Client Lifecycle Management Banking: The Ultimate Guide
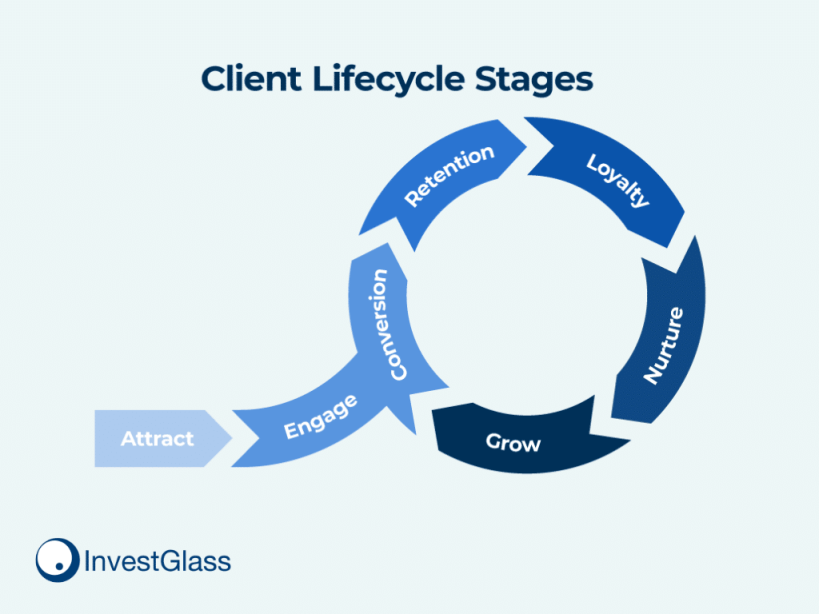
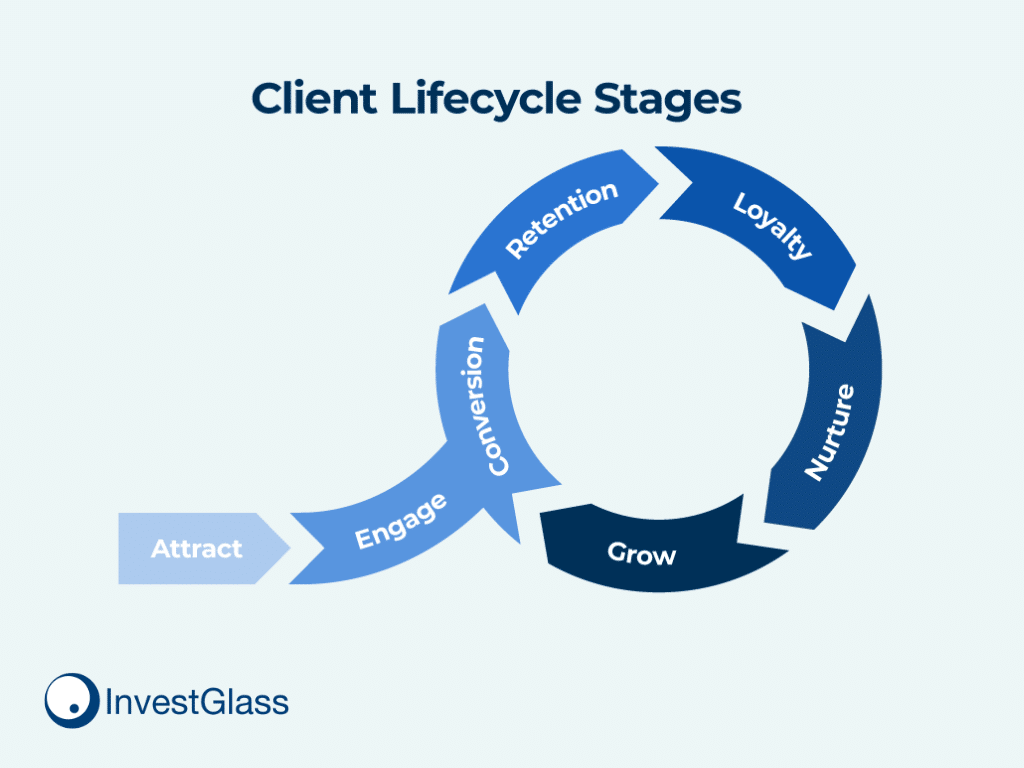
Client lifecycle management banking involves managing the customer journey from initial contact to offboarding. Leveraging market data is crucial for analyzing financial information and making informed decisions throughout the client lifecycle. It’s essential for building trust and ensuring compliance. This article covers key stages, strategies, and technologies enhancing client lifecycle management banking. Additionally, understanding the AI learning journey is vital as it equips banking professionals with the skills needed to navigate and optimize these processes.
Key Takeaways
Client lifecycle management (CLM) is vital for financial institutions to build trust and ensure customer satisfaction throughout the customer journey.
Effective onboarding, relationship management, and retention strategies are key stages in CLM that benefit from data-driven approaches and the integration of AI tools to analyze data, gather insights, and visualize information.
Emerging technologies, such as AI and machine learning, are transforming CLM processes, enhancing efficiency, compliance, and personalization in customer interactions. The AI learning journey is accessible to individuals across diverse backgrounds, providing structured learning paths and practical experiences tailored for different roles and organizations.
Understanding Client Lifecycle Management in Banking
Client lifecycle management (CLM) encompasses the supervision of customer interactions from their inception through to ongoing engagement and eventual offboarding, effectively managing the entire customer journey. For financial institutions, proficient CLM is crucial for fostering trust and reducing conflicts across the client’s experience. This requires constant vigilance and refinement of business processes in response to changing consumer demands.
At the core of effective CLM lies robust communication. By keeping a transparent dialogue open, banks are able to fortify their relationships with clients and proactively resolve and identify potential risks and issues. Not only does this bolster customer satisfaction, but it also aligns with regulatory requirements ensuring compliance. The AI learning journey is an integral part of these continuous improvement efforts, providing structured learning paths and practical experiences tailored for different roles and organizations.
AI technologies in CLM simulate human intelligence by performing complex tasks that traditionally required human cognitive abilities, such as analyzing customer data and predicting client needs.
For financial entities eager to maintain a competitive edge, excelling in CLM is key for securing enduring prosperity in an aggressive marketplace.
Definition and Importance of CLM in Banking
Customer Lifecycle Management (CLM) is a cornerstone of the banking industry, encompassing the entire customer journey from initial onboarding to eventual offboarding. Effective CLM is indispensable for financial institutions aiming to deliver personalized services, enhance customer satisfaction, and foster long-term loyalty. The advent of artificial intelligence (AI) and machine learning (ML) has further underscored the importance of CLM, enabling banks to analyze vast amounts of customer data and tailor their services accordingly. By leveraging AI and machine learning, banks can gain deeper insights into customer behavior, preferences, and needs, thereby offering more relevant and timely services that significantly boost customer satisfaction. Additionally, the AI learning journey plays a crucial role in these advancements, making AI skills accessible to individuals from diverse backgrounds and supporting structured learning paths tailored for different roles and organizations.
Benefits of Effective CLM
Effective CLM, by managing the entire customer journey, offers a multitude of benefits for banks, including:
Improved Customer Satisfaction: Personalized services and offers tailored to individual customer needs can significantly enhance customer satisfaction and loyalty.
Increased Revenue: By identifying cross-selling and upselling opportunities, effective CLM can drive additional revenue streams.
Reduced Churn: Timely and relevant services help in reducing customer churn, ensuring higher retention rates.
Enhanced Customer Insights: CLM provides valuable insights into customer behavior and preferences, enabling banks to make informed decisions and continuously improve their services. Additionally, the AI learning journey supports the development of personalized services by equipping teams with the skills to leverage AI for deeper customer insights.
Challenges in Implementing CLM
Implementing effective CLM in banking to enhance the customer journey is not without its challenges. Key hurdles include:
Integration of Multiple Systems and Data Sources: Seamlessly integrating various systems and data sources is crucial for a unified view of the customer.
Advanced Analytics and Machine Learning Capabilities: Leveraging advanced analytics and machine learning is essential for extracting actionable insights from customer data. Additionally, embarking on the AI learning journey is a significant challenge, as it involves acquiring the necessary skills and knowledge through structured learning paths and practical experiences.
Real-Time Data Processing and Decision-Making: The ability to process data in real-time and make swift decisions is vital for delivering timely and relevant services.
Personalization and Contextualization of Services: Offering personalized and contextually relevant services requires a deep understanding of customer behavior and preferences.
Continuous Monitoring and Improvement: Ongoing monitoring and refinement of CLM processes are necessary to adapt to changing customer needs and market conditions.
Key Stages of Client Lifecycle Management
The process of managing the client lifecycle, or customer journey, covers the entire span from the first interaction with prospective clients to fostering enduring loyalty. At every point in this progression, leveraging data is essential for improving customer interactions and guiding decisions throughout their learning path. The significance of top-tier data cannot be overstressed as it impacts each phase, ranging from acquiring customers to keeping them engaged. Additionally, embarking on an AI and machine learning course or journey is crucial for continuously enhancing these interactions and making informed decisions. This continuous enhancement is part of the broader AI learning journey, which is accessible to individuals across diverse backgrounds and supported by structured learning paths and practical experiences.
We will delve into the critical phases of CLM comprehensively in subsequent sections: initiating client onboarding, nurturing active relationships, and implementing tactics to maintain clientele. These stages are instrumental in ensuring a seamless and rewarding experience for both clients and financial organizations during their journey together.
Onboarding Process
The initiation of the client relationship is marked by a vital interaction in the customer journey—the onboarding process. Implementing rigorous Know Your Customer (KYC) measures not only ensures regulatory compliance but also aids in gaining insight into clients’ necessities. Enhancing this critical stage with compliance diligence and tailored offerings can elevate its effectiveness, as evidenced by StoneX’s adoption of an integrated system for handling client data and documentation throughout both onboarding and ongoing management.
Incorporating AI projects into the onboarding process can provide deeper insights and improve compliance, making the procedure more efficient and effective. Additionally, embarking on an AI learning journey can further enhance these efforts by providing structured learning paths and practical experiences tailored for different roles and organizations.
Digital onboarding has been revolutionized by several financial institutions setting exemplary standards. For example, First Abu Dhabi Bank embraced a cloud-powered solution for compliance that placed it at the forefront of digital customer integration within the banking industry in UAE. Northern Ireland is the most northern region. Trust similarly refined its processes to welcome new clients digitally, which bolstered their strategy learning plan for investing capital funds more dynamically.
These instances serve as testament to how proficiently managing initial interactions with clients lays down a robust groundwork for enduring relationships between clientele and financial establishments.
Active Relationship Management
Maintaining robust client connections through consistent engagement and tailored offerings is the essence of active relationship management, which encompasses the entire customer journey. The integration of AI tools can significantly bolster these relationships, allowing for prompt replies and well-informed suggestions that still maintain a sense of empathy. Financial institutions can build AI apps to enhance client relationship management by leveraging frameworks like TensorFlow, which can be tailored to specific roles and applications in fields such as Computer Vision and Natural Language Processing. Tailoring services to individual needs plays a pivotal role in elevating customer satisfaction and fidelity at this juncture. Additionally, the AI learning journey supports these efforts by providing structured learning paths and practical experiences tailored for different roles and organizations.
By streamlining complex directions into concise summaries and shortening the time it takes to respond, generative AI can notably augment the productivity of communication with clients. In conjunction with artificial intelligence, such AI systems are adept at personalizing experiences for users by enhancing their ability to navigate processes more easily and accessibly.
Nevertheless, striking an equilibrium between AI capabilities and human insight remains crucial to preserve an empathic, customer-centric service ethos.
Client Retention Strategies
Maintaining enduring connections with clients hinges on understanding the customer journey and the effectiveness of customer retention strategies. In the context of banking, loyalty programs and proactive engagement approaches serve as potent methods for boosting client retention rates. The introduction of such loyalty schemes can motivate customers to sustain their association with a financial institution by offering them incentives.
Tailoring services to meet individual client requirements proactively aids in bolstering retention while diminishing churn. Personalization markedly elevates both customer satisfaction and fidelity to the bank. Through prioritizing these tactics, financial institutions are able to cultivate steadfastness among their clientele and secure an advantageous position amidst fierce market competition. Additionally, incorporating an AI learning journey helps in understanding customer needs and improving retention rates.
Leveraging AI and Machine Learning in CLM
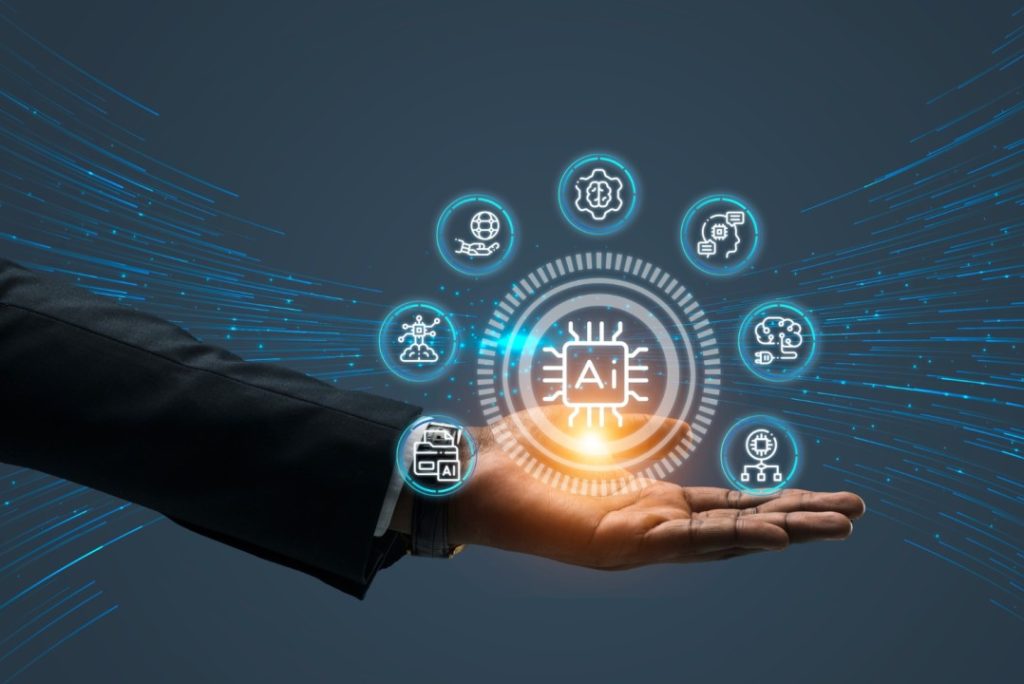
Incorporating AI and machine learning into the customer journey and management of client lifecycles could fundamentally transform the operations of financial institutions. By utilizing AI, these establishments can refine marketing strategies, sales tactics, and customer support services at every point of interaction with clients. For instance, around the clock availability of AI chatbots ensures immediate reply capabilities and suggestions without needing human input.
Building a strong foundation in the programming language, and mathematics is crucial for a successful journey in AI development. Various online courses offer foundational knowledge and practical skills essential for understanding AI and its applications, including specific training related to generative AI and ethical considerations in AI development. This comprehensive approach is part of the broader AI learning journey, making AI skills accessible to individuals from diverse backgrounds.
The application of automation within Know Your Customer (KYC) protocols has the potential to elevate both efficiency levels and adherence to regulations by simplifying steps related to documentation verification. Through automating monotonous tasks in marketing efforts, handling campaigns becomes more streamlined.
AI’s predictive analytics tools are adept at spotting customers who may be considering a departure from the business’s services. This enables companies to take proactive measures. Dynamic pricing models driven by artificial intelligence have the ability to modify prices instantly according to prevailing market trends as well as specific patterns in consumer behavior. Embracing these innovative technologies substantially bolsters decision-making prowess within financial entities.
AI Skills Required for CLM
To implement effective CLM and manage the customer journey, banks need to develop a robust set of AI skills, including:
Machine Learning: Essential for analyzing customer data and providing personalized services.
Deep Learning: Crucial for understanding complex customer behaviors and preferences.
Natural Language Processing (NLP): Important for analyzing customer feedback and sentiment.
Predictive Analytics: Helps in forecasting customer behavior and preferences.
Generative AI: Useful for creating personalized offers and services that resonate with individual customers.
Embarking on an AI learning journey is vital for acquiring these skills, as it provides structured learning paths and practical experiences tailored for different roles and organizations.
Personalized Banking with Generative AI
Introduction to Generative AI in Banking
Generative AI is revolutionizing the banking industry by enabling highly personalized experiences for customers. By leveraging advanced machine learning and deep learning techniques, generative AI can analyze vast amounts of customer data to create tailored financial products and services. This technology has the potential to transform the way banks interact with their customers, significantly improving customer satisfaction and loyalty. Through the use of generative AI, banks can offer more relevant and timely services, enhancing the overall customer experience and fostering long-term relationships. As part of this transformation, the AI learning journey plays a crucial role in equipping professionals with the skills needed to effectively implement and manage these advanced technologies.
Generative AI for Personalized Banking Experiences
Generative AI can be utilized to create personalized banking experiences in several innovative ways. For instance, it can generate customized financial plans based on a customer’s unique financial goals and risk tolerance. Additionally, generative AI can craft personalized investment portfolios that align with a customer’s individual needs and preferences. By employing generative AI, banks can provide their customers with a more personalized and human-like experience, which not only improves customer satisfaction but also strengthens customer loyalty. This level of personalization ensures that customers feel valued and understood, leading to a more engaging and fulfilling banking experience. As part of this process, the AI learning journey plays a crucial role in creating these personalized financial plans and investment portfolios.
Data Analysis and Its Role in CLM
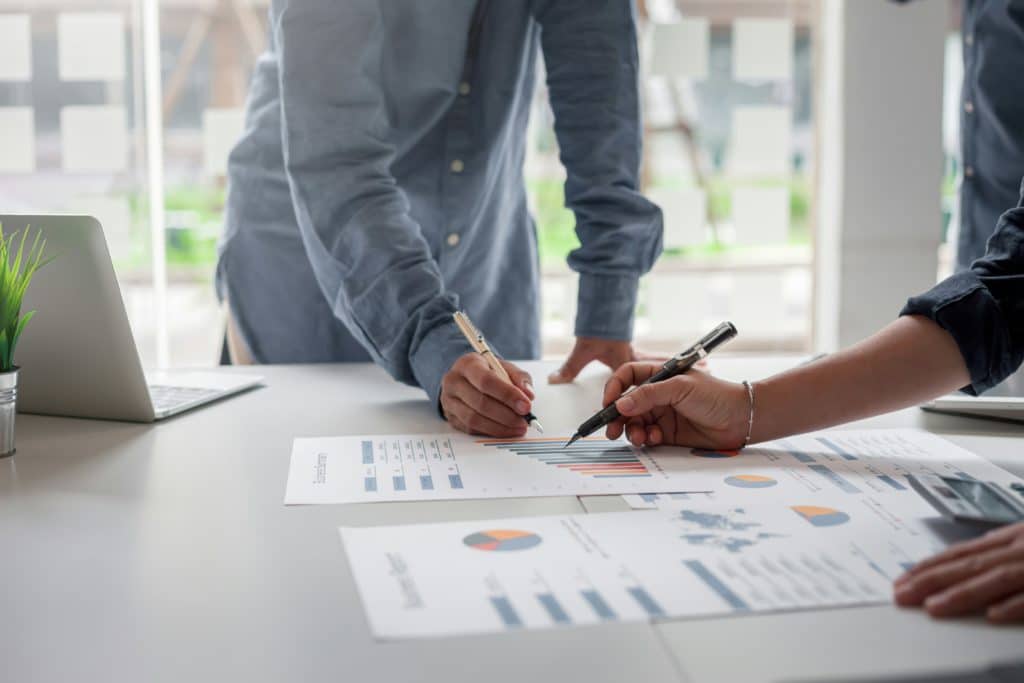
Understanding the customer journey through data analysis is essential in the management of client lifecycles, as it supports decisions rooted in data that enhance customer interactions and elevate service standards. The field of data science is dedicated to uncovering trends within unprocessed data and discerning user requirements. By employing feedback from customers, financial institutions and healthcare industry can leverage data analytics to pinpoint shortcomings in their offerings and tackle problems that may otherwise lead to customer attrition. The AI learning journey is a crucial part of acquiring these data analysis skills, making it accessible to individuals from diverse backgrounds.
AI tools are instrumental for conducting real-time analyses which enable swift detection of potential risks within contracts and any deviations from established norms. AI technology has the capability to autonomously pull out vital information from contract metadata, thereby enhancing both accuracy of data and monitoring compliance. These tools automate comparisons between contractual clauses to effectively oversee those with a higher risk profile by flagging irregularities for additional scrutiny.
Utilizing such advanced instruments affords a more transparent view into ongoing contract commitments and deadlines, thus fostering ahead-of-time handling of renewals. Take BNP Paribas’s ‘One KYC’ initiative as an illustration. This award-winning project amalgamated client initiation processes with risk oversight functions resulting in significant improvements in how services are delivered to clients.
Data Governance and Security
Robust data governance and security measures are fundamental to managing the customer journey and effective CLM. Banks must ensure the integrity and confidentiality of customer data through:
Data Encryption and Anonymization: Protecting sensitive customer information from unauthorized access.
Access Controls and Authentication: Ensuring that only authorized personnel have access to customer data.
Data Quality and Validation: Maintaining high standards of data quality and accuracy.
Compliance with Regulatory Requirements: Adhering to legal and regulatory standards to build trust with customers.
Continuous Monitoring and Auditing: Regularly monitoring and auditing data governance and security processes to ensure ongoing compliance and data integrity.
By implementing effective CLM and leveraging AI and ML capabilities, banks can significantly improve customer satisfaction, increase revenue, and reduce churn. However, this requires careful consideration of the challenges and requirements involved, including the development of AI skills and the implementation of robust data governance and security measures. Part of this process involves embarking on an AI learning journey to better understand and implement these measures effectively.
Technology and Infrastructure
Cloud Computing and SaaS Solutions for CLM
Cloud computing and Software as a Service (SaaS) solutions are essential components for implementing generative AI in client lifecycle management (CLM). Cloud computing offers the scalability and flexibility required to handle large volumes of customer data, while SaaS solutions provide the necessary infrastructure and tools to deploy generative AI effectively. By leveraging cloud computing and SaaS solutions, banks can quickly and efficiently implement generative AI, leading to improved customer satisfaction and loyalty. These technologies also help reduce operational costs and enhance efficiency, allowing financial institutions to focus on delivering superior customer experiences. The integration of cloud-based AI tools and systems ensures that banks can stay ahead of market trends and continuously innovate their CLM processes. Additionally, embarking on an AI learning journey is crucial for leveraging these solutions effectively, as it equips individuals with the skills needed to navigate and implement generative AI across various roles and organizations.
Integrating Generative AI Tools
Generative AI has the potential to transform the customer journey by generating customized content and streamlining intricate procedures. When AI suggests personalized content, it can boost both customer involvement and conversion rates. By leveraging client information and preferences, generative AI is capable of producing content that aligns more closely with user interests.
In e-commerce, generative AI elevates the shopping experience by improving search comprehension and delivering pertinent results. Combining human-centric design principles with these tools can help demystify complicated processes while enhancing overall engagement for consumers.
To ensure successful deployment of reinforcement learning, it’s imperative that training data for generative AI tools be free from bias and meticulously curated. This strategy fosters innovation while unlocking a myriad of advantages across diverse sectors. As part of this integration, embarking on an AI learning journey is crucial to equip individuals with the necessary skills and knowledge to effectively utilize these tools in CLM.
Risk Management and Compliance
As AI becomes increasingly integrated into various industries, risk management and compliance have become crucial aspects to consider. In this section, we will explore the importance of AI-powered risk management in Contract Lifecycle Management (CLM), compliance and regulatory requirements, and best practices for risk management and compliance.
AI-Powered Risk Management in CLM
Contract Lifecycle Management (CLM) is a critical process that involves managing contracts from creation to expiration. AI-powered risk management in CLM can help identify potential risks and mitigate them before they become major issues. By analyzing large amounts of data, AI algorithms can detect patterns and anomalies that may indicate potential risks, such as non-compliance with regulatory requirements or contractual obligations.
AI-powered risk management in CLM can also help automate the contract review process, reducing the time and effort required to review contracts manually. This can lead to increased efficiency and reduced costs. Additionally, AI-powered risk management can provide real-time monitoring and alerts, enabling organizations to respond quickly to potential risks and minimize their impact.
Compliance and Regulatory Requirements
Compliance and regulatory requirements are critical aspects of risk management and compliance. Organizations must ensure that they comply with relevant laws, regulations, and industry standards to avoid fines, penalties, and reputational damage. AI can help organizations comply with regulatory requirements by analyzing large amounts of data and identifying potential compliance risks.
AI can also help organizations stay up-to-date with changing regulatory requirements by providing real-time monitoring and alerts. This can enable organizations to respond quickly to changes in regulatory requirements and minimize the risk of non-compliance.
Best Practices for Risk Management and Compliance
To ensure effective risk management and compliance, organizations should follow best practices, including:
Implementing AI-powered risk management tools: AI-powered risk management tools can help identify potential risks and mitigate them before they become major issues.
Conducting regular risk assessments: Regular risk assessments can help organizations identify potential risks and develop strategies to mitigate them.
Developing a compliance program: A compliance program can help organizations ensure that they comply with relevant laws, regulations, and industry standards.
Providing training and education: Providing training and education to employees can help ensure that they understand the importance of risk management and compliance.
Monitoring and reporting: Monitoring and reporting can help organizations identify potential risks and respond quickly to changes in regulatory requirements.
By following these best practices, organizations can ensure effective risk management and compliance, minimizing the risk of fines, penalties, and reputational damage.
Implementing Effective CLM Systems
Incorporating sophisticated systems for client lifecycle management (CLM) and enhancing the customer journey necessitates the use of cutting-edge solutions, including automated workflows computer systems. Such technologies play a vital role in boosting operational efficiency and aiding decision-making processes that are based on reliable information. For instance, a leading financial institution in the United States elevated its operational performance by shifting to CLM systems powered by automation. The AI learning journey is an integral part of developing and implementing these systems, making them accessible to individuals across diverse backgrounds.
The deployment of AI agents has the potential to transform customer interactions significantly, ensuring more seamless handovers between various service departments. It is also imperative to engage consistently in thorough data cleaning and upkeep protocols so as to preserve high standards of data integrity and enhance the overall functionality of these intelligent systems.
Taking such actions is indispensable when aiming to guarantee that the information utilized within CLM frameworks remains precise and current, thus supporting their effectiveness in ai applications.
Ethical Standards and Compliance in CLM
Customer journey, ethical standards, and compliance are critical aspects of client lifecycle management. Maintaining compliance ensures adherence to laws and regulations, which is vital for building trust with clients. Automated compliance monitoring helps businesses adhere to legal regulations and internal policies throughout the contract lifecycle.
Ethical frameworks help manage client data while ensuring adherence to legal standards. Effective compliance involves appointing dedicated officers to oversee adherence to ethical guidelines. Training programs help employees understand and apply ethical standards in their roles. The AI learning journey is an integral part of understanding and implementing these ethical standards and compliance measures.
Maintaining data quality and security is crucial for maximizing the effectiveness of AI in customer lifecycle management. Fostering a culture of ethics beyond mere compliance helps organizations navigate complex ethical dilemmas and maintain high standards.
Case Studies: Successful CLM in Financial Institutions
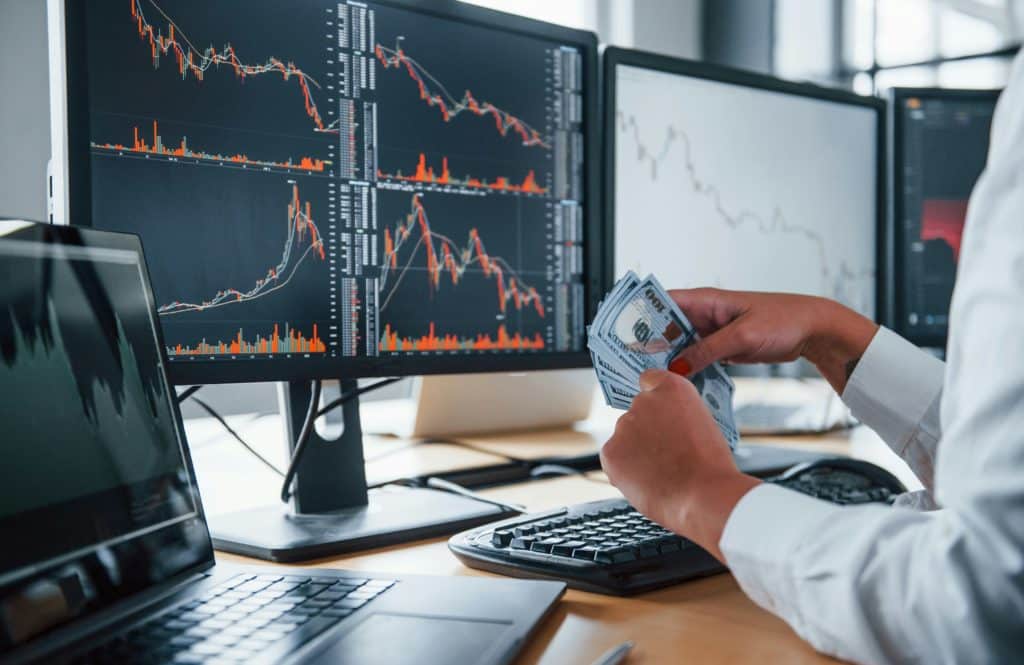
Case Study: Enhancing Client Lifecycle Management at HSBC
Background: HSBC, one of the world’s largest banking and financial services organizations, recognized the need to improve its client lifecycle management (CLM) processes to enhance customer satisfaction and operational efficiency. The bank aimed to streamline its onboarding process, improve relationship management, and boost client retention rates.
Challenge: HSBC faced challenges in integrating multiple systems and data sources, which resulted in fragmented customer data and inefficient processes. The bank needed to leverage AI and machine learning to analyze vast amounts of customer data and provide personalized services.
Solution: HSBC implemented a comprehensive CLM strategy by adopting advanced AI tools and machine learning algorithms. The bank utilized AI-powered chatbots to enhance customer interactions and streamline the onboarding process. Additionally, HSBC leveraged predictive analytics to identify at-risk customers and offer personalized retention strategies.
Outcome: By integrating AI and machine learning into its CLM processes, HSBC achieved a 30% reduction in onboarding time and a 25% increase in client retention rates. The bank also improved customer satisfaction by offering more personalized services and timely support.
Conclusion: HSBC’s strategic application of AI and machine learning in CLM not only solidified customer relationships but also opened doors for growth opportunities. By examining HSBC’s real-world scenario, other financial institutions can implement similar methods to enhance their own CLM systems and achieve superior results. An essential part of achieving success in these case studies is the AI learning journey, which provides structured learning paths and practical experiences tailored for different roles and organizations.
Future Trends in Client Lifecycle Management
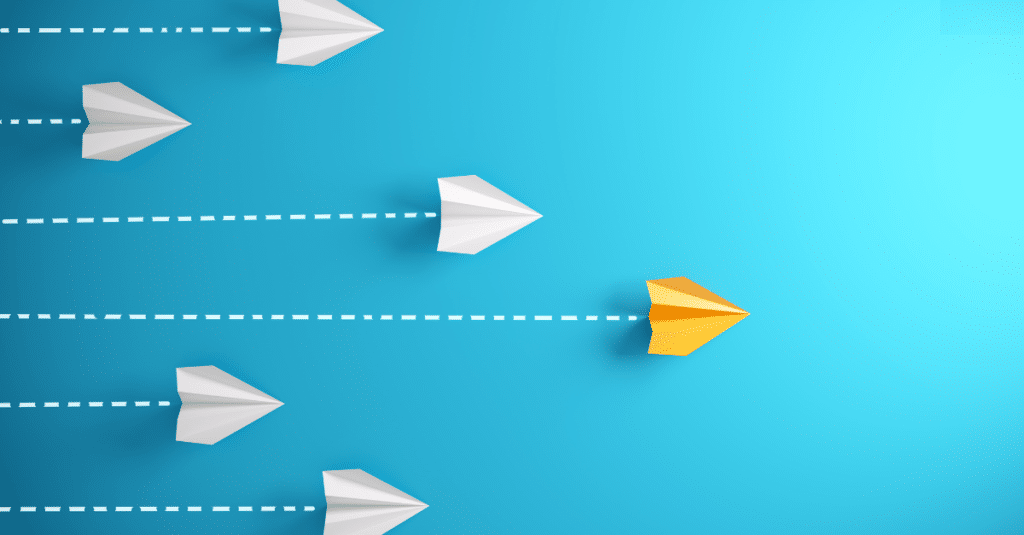
The incorporation of advanced capabilities of large language models (LLMs) into client lifecycle management is revolutionizing the customer journey for financial institutions, changing how they engage and converse with their customers. By utilizing these advanced models, banks are able to streamline communications and interactions, which significantly improves the customer experience.
Financial institutions need to proactively adapt new technologies and approaches as market trends advance in order to maintain a competitive edge. While such trends offer both chances for growth and possible hazards, by remaining vigilant and forward-thinking, these establishments can capitalize on them to enhance their client lifecycle management procedures and attain superior outcomes. Part of this adaptation involves embarking on an AI learning journey, which provides structured learning paths and practical experiences tailored for different roles and organizations.
Summary
To adeptly manage the client lifecycle in the banking sector, it’s imperative to comprehensively grasp and refine each phase of the customer journey. This encompasses instituting efficient onboarding procedures, maintaining active engagement with clients, and developing strategies for client retention. Each segment is pivotal in forging robust customer bonds while simultaneously boosting operational efficiency. The integration of AI and other machine learning algorithms into this process is vital. Utilizing generative AI tools effectively while upholding ethical standards ensures proficient client lifecycle management (CLM). The AI learning journey is an integral part of mastering CLM, providing structured learning paths and practical experiences tailored for different roles and organizations.
Financial institutions that incorporate these methodologies are better positioned to cultivate loyalty amongst their clientele, improve outcomes, and stimulate expansion. As market trends shift continuously and new technologies emerge within the industry, being proactive in adopting such innovations will prove indispensable for mastering CLM as well as securing enduring success within this competitive landscape.
Frequently Asked Questions
What is client lifecycle management (CLM) in banking?
Client lifecycle management (CLM) in banking encompasses the management of the customer journey from the initial contact to offboarding, integrating processes such as onboarding, relationship management, and retention strategies to foster trust and improve customer interactions.
How can AI and machine learning be leveraged in CLM?
AI and machine learning can significantly enhance Customer Lifecycle Management (CLM) by automating processes like KYC compliance and utilizing predictive analytics to identify at-risk customers, ultimately improving the customer journey, support, and engagement.
Leveraging these technologies can lead to more efficient operations and better customer relationships.
Why is data analysis important in CLM?
Data analysis is essential in Contract Lifecycle Management (CLM) as it enables data-driven decisions that enhance the customer journey, interactions, and service quality.
By utilizing real-time data analysis, organizations can identify contract risks and address service weaknesses effectively.
What are some client retention strategies in banking?
Implementing loyalty programs and proactively engaging with clients through personalized services are effective strategies for client retention in banking, as they enhance the customer journey.
These approaches enhance customer satisfaction and significantly reduce turnover rates.
How do ethical standards and compliance impact CLM?
Maintaining ethical standards and compliance is of paramount importance in the realm of Contract Lifecycle Management (CLM), as it solidifies law abidance throughout the customer journey, which in turn builds confidence among clients.
To safeguard these principles, it’s imperative to put into place systems for automated compliance surveillance and human oversight, establish ethical guidelines, and conduct comprehensive training initiatives for staff members.