How does the ChatGPT actually work?
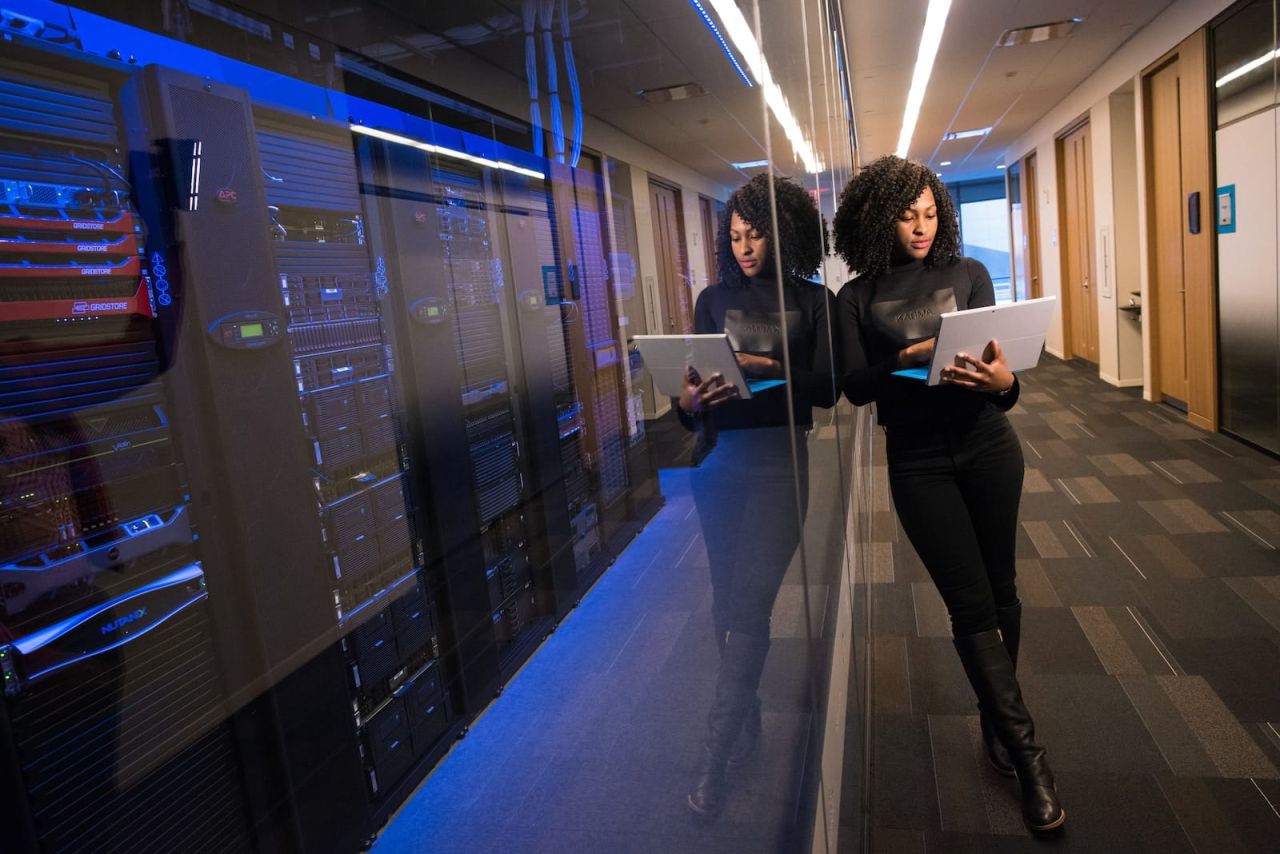
The intrigue surrounding how ChatGPT functions stems from its proficiency in understanding and producing human-like language. In this article, we will take a deep dive into the inner workings of this artificial intelligence program as InvestGlass now include chatgpt for banking and sales processes.
Large Language Model
At its core, ChatGPT is built upon a large language model. This essentially means it’s a form of artificial intelligence designed to understand and generate human language. Think of it as a neural network that’s been trained on vast amounts of text data, aiming to mimic the way the human brain processes language.
Training Data
A large part of what makes ChatGPT efficient is the training data it’s exposed to. The more diverse and comprehensive this data is, the better ChatGPT becomes at recognizing patterns and generating appropriate responses. This is where the concept of supervised learning comes into play. The model is exposed to vast datasets, with both user queries and the best possible responses, to learn patterns and refine its language models. The approach of masked language modelling is a variation of predicting the next token. In this method, certain words within the input phrase are substituted with a unique token, often denoted as [MASK].
Human Brain and Neural Network
To grasp how ChatGPT works, it’s helpful to draw a parallel between the human brain and a neural network. Just as our brains recognize patterns and learn from experiences, neural networks adjust and fine-tune themselves through a learning process. Deep learning, a subfield of machine learning, uses neural networks to achieve complex tasks, such as language translation, sentiment analysis, and speech recognition.
The training process of ChatGPT can be likened to teaching a child a language. Through reinforcement learning, a technique in machine learning, the model receives human feedback for its outputs. This feedback, often in the form of a reward model, helps the system understand context and improve over time.
Language Model & Natural Language Processing
Central to the magic of ChatGPT is the concept of natural language processing (NLP). NLP allows ChatGPT to understand context, recognize sentiment, and produce human-like responses. The underlying structure of language models in ChatGPT is based on the principles of NLP.
One key task in NLP is next-token prediction or forecasting the next word in a sequence. When you ask ChatGPT a question, the model essentially utilizes the fine-tuning it has undergone to predict the most likely next word, and the word after that, and so on, until a meaningful text response is constructed.
Fine-tuning and Learning Process
Although ChatGPT starts with a strong foundation in its initial training, what makes it truly stand out is the ability to fine-tune. This refinement is achieved through supervised learning, where the model is trained on a labeled dataset to understand human input and produce appropriate outputs.
The fine-tuning of ChatGPT is a continuous process, and as it’s exposed to new words, phrases, and nuances, it becomes more adept at text generation, answering questions, and essentially everything related to human language.
Data Sovereignty and ChatGPT
Understanding how ChatGPT works is not just a matter of appreciating its impressive language modelling but also recognizing the implications related to data sovereignty. ChatGPT, like other large language models, relies on vast amounts of input data, gathered from diverse sources through data collection processes. In training, ChatGPT, the so-called “supervised approach” involves feeding it with masked language modelling tasks, text-based and numerical data alike. This enhances the model’s capability to recognize human speech patterns, improve reading comprehension, and generate responses that align closely with the probability distribution of real-world human language.
However, this entire training process raises questions. Specifically, how does ChatGPT handle the input data in terms of sovereignty? As ChatGPT is fine-tuned for specific tasks, it’s vital to identify areas where user data might be at risk. Is the model’s training, especially the so-called fine-tuning, respecting the bounds of data sovereignty? The model’s capability to understand the context and provide multiple outputs based on word-based inputs is remarkable, but the whole process should maintain a balance with users’ rights to their own data.
The introduction of a new reward model for ChatGPT has aimed to refine its responses further, but there’s a pressing need to ensure that this doesn’t come at the expense of data sovereignty. As efforts to fine-tune ChatGPT continue, it becomes paramount to ensure that data privacy and sovereignty remain at the core of these endeavours, ensuring that common sense principles regarding user data are upheld.
Conclusion
In the realm of artificial intelligence, ChatGPT represents a significant stride in language modelling. Its capability to understand and answer questions, combined with its continuous fine-tuning, makes it an invaluable tool in the ever-evolving field of computer science. The next time you interact with ChatGPT or similar virtual assistants, you’ll have a clearer insight into the deep dive processes that enable such human-like interactions. InvestGlass now includes chat for specific task processes: portfolio rebalancing, and sales optimisation. As investors already include rule-based engine for financial intermediates ( MIFID, FIDLEG SLFIN) it is not needed to train chatgpt in advance.