Top AI Agent for Trading: Revolutionizing Financial Market Strategies

Wondering how to sharpen your trading strategies? An AI agent for trading analyzes market data, utilizing historical data to enhance trading strategies and make automated decisions to improve performance. In this article, we’ll explore how these systems work, their main features, and their benefits for traders.
Introduction to AI Agents in Trading
AI agents in trading are sophisticated computer programs that leverage artificial intelligence (AI) and machine learning (ML) algorithms to analyze and make decisions based on real-time market data. These agents are designed to execute trades, manage risk, and adapt to changing market conditions, making them invaluable tools for traders and investors. By continuously processing vast amounts of data, AI agents can identify patterns and trends that might be missed by human traders, allowing for more precise and timely trading decisions. This capability not only enhances trading performance but also helps in mitigating risks associated with market volatility.
Key Takeaways
AI trading agents improve financial market strategies by analyzing vast amounts of market data and adapting trading methods in real-time, leading to more informed and efficient trading decisions.
Key features of AI trading agents include real-time data analysis, adaptive learning capabilities, and robust risk management techniques, which enhance their effectiveness in dynamic market environments.
The development of AI trading agents involves a multi-step process emphasizing technical skills, data collection, model training, and compliance with regulatory standards to ensure optimal performance and risk mitigation.
Understanding AI Agents in Trading
AI agents are advanced systems designed to refine trading strategies, addressing specific market demands through their tailored functions. These agents function within an intricate multi-agent framework, collaborating to scrutinize a variety of market elements and enhance overall trading efficacy. AI agents analyze different trading pairs to optimize their strategies. Utilizing sophisticated artificial intelligence algorithms allows these agents to process numerous factors simultaneously, enabling them to make complex decisions that would typically be difficult for human traders.
The success of AI trading agents in making well-informed trade decisions hinges on the availability of high-caliber data from a wide range of sources. By synthesizing this information, AI agents can provide extensive insights into the market, covering aspects such as pricing trends and investor mood swings. Equipped with such knowledge, they possess the ability not only to carry out trades with high accuracy, but also continuously fine-tune their strategic approaches.
Incorporating AI into the realm of trading transcends mere automation. It involves enhancing decision-making processes by anchoring them in robust data analysis, which ultimately exerts a significant influence over trade outcomes.
How AI Agents Work in Trading
AI agents in trading operate by meticulously analyzing large volumes of historical and real-time market data, including prices, volumes, and other pertinent information. They utilize this data to identify patterns, trends, and correlations, which are then used to make predictions about future market movements. Based on these predictions, the AI agent can execute trades, adjust risk management strategies, and optimize overall trading performance. The integration of machine learning algorithms allows these agents to continuously learn and adapt, refining their trading strategies to stay effective in dynamic market environments. This adaptability ensures that AI agents can respond swiftly to market shifts, making informed decisions that enhance trading outcomes.
Key Features of AI Trading Agents

AI trading agents are equipped with distinct characteristics that set them apart from conventional trading systems. Their most notable attribute is the capacity to process and interpret large quantities of data, which empowers them to forecast market trends and pinpoint opportunities for trades. By leveraging insights derived from real-time information, these AI agents can execute well-informed trading decisions.
They utilize sophisticated algorithms capable of learning on the fly by analyzing changes in the market as well as patterns in investor actions. This ability to adapt enables AI agents to perpetually refine their strategies, thereby increasing their proficiency under ever-changing market scenarios.
To gain a better understanding of these aspects, we will examine each feature more closely in the following subsections.
Real-Time Market Data Analysis
The capability to scrutinize market data in real-time is critical for any AI-powered trading system. These AI agents are constantly gathering current market insights, which allows them to refine their trading strategies with precision. Crafting a trading agent requires the construction of a sturdy framework capable of handling real-time data inflows. By employing statistical tools, these systems can analyze pricing trends and pinpoint potential trades.
Incorporating high-quality exchange information along with an understanding of market sentiment significantly bolsters the decision-making process for AI-driven trading entities. With access to diverse exchange inputs and sources of sentiment analysis, these intelligent instruments can sift through extensive datasets rapidly, spotting both patterns and chances that elevate trade decision accuracy. The core strength enabling such adaptiveness and speed in AI-based traders stems from robust analysis powered by immediate access to market figures.
Historical price evaluation plays a pivotal role as AI-operated systems discern trend developments while determining the most advantageous moments for initiating or concluding trades—a key element for following prevailing trends effectively—and it enhances arbitrage efforts by promptly catching discrepancies in pricing across different venues, permitting swift action-taking measures. Persistent processing coupled with timely scrutiny endorses how well-equipped such automated agents remain when faced with dynamically shifting conditions within the markets they operate.
Risk Management Techniques
Successful trading strategies hinge on robust risk management, which is where AI agents demonstrate remarkable prowess. By employing a range of risk management tactics that adjust to fluctuating market conditions, such as dynamically modifying stop-losses, these agents help maintain control over exposure. The Portfolio Manager Agent embodies this by incorporating risk evaluations into its strategic planning for trades to align with predefined risk parameters and recalibrate positions accordingly.
The allure of enhanced trade effectiveness has led numerous financial institutions to channel investments into AI-powered systems dedicated to managing risks. An adept AI trading system must possess the agility to adapt seamlessly to the ever-changing terrain of the market while ensuring that trade execution is optimized and risks are strategically mitigated. Through this methodology, AI agents offer traders vital assistance in navigating the intricacies of financial markets with greater assurance and diminished susceptibility to loss.
Adaptive Learning Capabilities
AI trading agents are enhanced by adaptive learning, which allows them to perpetually fine-tune their approaches. Utilizing machine learning and deep learning, these agents can process real-time data alongside prevailing market conditions. An example of this is the AI trading bot from WunderTrading that employs machine learning to stay responsive and effective amidst ever-shifting market scenarios.
Specifically, reinforcement learning models excel in recalibrating strategies in alignment with ongoing market circumstances. Such dynamic adjustment and evolution bestow a competitive edge on AI agents as they can execute decisions based on informed analysis, thereby optimizing their performance. This application of sophisticated adaptive techniques empowers AI trading entities to preserve superiority within the intensely contesting realm of financial markets.
Developing an AI Agent for Trading
Creating an AI agent dedicated to trading involves a complex procedure that necessitates extensive knowledge in both technological and financial realms. These autonomous agents scrutinize market data, making choices and performing trades independently of human oversight. They refine their trading strategies dynamically, assimilating lessons from the prevailing market trends and sentiments.
In subsequent subsections, we will delve into numerous essential phases involved in the development journey of such agents.
Essential Skills Required
To develop a successful AI trading agent, an integration of technological prowess in both finance and analysis is essential. Proficiency in software development and data analytics plays a critical role for the implementation and preparation of data tailored for AI-driven trading agents. It’s imperative to possess comprehensive knowledge of machine learning methodologies as these are fundamental for forecasting market trends and refining trading strategies.
A robust grasp on financial markets significantly contributes to the comprehension of various trading tactics and risk management principles. For artificial intelligence algorithms focused on trading to function optimally, they must be fed with high-quality, accurate data inputs. Substandard or erroneous data could lead to defective decisions by the agent. Constructing an AI entity that can trade effectively requires concerted efforts from professionals with varied expertise across several domains, emphasizing teamwork among specialists equipped with these divergent capabilities.
Prerequisites and Planning
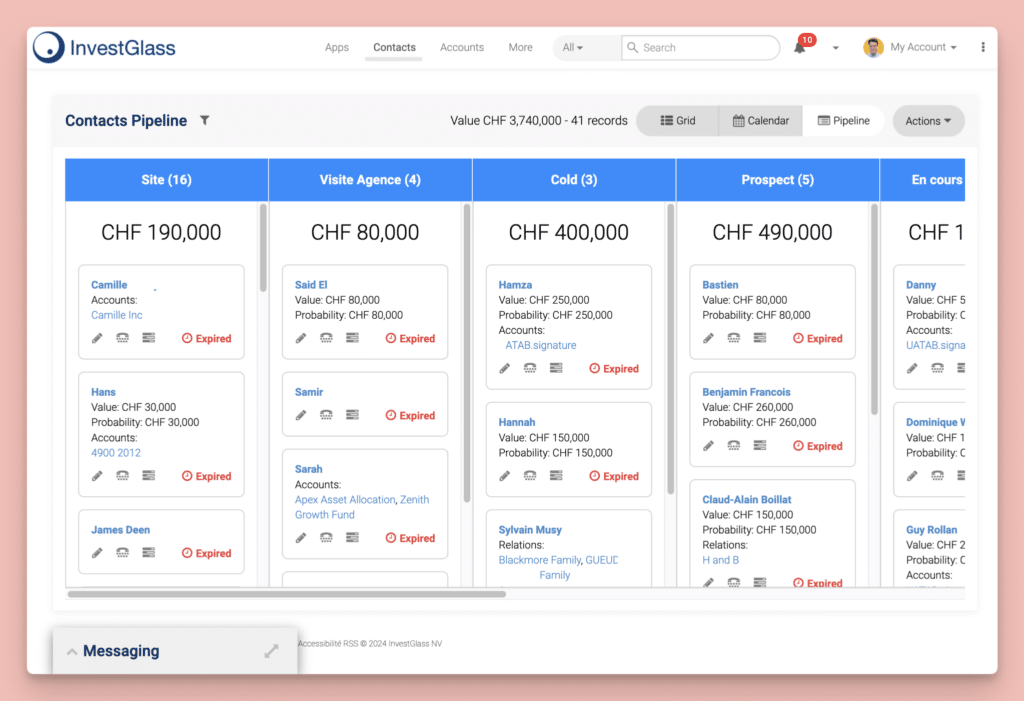
Prior to initiating the creation of an AI trading agent, it is imperative to lay down firm foundational prerequisites and a comprehensive plan. This entails constructing a sturdy architecture, guaranteeing the processing of data in real time, and embedding capabilities for adaptive learning into the system. In the realm of trading, where every millisecond counts, having a data pipeline with low latency is critical in order to prevent missed opportunities and monetary setbacks.
As part of this development endeavor, it’s necessary to incorporate various specialized agents such as the Portfolio Manager Agent and Risk Manager Agent. These agents are tasked with devising conclusive trading strategies by interpreting risk-adjusted signals and ensuring that all trades remain within predetermined risk parameters. Factoring these elements into your planning process sets up a robust groundwork essential for cultivating an effective AI trading agent.
Step-by-Step Development Process
Establishing a methodical development procedure is crucial for the construction of AI trading systems that are both efficient and capable of adaptation. This approach encompasses multiple phases, beginning with the establishment of trading goals and prerequisites, followed by crafting the system architecture and carrying out the integration of AI algorithms.
Throughout this developmental journey, it’s imperative to prioritize adaptability and efficiency. Such emphasis necessitates persistent testing and validation to enhance the refinement process for AI models, thereby boosting their effectiveness within actual trading environments. Adhering to a structured strategy serves as an instrumental means in risk reduction while striving towards accomplishing intended trading results.
Data Collection and Preparation
Collecting and preparing data is an essential process in the creation of AI agents, as it lays the groundwork for successful trading strategies. These agents are trained using both structured and unstructured forms of data to interpret a range of market signals. While structured data encompasses elements like past price movements and volume figures, unstructured data might consist of news stories or opinions shared on social media platforms.
This careful assembly and refinement of information bolsters the capacity of AI to execute sound trading choices, which can lead to enhanced performance outcomes. Having access to datasets that are not only high-quality but also varied is crucial for making precise predictions within AI-driven trading. This stage includes pre-processing tasks designed to guarantee that the dataset is devoid of errors and inconsistencies before being fed into AI models.
Training the AI Model
The education of the AI model is a pivotal step in creating an AI trading agent. Through reinforcement learning, these AI models can refine their trading strategies via a process of trial and error within simulated settings. Machine learning permits these automated agents to adapt their approaches as market conditions change.
Fine tuning hyperparameters is critical for improving the efficacy of AI models in trading activities. This requires tweaking different settings to boost both the precision and effectiveness of the model.
This preparatory stage guarantees that once deployed, the AI agent will be fully prepared to navigate actual trading environments and execute decisions based on informed judgments.
Backtesting and Optimization
The practice of backtesting entails using historical market data to simulate trading activities with the goal of gauging an AI agent’s effectiveness. Historical data is crucial for evaluating the effectiveness of trading strategies against past market conditions. By doing this, one can determine how profitable and efficient the risk management aspect of their trading strategies is. The improvement in these systems’ ability to analyze market trends stems from a feedback loop that uses previous trade outcomes.
Fine-tuning AI models is a continuous effort aimed at boosting their functionality. This process requires tweaking algorithms and various settings so that they correspond appropriately with present-day market scenarios. Both backtesting and optimization play critical roles in cultivating strong and reliable agents for AI-driven trading strategies.
Deployment and Execution
Upon confirmation of the AI agent’s reliability, it is introduced into real-time trading settings where proficient execution is essential. To enhance trade performance during deployment, intelligent order routing systems are employed. These AI agents persistently track market conditions to detect irregularities and guarantee precise decision-making processes.
Constant vigilance aids in safeguarding against execution mishaps that could result from market manipulation or external influences. This continuous oversight guarantees that the AI trading agent stays efficient and adaptable to evolving market circumstances. The implementation and fulfillment of trades represent the concluding phases in activating an AI trading agent within the marketplace.
AI-Powered Trading Platforms
AI-powered trading platforms are advanced software systems that employ AI agents to analyze and execute trades. These platforms cater to a wide range of users, from individual traders to institutional investors and financial institutions, helping them manage their trading activities more efficiently. By leveraging the capabilities of AI agents, these platforms can offer significant benefits, including improved trading performance, reduced risk, and increased operational efficiency. The ability to process real-time market data and execute trades with precision makes AI-powered trading platforms a valuable asset in the competitive world of financial markets.
Popular AI-Powered Trading Strategies
AI agents employ a variety of trading strategies to achieve optimal performance. Common strategies include arbitrage trading, trend following, market-making, sentiment analysis, and reinforcement learning. These strategies leverage AI’s ability to analyze real-time market data and make informed decisions.
Let’s explore some of these strategies in detail.
Trend Following
Trend following constitutes a trading strategy focused on leveraging market momentum rather than attempting to forecast reversals in the market. By examining historical price trends and employing technical indicators, AI agents are able to ascertain the trajectory of market trends. Utilizing historical data, these agents can identify and follow market trends more accurately, enhancing their trading decisions.
In the ever-changing conditions of the stock market, where shifts can occur swiftly, this strategy gains particular prominence. AI agents remain vigilant, tracking ongoing changes in market trends and fine-tuning their strategies as necessary to ensure sustained success within financial markets.
As a foundational approach, trend following exemplifies how artificial intelligence enhances our ability to make more informed trading choices by harnessing sophisticated analysis techniques for navigating through complex patterns and fluctuations inherent in contemporary trading landscapes.
Arbitrage
Arbitrage is the strategy of exploiting price differences across various markets to achieve risk-free profits. AI agents analyze various trading pairs to identify arbitrage opportunities, leveraging algorithmic methods to swiftly and effectively pinpoint these chances, executing trades with great precision. By processing information from multiple markets at once and taking action in mere milliseconds, these AI agents notably boost profit prospects.
Employing this approach offers a competitive edge since AI agents are capable of detecting and capitalizing on arbitrage opportunities that might elude human traders. The integration of artificial intelligence into trading activities enables practitioners to amplify their earnings while mitigating risks tied to fluctuating market conditions.
As an example of how artificial intelligence can augment the effectiveness and financial gain within the realm of trading, arbitrage stands out as a clear illustration.
Sentiment Analysis
AI agents harness sentiment analysis to assess the mood of market participants, a critical aspect in understanding market sentiment. Through natural language processing methods, these agents are able to parse and identify shifts in attitudes within the marketplace. Advanced models such as Bidirectional Encoder Representations from Transformers (BERTs) and Generative Pre-trained Transformers (GPTs) play a key role in detecting changes in this sentiment.
By collating and examining data from various social media channels and news articles, AI agents achieve a holistic perspective of market sentiments. This enables them to execute trading decisions grounded on real-time scrutiny of prevailing market trends.
Incorporating sentiment analysis into their arsenal significantly bolsters AI trading agents’ ability to make strategic choices based upon comprehensive data interpretation.
Decision Making with AI Agents
AI agents in trading utilize a variety of decision-making strategies, incorporating machine learning algorithms, statistical models, and rule-based systems. These strategies enable the AI agent to analyze market data, identify trading opportunities, and make informed decisions about when to buy or sell. Additionally, AI agents can employ natural language processing (NLP) and sentiment analysis to gauge market sentiment, providing a more comprehensive understanding of market conditions. By integrating these advanced techniques, AI agents can make more accurate and timely trading decisions, ultimately enhancing their effectiveness in the stock market.
Performance Metrics for AI Agents
The performance of AI agents in trading is typically evaluated using a range of metrics, including profit/loss, return on investment (ROI), and risk-adjusted return. Other important metrics, such as the Sharpe ratio, Sortino ratio, and Calmar ratio, are also used to assess the performance of AI agents. These metrics provide a quantitative measure of the effectiveness of the AI agent’s trading strategy, helping to identify areas for improvement. By continuously monitoring and analyzing these performance metrics, traders can ensure that their AI agents are operating at optimal levels, making informed decisions that align with their trading goals.
Challenges in AI Trading
Despite the numerous benefits they offer, AI trading systems encounter a variety of obstacles. Constructing such systems is technically complex and requires ongoing enhancements as well as a departure from conventional procedures. Operating several AI trading agents at once can create processing loads and interdependencies that have the potential to bring about delays and mistakes.
It’s imperative to grasp these difficulties in order to effectively utilize AI within the realm of trading.
Market Volatility
Market fluctuations pose a considerable obstacle for AI systems involved in trading. These advanced systems can accelerate the speed of trades, but they also have the potential to exacerbate market instability when stress levels are high, especially in times of unforeseen market occurrences. Nevertheless, AI agents engaged in trading offer valuable assistance by mitigating emotional factors like fear and greed that tend to negatively impact decision making.
By offering an impartial analysis of data, AI-driven trading mechanisms aid investors in steering clear of typical emotionally charged errors like hastily selling off assets during a downturn or buying impulsively on a whim. With the support of AI, traders are better equipped to handle periods of heightened market volatility with enhanced assurance and make more judicious decisions regarding their trades.
Regulatory Compliance
Ensuring regulatory compliance is essential when utilizing AI-powered trading models to guarantee conformity with prevailing regulations. Due to the variable nature of artificial intelligence algorithms, aligning these models with shifting legal requirements presents a significant challenge. Vigilant monitoring of legal norms is crucial in mitigating compliance risks and upholding ethical standards in trading activities.
Given the dynamic behaviors exhibited by AI algorithms, they present unique complications for regulatory governance. It is imperative that one remains abreast of modifications in policies and adjust AI models in response, as this proactive approach is key to sustaining regulatory compliance and circumventing potential legal repercussions.
Data Integrity Issues
Reliable and precise data inputs are essential for AI trading agents to operate effectively, as they depend on such data to make well-informed choices. Faulty or inconsistent data can compromise the performance of AI-driven trading strategies, resulting in potentially severe economic consequences.
In times of market volatility, AI instruments that have been conditioned under steadier circumstances may falter. During unstable market periods, the quick purchasing and selling behaviors prompted by AI algorithms could intensify market turbulence. For the dependability and success of these agents engaging in trading activities, maintaining high standards for data integrity and quality is imperative.
Future of AI Agents in Financial Markets
The outlook for AI agents within the financial markets is optimistic, as their role in decentralized finance (DeFi) expands, particularly in managing and refining liquidity. The next generation of AI trading agents will likely meld with blockchain technology to boost transactional efficiency while offering clear transparency.
AI advancements are anticipated to improve these agents’ capacity for devising tailored investment strategies that reflect individual user preferences and behaviors. This fusion of artificial intelligence into the fabric of financial markets aims to level the playing field by granting average investors access to complex trading strategies typically reserved for professionals.
As artificial intelligence continues its rapid advancement, it stands poised to significantly influence and transform how financial markets operate moving forward.
Case Studies: Successful AI Trading Implementations
Numerous instances of triumphant AI trading systems underscore the capabilities of these intelligent agents. An exemplary implementation achieved a 30% decrease in order processing times and a boost in customer satisfaction by 20%, thanks to its automation features.
These empirical examples showcase the concrete advantages that AI can bring to trading, ranging from heightened efficiency to better trading results.
Best Practices for Using AI Agents in Trading
It’s crucial to adhere to the best practices when leveraging AI trading agents, as these agents are programmed to carry out activities autonomously while requiring minimal supervision from humans, thus boosting efficiency. By integrating sentiment analysis into their strategies, traders can leverage real-time public mood and opinion data for more informed decision-making.
Employing tools like risk management plans and keeping detailed trading journals aids in fostering disciplined trading habits among traders. It’s imperative that AI models be regularly updated in order to remain compliant with evolving regulatory standards.
By sticking to these recommended protocols, traders can optimize the use of AI agents resulting in improved performance within their trading ventures.
Summary
In essence, artificial intelligence trading agents are transforming strategies within financial markets through their advanced capabilities in analyzing data, managing risk, and adapting through learning. To develop these AI agents involves a blend of technical proficiency, meticulous strategy formulation and ongoing refinement. Although there may be hurdles to overcome, the advantages they provide—such as heightened efficiency in trading activities and superior decision-making—are substantial. As artificial intelligence advances, it progresses. Its importance in shaping the future landscape of financial markets is set to grow significantly. Consequently, making complex trading strategies more accessible across the market spectrum.
Frequently Asked Questions
What are AI agents in trading?
AI agents in trading are specialized systems that analyze market data to enhance trading strategies and make informed decisions, ultimately improving trading outcomes.
How do AI trading agents manage risk?
AI trading agents effectively manage risk by dynamically adjusting stop-loss orders and incorporating comprehensive risk assessments into their trading strategies.
These approaches help mitigate potential losses and optimize performance.
What is the role of real-time market data analysis in AI trading?
Analysis of real-time market data is vital for AI trading because it permits agents to constantly collect and analyze information, which leads to well-informed and prompt trading decisions.
Such functionality significantly improves the performance and productivity of trading strategies.
What challenges do AI trading systems face?
AI trading systems encounter significant challenges, including market volatility, regulatory compliance, and ensuring data integrity.
These obstacles can impact their effectiveness and reliability in dynamic trading environments.
What is the future of AI agents in financial markets?
AI agents are poised to significantly shape the future of financial markets by becoming integral to decentralized finance systems. They will offer personalized investment strategies, thus broadening everyday investors’ access to advanced trading opportunities.