Challenges in the Financial Sector
Integrating AI into banking operations within the financial sector requires a strategic approach and substantial investment in infrastructure, talent, and training. Developing comprehensive internal policies and governance frameworks aligned with overall risk management strategies is essential. Banks must also consider the long-term implications of AI, including potential workforce impacts and the need for continuous technological adaptation. This involves not only hiring and training AI experts but also fostering a culture of innovation and adaptability within the organization. The workforce impacts of AI adoption include job displacement in certain roles and the creation of new opportunities in AI management and oversight.
AI’s integration impacts various facets of the banking sector. For instance, operational processes like customer service, loan processing, and compliance reporting can be vastly improved through automation and AI-driven analytics. However, these improvements require a substantial shift in the bank’s operational framework, which includes updating legacy systems, investing in new technologies, and reskilling employees to work alongside AI systems
Regulatory Compliance and Legal Framework
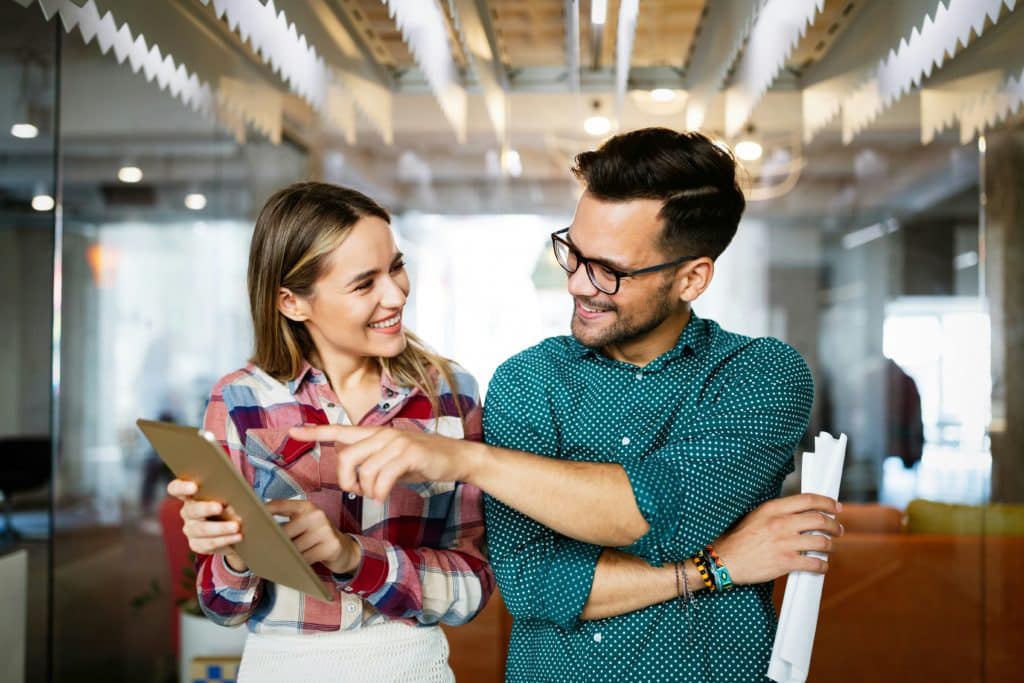
The banking industry operates within a stringent regulatory framework, and AI introduces additional compliance complexities in financial services. Ongoing updates to risk management and control measures are necessary to keep pace with evolving regulations. Compliance management systems play a crucial role in managing these updates. The increasing use of AI for tasks like regulatory reporting highlights the need for a deep understanding of the legal landscape. Regulatory reporting is essential in maintaining compliance. Close collaboration with regulators is crucial to ensure AI applications adhere to current laws and prepare for future regulations. Banks must engage in continuous dialogue with regulatory bodies to stay informed and compliant.
Regulatory compliance in AI adoption is a multifaceted challenge. Banks must navigate different regulations across various jurisdictions, each with its own requirements for data handling, privacy, and AI system transparency. This complexity necessitates a comprehensive compliance strategy that includes regular audits, compliance training for staff, and the implementation of compliance management systems that can adapt to changing regulations.
Conclusion
AI adoption in banking presents multifaceted challenges, including issues related to data quality, risk management, legal and ethical considerations, security, and regulatory compliance. To realize AI’s benefits while mitigating risks, banks must develop robust AI risk management strategies, implement comprehensive internal policies, and actively engage with regulators. Effective AI risk management strategies and internal policies are crucial for overcoming the challenges of AI adoption. Prioritizing responsible and secure AI use is essential for protecting consumers and ensuring the industry’s long-term sustainability. By addressing these challenges head-on, banks can effectively leverage AI to drive innovation and improve their services.
Banks that successfully integrate AI into their operations will be better positioned to offer superior customer experiences, improve operational efficiencies, and maintain a competitive edge in the rapidly evolving financial landscape. However, this requires a commitment to overcoming the significant hurdles posed by AI adoption. Financial institutions must be proactive in addressing these challenges, investing in the necessary infrastructure, talent, and governance frameworks to ensure AI’s safe and effective deployment. The journey to full AI integration in banking is complex, but with strategic planning and robust risk management, the benefits far outweigh the challenges.